Multi-scale Gaussian Difference Preprocessing and Dual Stream CNN-Transformer Hybrid Network for Skin Lesion Segmentation.
MMM (2)(2023)
摘要
Skin lesions segmentation from dermoscopic images has been a long-standing challenging problem, which is important for improving the analysis of skin cancer. Due to the large variation of melanin in the lesion area, the large number of hairs covering the lesion area, and the unclear boundary of the lesion, most previous works were hard to accurately segment the lesion area. In this paper, we propose a Multi-Scale Gaussian Difference Preprocessing and Dual Stream CNN-Transformer Hybrid Network for Skin Lesion Segmentation, which can accurately segment a high-fidelity lesion area from a dermoscopic image. Specifically, we design three specific sets of Gaussian difference convolution kernels to significantly enhance the lesion area and its edge information, conservatively enhance the lesion area and its edge information, and remove noise features such as hair. Through the information enhancement of multi-scale Gaussian convolution, the model can easily extract and represent the enhanced lesion information and lesion edge information while reducing the noise information. Secondly, we adopt dual steam network to extract features from the Gaussian difference image and the original image separately and fuse them in the feature space to accurately align the feature information. Thirdly, we apply the convolution neural network (CNN) and vision transformer (ViT) hybrid architectures to better exploit the local and global information. Finally, we use the coordinate attention mechanism and the self-attention mechanism to enhance the sensitivity to the necessary features. Extensive experimental results on the ISIC 2016, PH2, and ISIC 2018 dataset demonstrate that our proposed approach achieves compelling performance in skin lesions segmentation.
更多查看译文
关键词
skin lesion segmentation,multi-scale,cnn-transformer
AI 理解论文
溯源树
样例
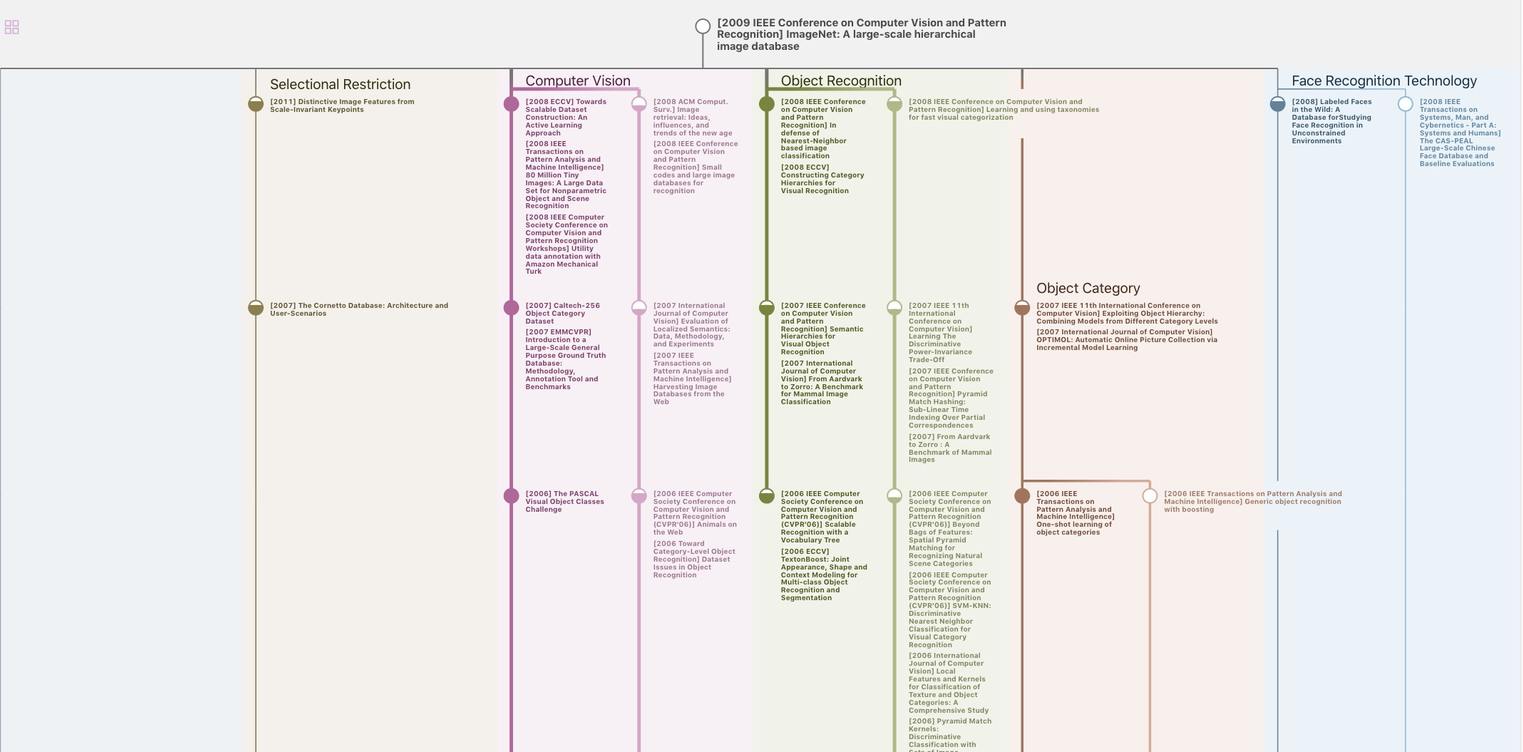
生成溯源树,研究论文发展脉络
Chat Paper
正在生成论文摘要