Data-Driven Early Diagnosis of Chronic Kidney Disease: Development and Evaluation of an Explainable AI Model.
IEEE Access(2023)
摘要
Chronic Kidney Disease (CKD) is currently experiencing a growing worldwide incidence and can lead to premature mortality if diagnosed late, resulting in rising costs to healthcare systems. Artificial Intelligence (AI) and Machine Learning (ML) offer the possibility of an early diagnosis of CKD that could revert further kidney damage. However, clinicians may be hesitant to adopt AI models if the reasoning behind the predictions is not understandable. Since eXplainable AI (XAI) addresses the clinicians' requirement of understanding AI models' output, this work presents the development and evaluation of an explainable CKD prediction model that provides information about how different patient's clinical features contribute to CKD early diagnosis. The model was developed using an optimization framework that balances classification accuracy and explainability. The main contribution of the paper lies in an explainable data-driven approach to offer quantitative information about the contribution of certain clinical features in the early diagnosis of CKD. As a result, the optimal explainable prediction model implements an extreme gradient boosting classifier using 3 features (hemoglobin, specific gravity, and hypertension) with an accuracy of 99.2% (standard deviation 0.8) and 97.5% with a 5-fold cross-validation and with new unseen data respectively. In addition, an explainability analysis shows that hemoglobin is the most relevant feature that influences the prediction, followed by specific gravity and hypertension. This small number of features selected results in a reduced cost of the early diagnosis of CKD implying a promising solution for developing countries.
更多查看译文
关键词
Clinical prediction model,early diagnosis,chronic kidney disease,feature selection,medical explainable AI
AI 理解论文
溯源树
样例
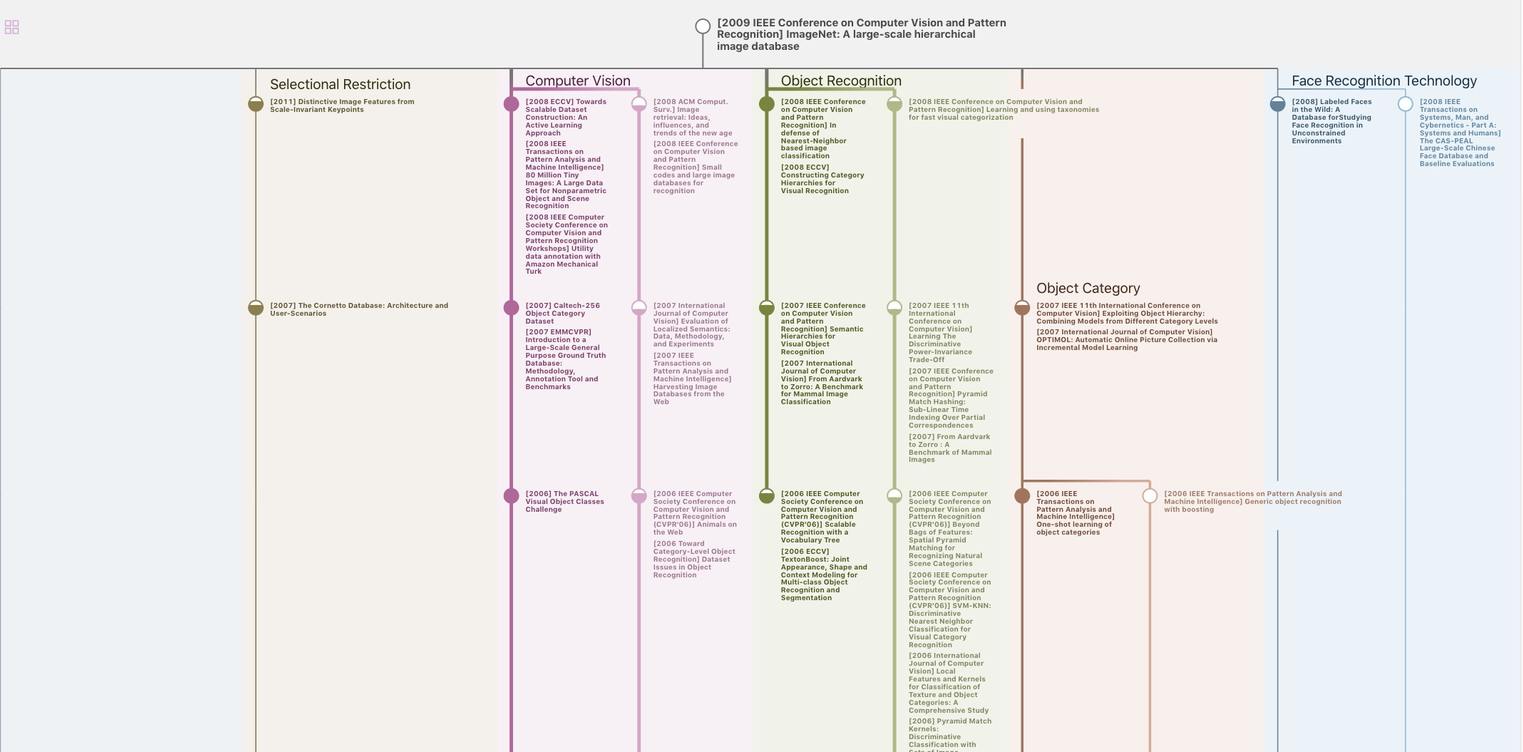
生成溯源树,研究论文发展脉络
Chat Paper
正在生成论文摘要