Searching for Textual Adversarial Examples with Learned Strategy.
ICONIP (4)(2022)
摘要
Adversarial attacks can help to reveal the vulnerability of neural networks. In the text classification domain, synonym replacement is an effective way to generate adversarial examples. However, the number of replacement combinations grows exponentially with the text length, making the search difficult. In this work, we propose an attack method which combines a synonym selection network and search strategies of beam search and Monte Carlo tree search (MCTS). The synonym selection network learns the patterns of synonyms which have high attack effect. We combine the network with beam search to gain a broader view by multiple search paths, and with MCTS to gain a deeper view by the exploration feedback, so as to effectively avoid local optimum. We evaluate our method with four datasets in a challenging black box setting which requires no access to the victim model’s parameters. Experimental results show that our method can generate high-quality adversarial examples with higher attack success rate and fewer number of victim model queries, and further experiments show that our method has higher transferability on the victim models. The code and data can be obtained via https://github.com/CMACH508/SearchTextualAdversarialExamples .
更多查看译文
关键词
textual adversarial examples,learned strategy
AI 理解论文
溯源树
样例
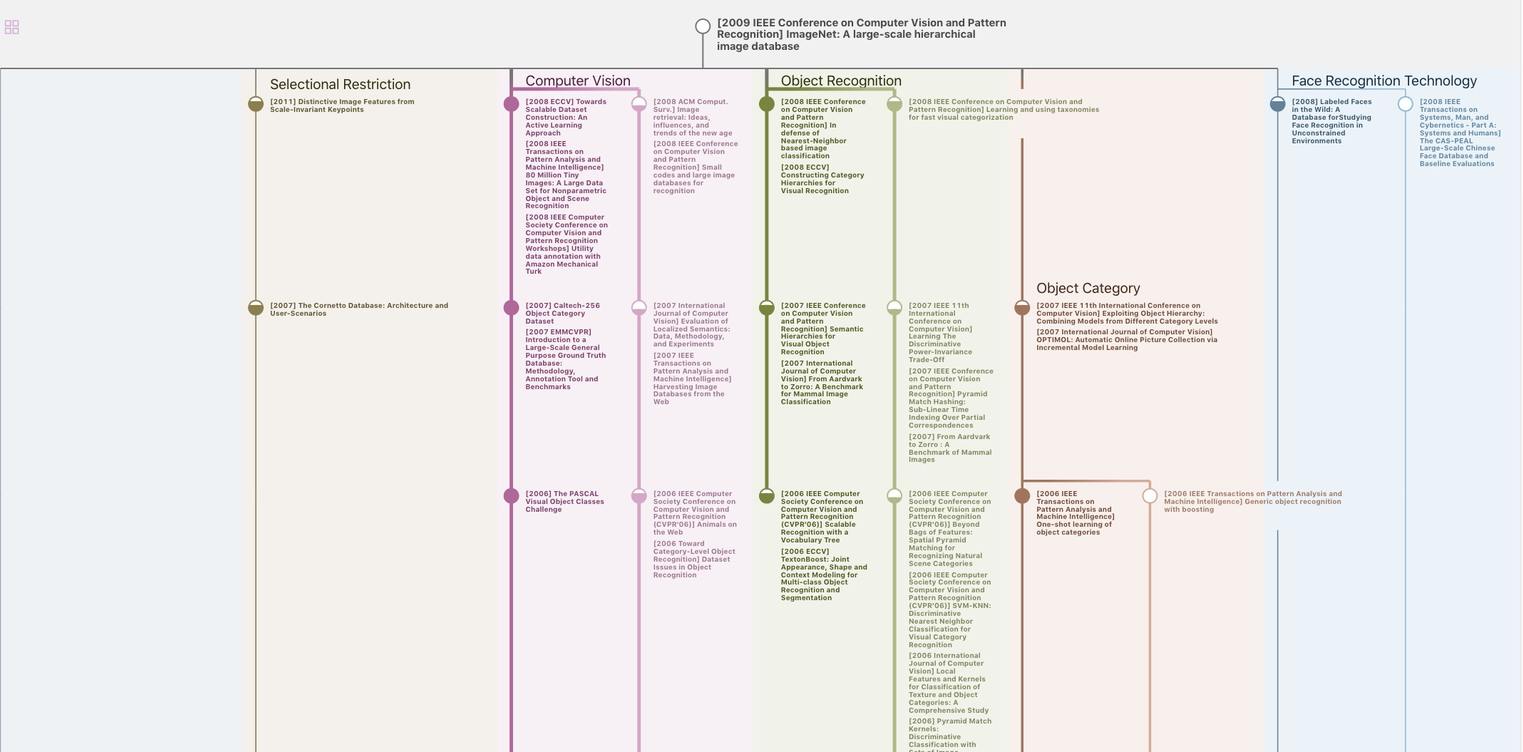
生成溯源树,研究论文发展脉络
Chat Paper
正在生成论文摘要