Intelligent Urban Sensing for Gas Leakage Risk Assessment.
IEEE Access(2023)
摘要
In order to mitigate gas leakage damage, it is important to assess systematic risk of gas leakage in utility networks. In practice, leakage detection systems heavily rely on tedious and expensive human efforts, such as manually checking and assessing the risk, and thus only a very limited portion of the gas pipeline network can usually be assessed during a period of time. Therefore, it is critical to develop tools for automatically and systematically evaluating the whole gas pipeline network and revealing high-risk sites for checking in a timely fashion. To this end, in this paper, we develop an intelligent gas leakage risk assessment system based on the analysis of large-scale multi-source data, such as gas pipeline data, Point of Interests (POIs) data, and human mobility data. However, it is a non-trivial endeavor to design such a risk assessment system due to the unclear leakage mechanism, complex environmental conditions, and large size of the pipeline network. To address these challenges, both internal features of gas pipelines and external environmental features should be exploited. Specifically, we design a novel urban sensing technique to extract environmental features by analyzing human mobility data. Then, a joint learning neural network is developed to assess the leakage risk by integrating both internal and external features. Moreover, an intelligent risk assessment system is implemented and deployed for experiments in real-world scenarios. The results show the effectiveness of our system for citywide gas leakage risk assessment. Indeed, the proposed system could help to locate the potential leakage sites in a timely way.
更多查看译文
关键词
gas leakage risk assessment,urban,risk assessment
AI 理解论文
溯源树
样例
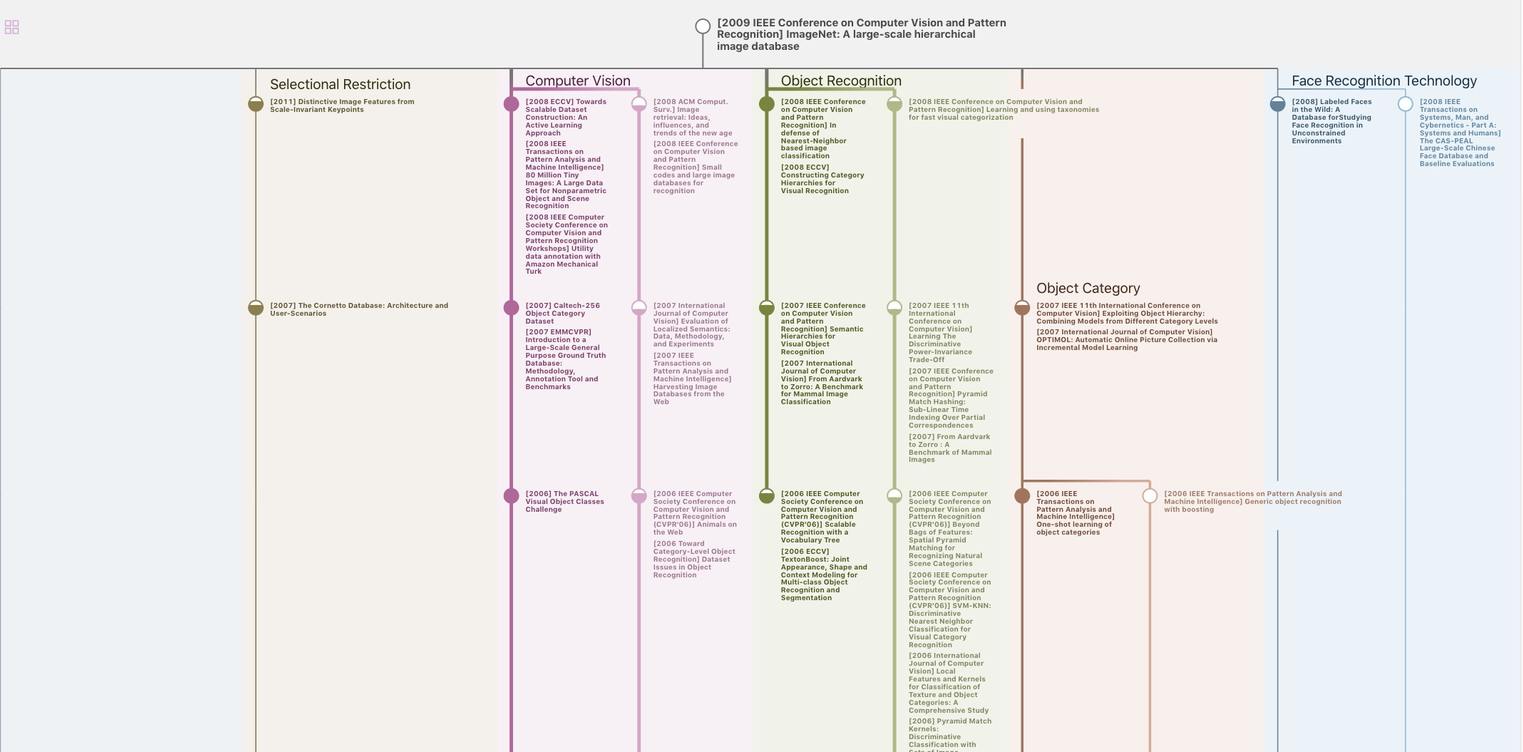
生成溯源树,研究论文发展脉络
Chat Paper
正在生成论文摘要