InvMap and Witness Simplicial Variational Auto-Encoders
Mach. Learn. Knowl. Extr.(2023)
摘要
Variational auto-encoders (VAEs) are deep generative models used for unsupervised learning, however their standard version is not topology-aware in practice since the data topology may not be taken into consideration. In this paper, we propose two different approaches with the aim to preserve the topological structure between the input space and the latent representation of a VAE. Firstly, we introduce InvMap-VAE as a way to turn any dimensionality reduction technique, given an embedding it produces, into a generative model within a VAE framework providing an inverse mapping into original space. Secondly, we propose the Witness Simplicial VAE as an extension of the simplicial auto-encoder to the variational setup using a witness complex for computing the simplicial regularization, and we motivate this method theoretically using tools from algebraic topology. The Witness Simplicial VAE is independent of any dimensionality reduction technique and together with its extension, Isolandmarks Witness Simplicial VAE, preserves the persistent Betti numbers of a dataset better than a standard VAE.
更多查看译文
关键词
variational auto-encoder,topological machine learning,non-linear dimensionality reduction,topological data analysis,data visualization,representation learning,Betti number,persistence homology,simplicial complex,simplicial regularization
AI 理解论文
溯源树
样例
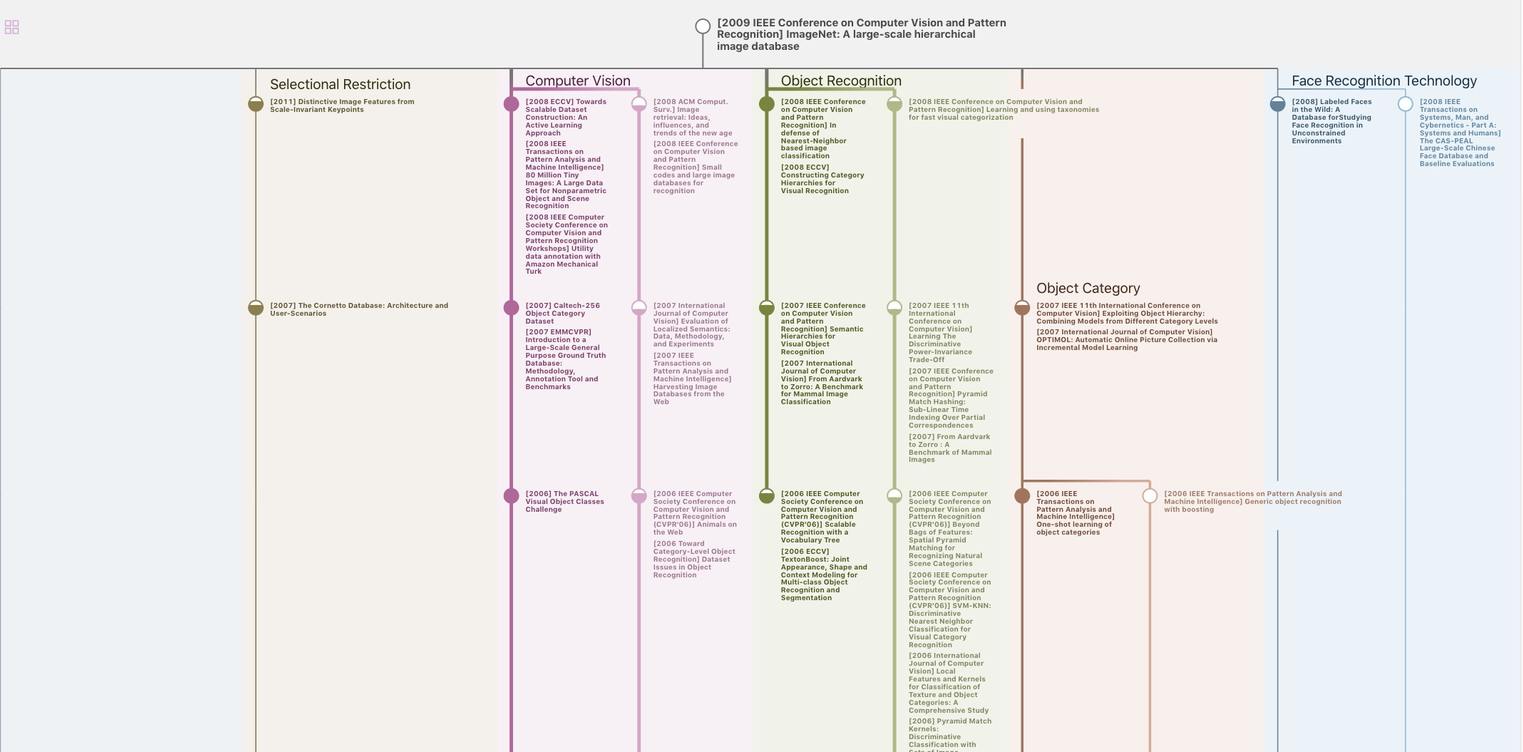
生成溯源树,研究论文发展脉络
Chat Paper
正在生成论文摘要