IoT-assisted feature learning for surface settlement prediction caused by shield tunnelling.
Comput. Commun.(2023)
摘要
The surface settlement caused by shield tunnelling may lead to collapse disaster, threatening the safety of ground and underground structures. Therefore, the prediction of surface settlement has attracted much attention, but the problem has not been well solved. Based on the Internet of Things and data driven method, this paper constructs a data model to predict the surface settlement on the basis of fully considering the time-space relationship of surface settlement caused by shield tunnelling. Then, based on this model, the convolutional neural network is used to learn time series features, the attention mechanism is used to realize feature interaction between parameters, and the tensorized long-term and short-term memory network are used to complete feature fusion between rings. A tensorized long-term and short-term memory network based on convolution feature adaptive interaction (TLCFAI) is proposed. Taking the tunnelling process of 10 303 rings in 13 shield tunnel sections in Changzhou, China as an example, TLCFAI is obviously better than the other 9 typical algorithms, and the prediction effect is good. The research shows that this paper provides a relatively complete method and technical means for the prediction of surface settlement caused by shield tunnelling from the construction of data model to feature learning algorithm.
更多查看译文
关键词
Shield tunnelling,Surface settlement,Internet of Things,Data model,Feature learning
AI 理解论文
溯源树
样例
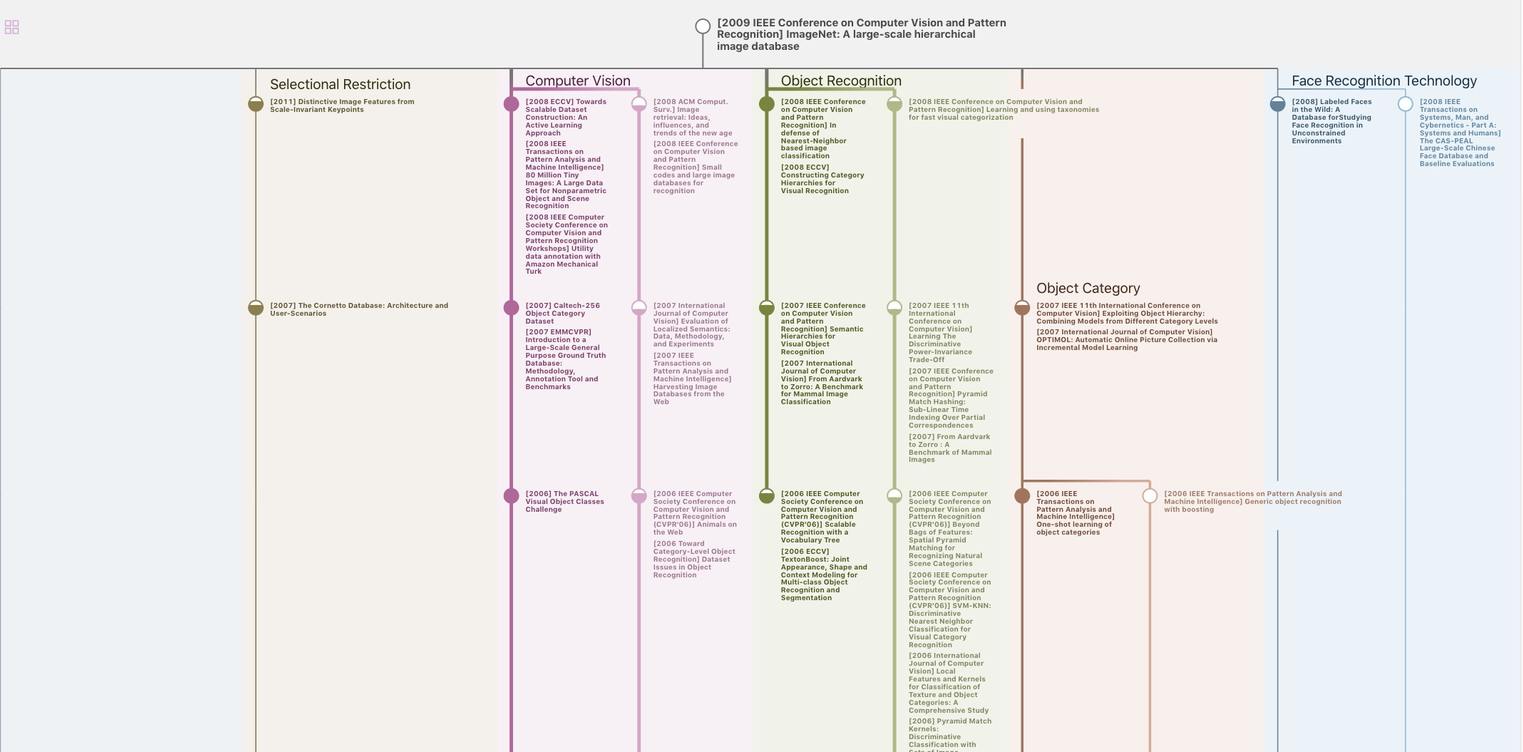
生成溯源树,研究论文发展脉络
Chat Paper
正在生成论文摘要