Decentralized Multi-Robot Information Gathering From Unknown Spatial Fields.
IEEE Robotics Autom. Lett.(2023)
摘要
We present an incremental scalable motion planning algorithm for finding maximally informative trajectories for decentralized mobile robots. These robots are deployed to observe an unknown spatial field, where the informativeness of observations is specified as a density function. Existing works that are typically restricted to discrete domains and synchronous planning often scale poorly depending on the size of the problem. Our goal is to design a distributed control law in continuous domains and an asynchronous communication strategy to guide a team of cooperative robots to visit the most informative locations within a limited mission duration. Our proposed Asynchronous Information Gathering with Bayesian Optimization (AsyncIGBO) algorithm extends ideas from asynchronous Bayesian Optimization (BO) to efficiently sample from a density function. It then combines them with decentralized reactive motion planning techniques to achieve efficient multi-robot information gathering activities. We provide a theoretical justification for our algorithm by deriving an asymptotic no-regret analysis with respect to a known spatial field. Our proposed algorithm is extensively validated through simulation and real-world experiment results with multiple robots.
更多查看译文
关键词
multi-robot
AI 理解论文
溯源树
样例
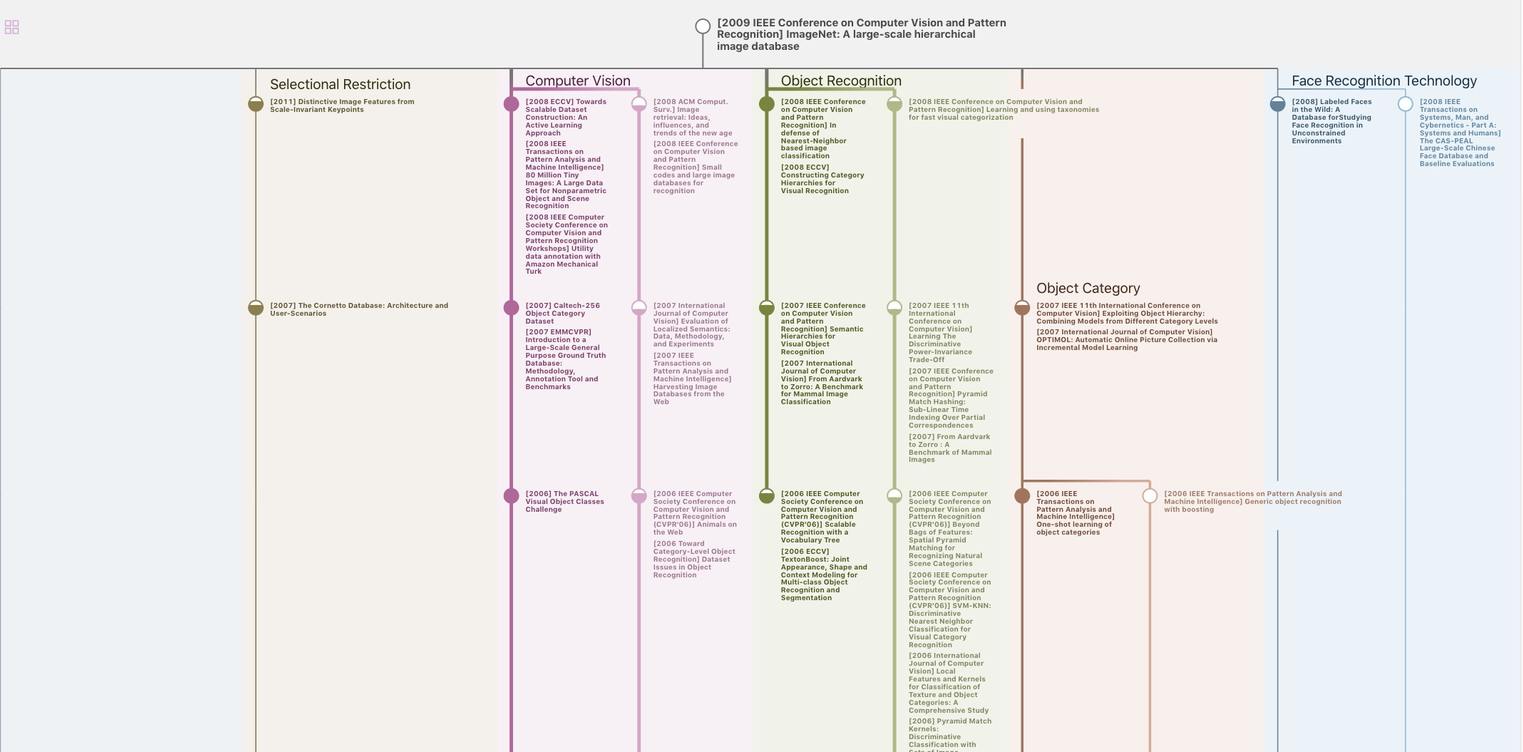
生成溯源树,研究论文发展脉络
Chat Paper
正在生成论文摘要