Early Yield Estimation in Viticulture Based on Grapevine Inflorescence Detection and Counting in Videos
IEEE Access(2023)
摘要
In viticulture, yield estimation is a key activity, which is important throughout the wine industry value chain. The earlier that an accurate yield estimation can be made the greater its value, increasing management options for grape growers and commercial options for winemakers. For the yield estimate based on in- field measurements at scale, the number of inflorescences emerging after bud-burst offers the earliest practical signal, allowing a yield potential to be determined months before harvest. This paper presents an approach to automatically count the inflorescence number at the phenological stage E-L 12 using RGB video data and demonstrates its use for estimating yield. A dataset consisting of RGB videos was collected shortly after bud-burst from multiple vineyards, in conjunction with hand counts to produce a manual ground-truth for the inflorescence counting task. The video frames were annotated using bounding-boxes around the inflorescences to produce a digital ground-truth. A deep learning architecture was developed to learn features from the video frames during training and detect the inflorescences at the later inference stage. The detection results were fed to a tracking pipeline built using computer vision and deep learning techniques to generate numbers of inflorescences present in test videos. The visual and quantitative results are presented and evaluated for the inflorescence detection and counting tasks. The developed inflorescence detector achieves an average precision of 80.00%, a recall of 83.92%, and an F1-score of 80.48%, through a five-fold cross-validation on the annotated dataset. For the test videos, the developed automatic inflorescence counting model reports an absolute error of 11.03 inflorescences per panel, a normalized mean absolute error of 10.80%, and an R(2 )of 0.86, when the predicted per-panel counts were compared to the corresponding manual ground-truth. Based on the counting results, we estimate an early yield that is within 4% to 11% error when compared to the actual yield after harvest. Based on these results and a separate analysis of the relationship between hand counts of inflorescences and harvest yields in three vineyards over three growing seasons, we conclude that computer vision and machine learning based methods have the potential to provide early yield estimation in viticulture with a commercially viable accuracy.
更多查看译文
关键词
Videos,Yield estimation,Detectors,Pipelines,Australia,Task analysis,Feature extraction,Grapevine,inflorescence detection,inflorescence tracking,early yield estimation,viticulture,computer vision,deep learning
AI 理解论文
溯源树
样例
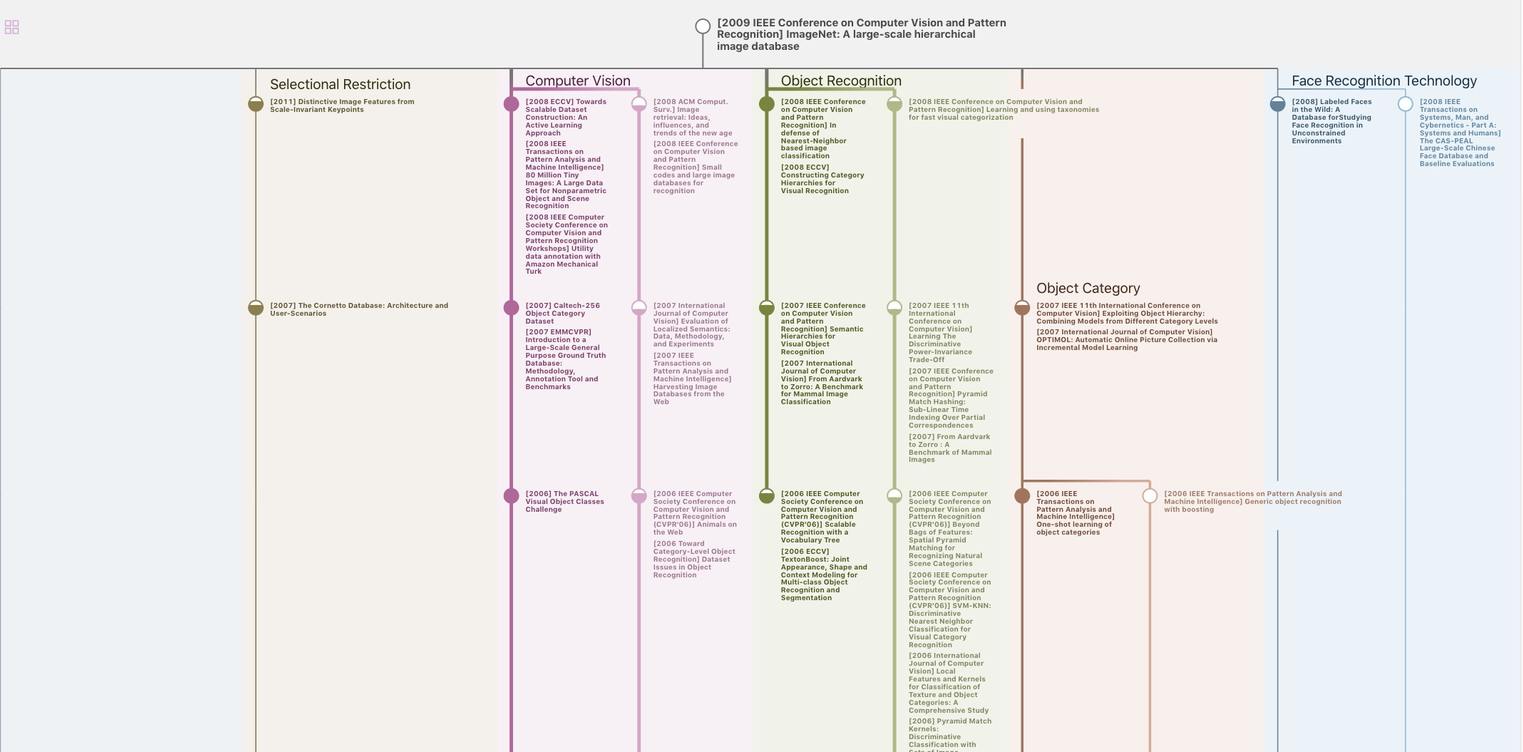
生成溯源树,研究论文发展脉络
Chat Paper
正在生成论文摘要