Object-centric Contour-aware Data Augmentation Using Superpixels of Varying Granularity.
Pattern Recognit.(2023)
摘要
Regional dropout strategies have demonstrated to be very effective in improving both the performance and the generalization capability of deep learning models. However, when such strategies are performed in a totally random manner, the background noise and label mismatch problems arise. To tackle such problems, existing approaches typically focus on regions with the highest distinctiveness. Yet, there are two main drawbacks of existing approaches: (I) Many existing region-based augmentation methods can only use rectangular regions, resulting in the loss of object contour information; (II) Deterministic se-lection of the most discriminative regions leads to poor diversification in data augmentation. In fact, a trade-off is needed between diversification and concentration, which can decrease the undesirable noise.In this paper, we propose a novel object-centric contour-aware CutMix data augmentation strategy with arbitrary-shape and size superpixel supports, which is hereafter referred to as OcCaMix for short. It not only captures the most discriminative regions, but also effectively preserves the contour details of the objects. Moreover, it enables the search of natural object parts of different sizes. Extensive experiments on a large number of benchmark datasets show that OcCaMix significantly outperforms state-of-the-art CutMix based data augmentation methods in classification tasks. The source codes and trained models are available at https://github.com/DanielaPlusPlus/OcCaMix .(c) 2023 The Author(s). Published by Elsevier Ltd. This is an open access article under the CC BY-NC-ND license ( http://creativecommons.org/licenses/by-nc-nd/4.0/ )
更多查看译文
关键词
Data augmentation,Cutmix,Object-centric Contour-aware,Discriminative regions,Attention,Superpixels
AI 理解论文
溯源树
样例
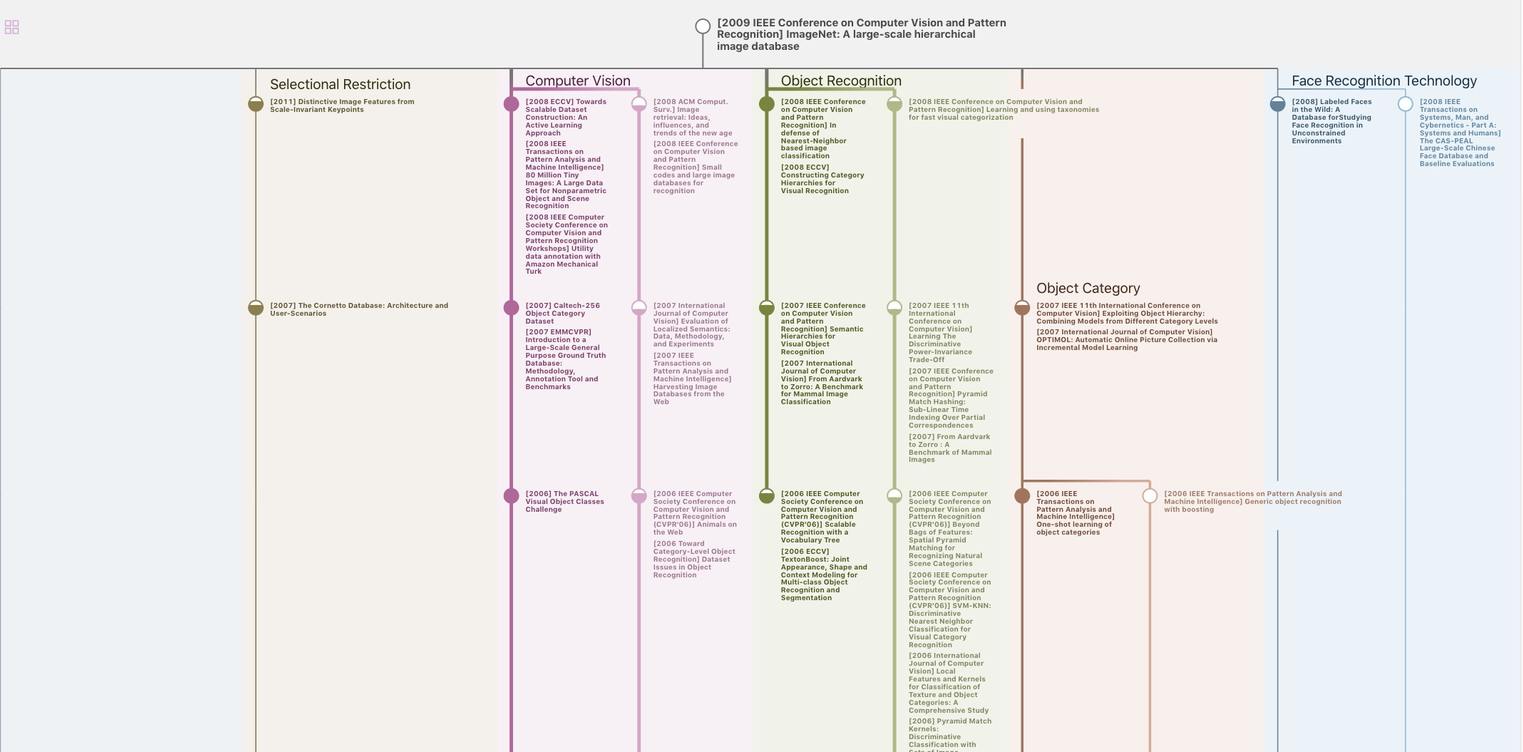
生成溯源树,研究论文发展脉络
Chat Paper
正在生成论文摘要