Brain-Machine Interfaces: Closed-Loop Control in an Adaptive System.
Annu. Rev. Control. Robotics Auton. Syst.(2021)
摘要
wBrain-machine interfaces (BMIs) promise to restore movement and communication in people with paralysis and ultimately allow the human brain to interact seamlessly with external devices, paving the way for a new wave of medical and consumer technology. However, neural activity can adapt and change over time, presenting a substantial challenge for reliable BMI implementation. Large-scale recordings in animal studies now allow us to study how behavioral information is distributed in multiple brain areas, and state-of-the-art interfaces now incorporate models of the brain as a feedback controller. Ongoing research aims to understand the impact of neural plasticity on BMIs and find ways to leverage learning while accommodating unexpected changes in the neural code. We review the current state of experimental and clinical BMI research, focusing on what we know about the neural code, methods for optimizing decoders for closed-loop control, and emerging strategies for addressing neural plasticity.
更多查看译文
关键词
brain-machine interfaces, neural plasticity, optimal feedback control, neural coding, adaptive decoding
AI 理解论文
溯源树
样例
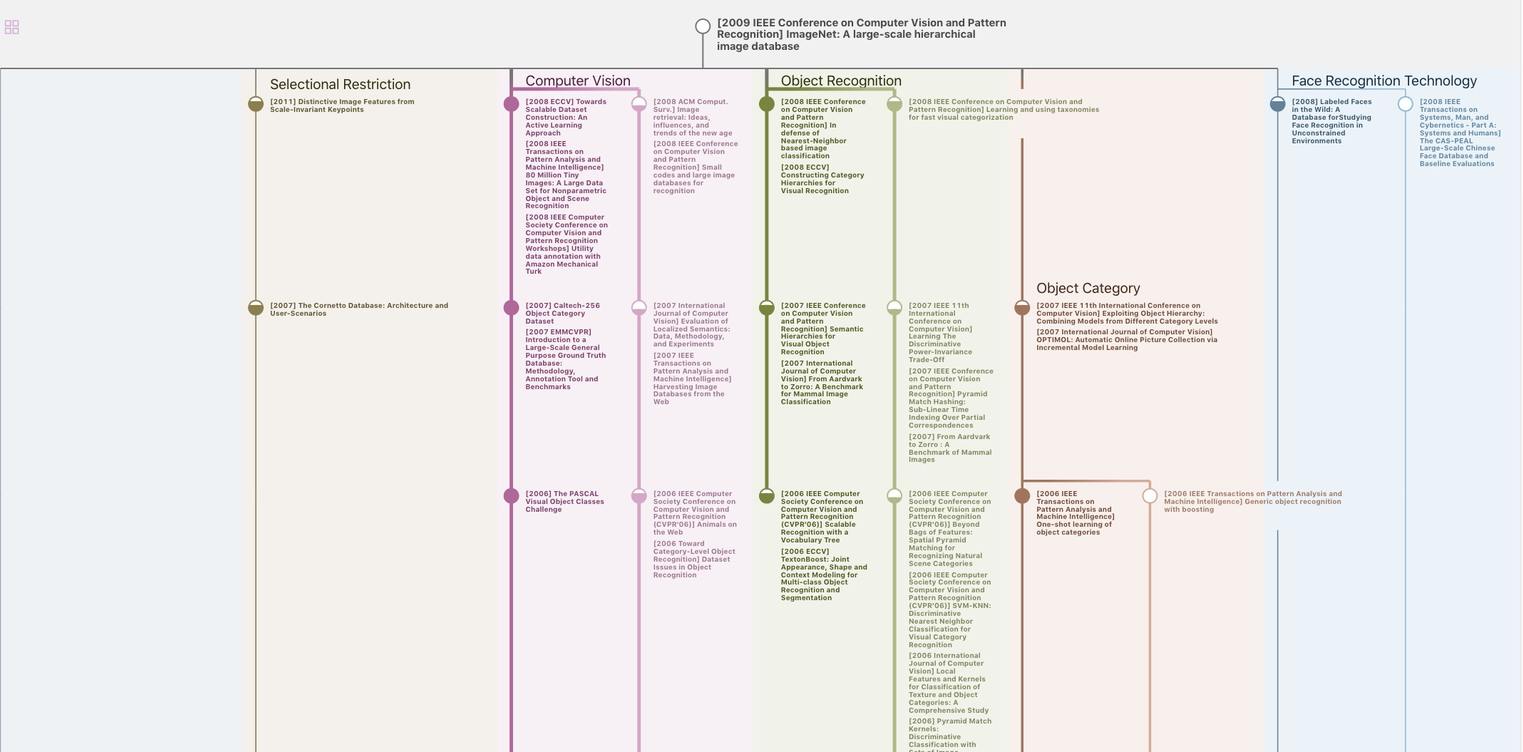
生成溯源树,研究论文发展脉络
Chat Paper
正在生成论文摘要