Prediction of Heart Disease Using a Hybrid XGBoost-GA Algorithm with Principal Component Analysis: A Real Case Study
Int. J. Artif. Intell. Tools(2023)
摘要
Cardiovascular diseases are one of the most common causes of death in the world. At this point, early diagnosis of heart diseases is critically important. The aim of this study is to predict the heart disease using feature selection, classification and optimization algorithms. Firstly, principal component analysis (PCA) is used to create the feature selection model and to determine the effective attributes. Then, Extreme Gradient Boosting (XGBoost) classification model is proposed to predict the heart disease. Finally, genetic algorithm (GA) is applied to optimize the parameters of XGBoost to improve the classification accuracy. The developed hybrid PCA-XGBoost-GA approach is compared with XGBoost, PCA-XGBoost, XGBoost-GA, artificial neural network (ANN) and support vector machine (SVM). The effectiveness of these approaches is illustrated with a case study with the actual data taken from a university hospital in Turkey. The numerical results show that the proposed PCA-XGBoost-GA model outperforms the other classification models in terms of accuracy rate, recall, precision and F-measure. Moreover, feature selection and parameter optimization improve the classification performance of the XGBoost model.
更多查看译文
关键词
principal component analysis,heart disease,prediction
AI 理解论文
溯源树
样例
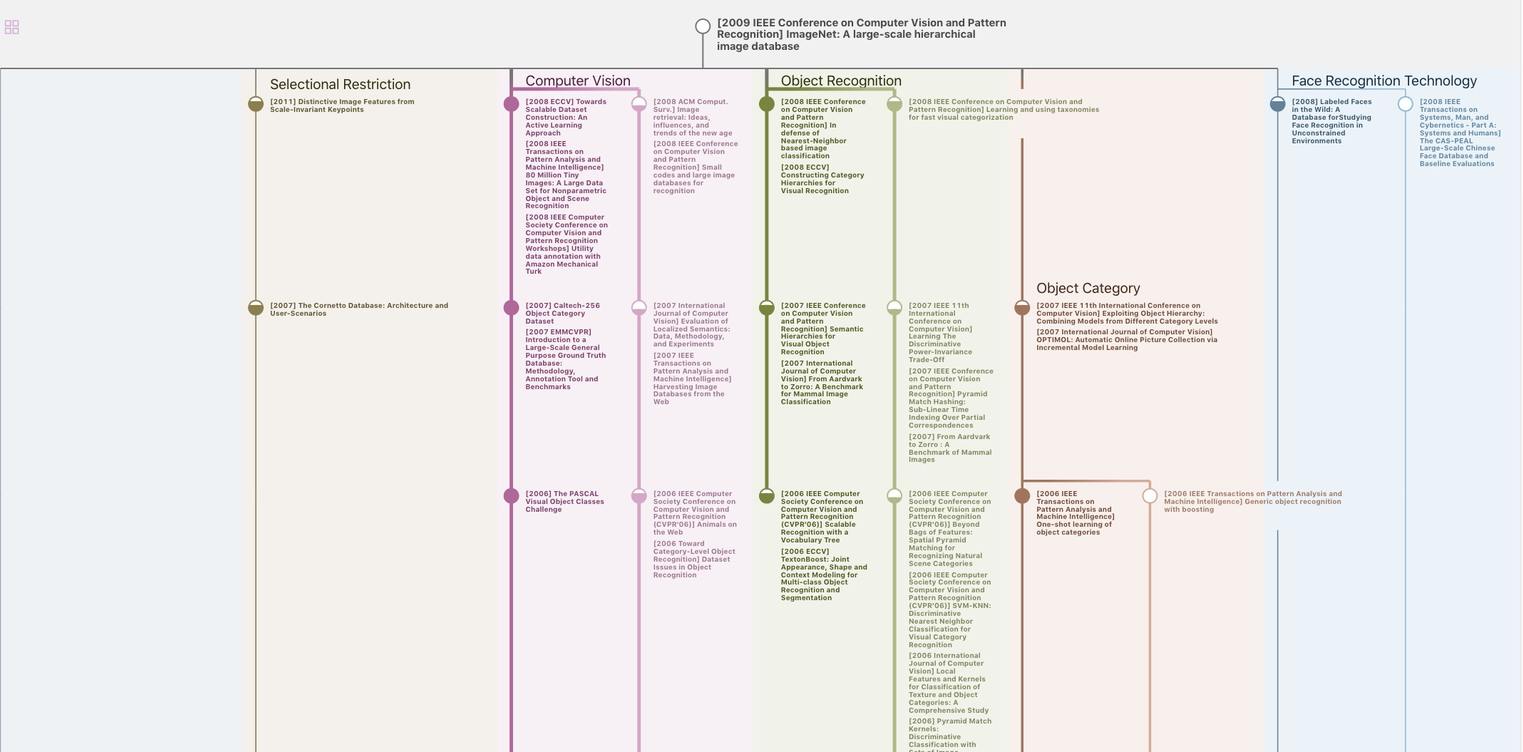
生成溯源树,研究论文发展脉络
Chat Paper
正在生成论文摘要