Channel Spatial Collaborative Attention Network for Fine-Grained Classification of Cervical Cells.
ICONIP (6)(2022)
摘要
Accurately classifying cervical cells based on the commonly used TBS (The Bethesda System) standard is critical for building the automatic cytology diagnosing system. However, the existing two publicly available datasets (Herlev and SIPaKMeD) do not provide the TBS labels for the cervical cells, leading to the lack of practicability of the models trained based on them. In addition, most deep learning-based methods for cervical cell classification do not explore the attention spots of the model, resulting in the limit of further focusing on discriminative parts of tiny cells. In this paper, we first build a cervical cell image dataset consisting of 10,294 cell image patches with TBS labels from 238 specimens, named LTCDD (Landing TBS Cervical Cell Dataset). Then we propose a novel visual attention module, channel spatial collaborative attention (CSCA), based on the integration of the channel and spatial attention. Two kinds of comparing experiments on LTCDD and two public datasets show that the CSCA embedded network can achieve the best classification performance. Moreover, the CSCA module performs better than other attention modules when integrated into ResNet. The visualization results illustrate that CSCA embedded networks can pay more attention to the nucleus part of cell images so as to focus on learning discriminative features that are beneficial to the classification of the cells.
更多查看译文
关键词
cervical cells,attention,classification,channel,fine-grained
AI 理解论文
溯源树
样例
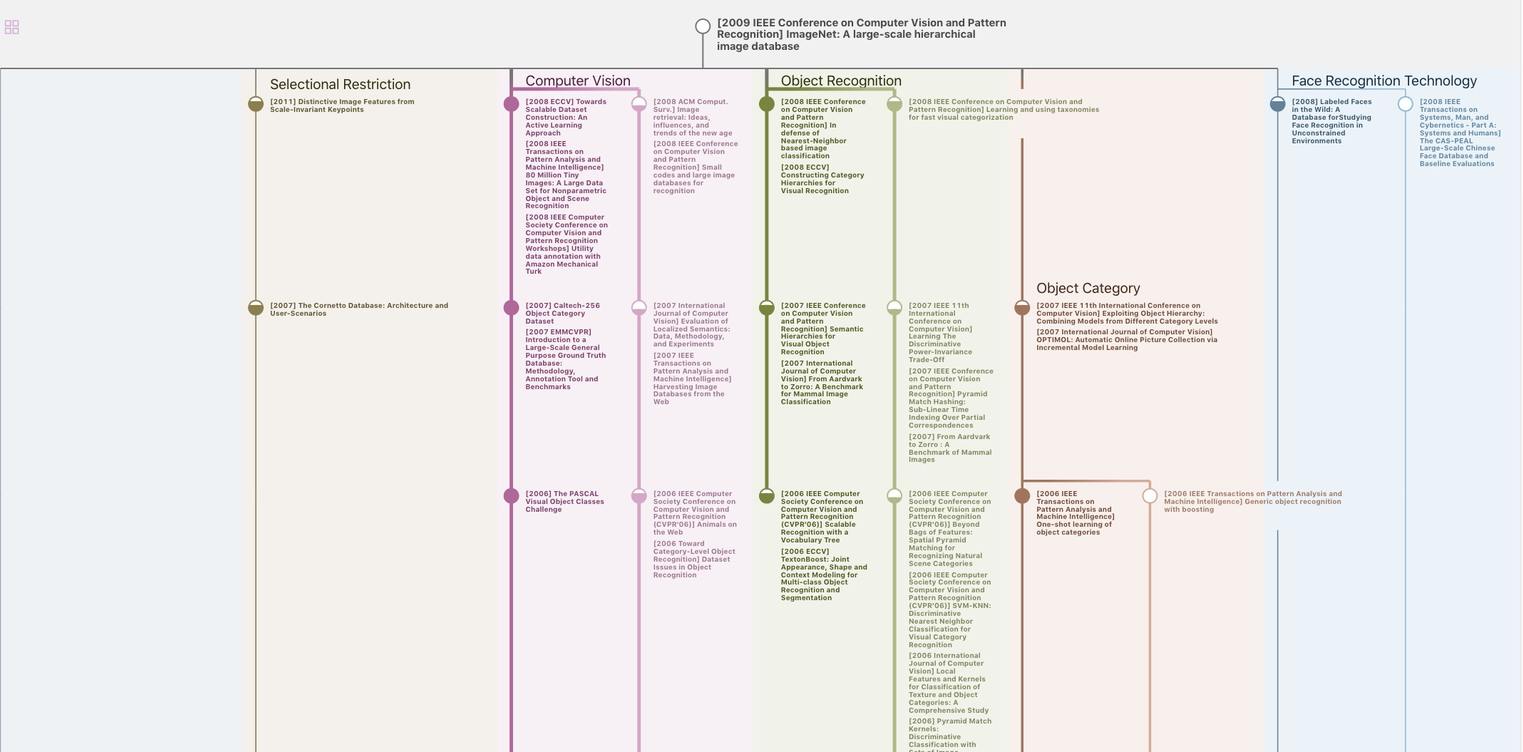
生成溯源树,研究论文发展脉络
Chat Paper
正在生成论文摘要