Subgraph Sampling for Inductive Sparse Cloud Services QoS Prediction.
ICPADS(2022)
摘要
Quality-of-Service (QoS) based collaborative prediction models are emerging to select appropriate edge cloud services for users. Nevertheless, there are still challenges in the real-world QoS prediction task. First, existing QoS prediction models are mostly transductive, failing to generalize to unseen users and services. Secondly, an accurate prediction model remains unexplored under the extreme sparse data scenario, where only a few interactions are available for collaborative filtering. To address these problems, we propose Inductive Subgraph Pattern Aware Graph Neural Network (ISPA-GNN), which leverages a novel graph-based collaborative filtering method with a subgraph sampling strategy. We further optimize the embeddings components, replacing the user/service embeddings with compositional context information to enable better generalization to unseen nodes while reducing memory usage. Extensive experiments on a large-scale real-world service QoS dataset demonstrate some decent properties of our model, including high prediction accuracy, memory efficiency, and slight performance degradation even if 25% of users/services are never seen.
更多查看译文
关键词
Collaborative Filtering,Cloud Service,Graph Neural Network,QoS Prediction
AI 理解论文
溯源树
样例
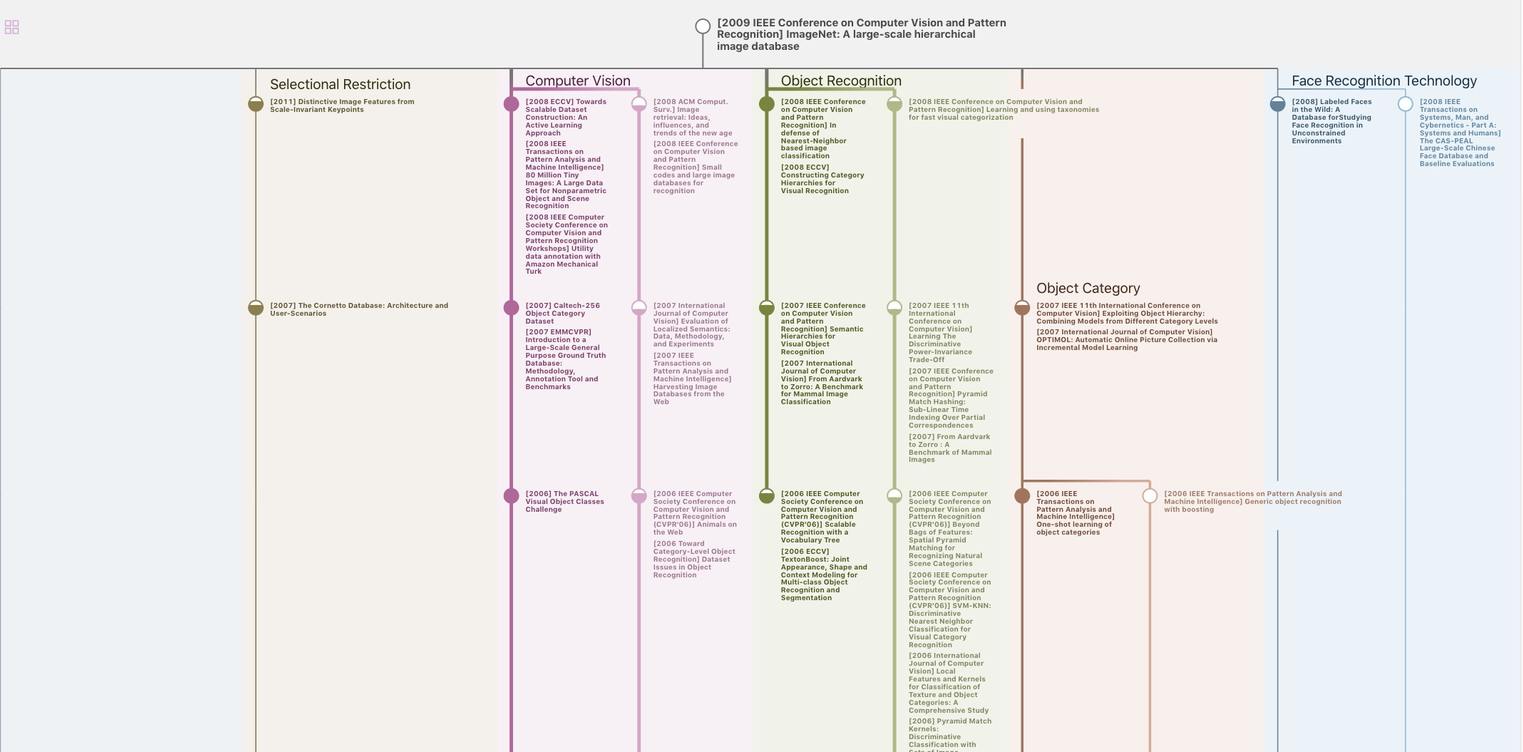
生成溯源树,研究论文发展脉络
Chat Paper
正在生成论文摘要