Automated streamliner portfolios for constraint satisfaction problems.
Artif. Intell.(2023)
摘要
Constraint Programming (CP) is a powerful technique for solving large-scale combinatorial problems. Solving a problem proceeds in two distinct phases: modelling and solving. Effec-tive modelling has a huge impact on the performance of the solving process. Even with the advance of modern automated modelling tools, search spaces involved can be so vast that problems can still be difficult to solve. To further constrain the model, a more aggressive step that can be taken is the addition of streamliner constraints, which are not guaranteed to be sound but are designed to focus effort on a highly restricted but promising portion of the search space. Previously, producing effective streamlined models was a manual, difficult and time-consuming task. This paper presents a completely automated process to the gen-eration, search and selection of streamliner portfolios to produce a substantial reduction in search effort across a diverse range of problems. The results demonstrate a marked im-provement in performance for both Chuffed, a CP solver with clause learning, and lingeling, a modern SAT solver.(c) 2023 The Authors. Published by Elsevier B.V. This is an open access article under the CC BY license (http://creativecommons .org /licenses /by /4 .0/).
更多查看译文
关键词
Constraint programming, Constraint modelling, Constraint satisfaction problem, Algorithm selection
AI 理解论文
溯源树
样例
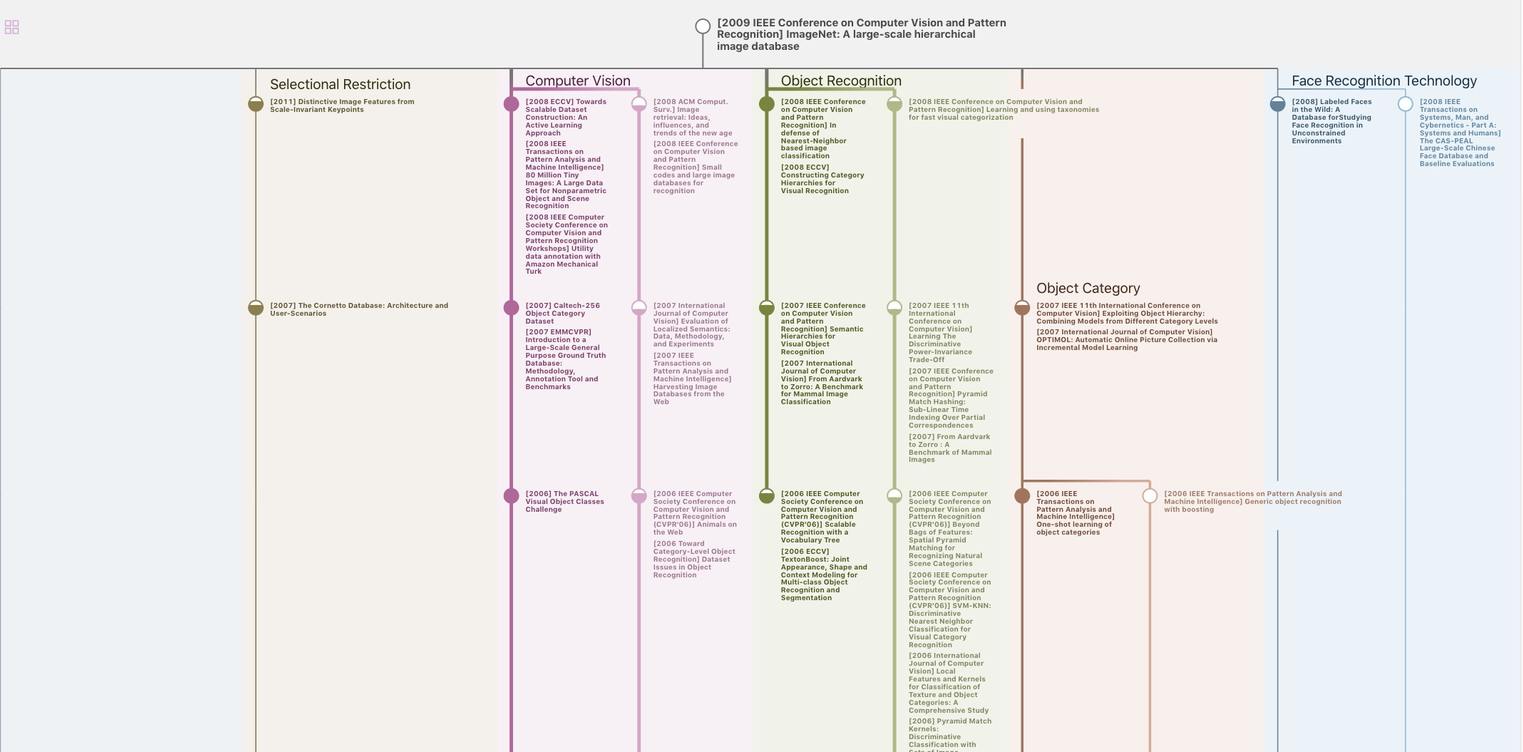
生成溯源树,研究论文发展脉络
Chat Paper
正在生成论文摘要