Offline Reinforcement Learning via Policy Regularization and Ensemble Q-Functions.
ICTAI(2022)
摘要
Offline reinforcement learning aims to learn effective policies from a fixed set of data collected in advance and without further interaction with the environment during learning. This setting will promote the applications of reinforcement learning in the real world, in which interaction is costly or dangerous. However, existing off-policy algorithms can fail in offline settings due to the distributional shift between the learned policy and the policy that collected the dataset. To solve the problem, we develop a lightweight and effective algorithm, policy regularization with behavior model (PRBM). Firstly, PRBM trains a behavior model as the regularization term during policy optimization to avoid choosing out-of-distribution (OOD) actions. Secondly, to avoid the overestimation of OOD actions, PRBM trains multiple Q-functions and uses their min-max mixture to compute Q-values. Our experiments on different datasets from various continuous control tasks demonstrate that PRBM outperforms most baselines (especially on medium quality datasets) and requires only half of their training time.
更多查看译文
关键词
Deep reinforcement learning,Offline RL,Distributional shift,Policy regularization
AI 理解论文
溯源树
样例
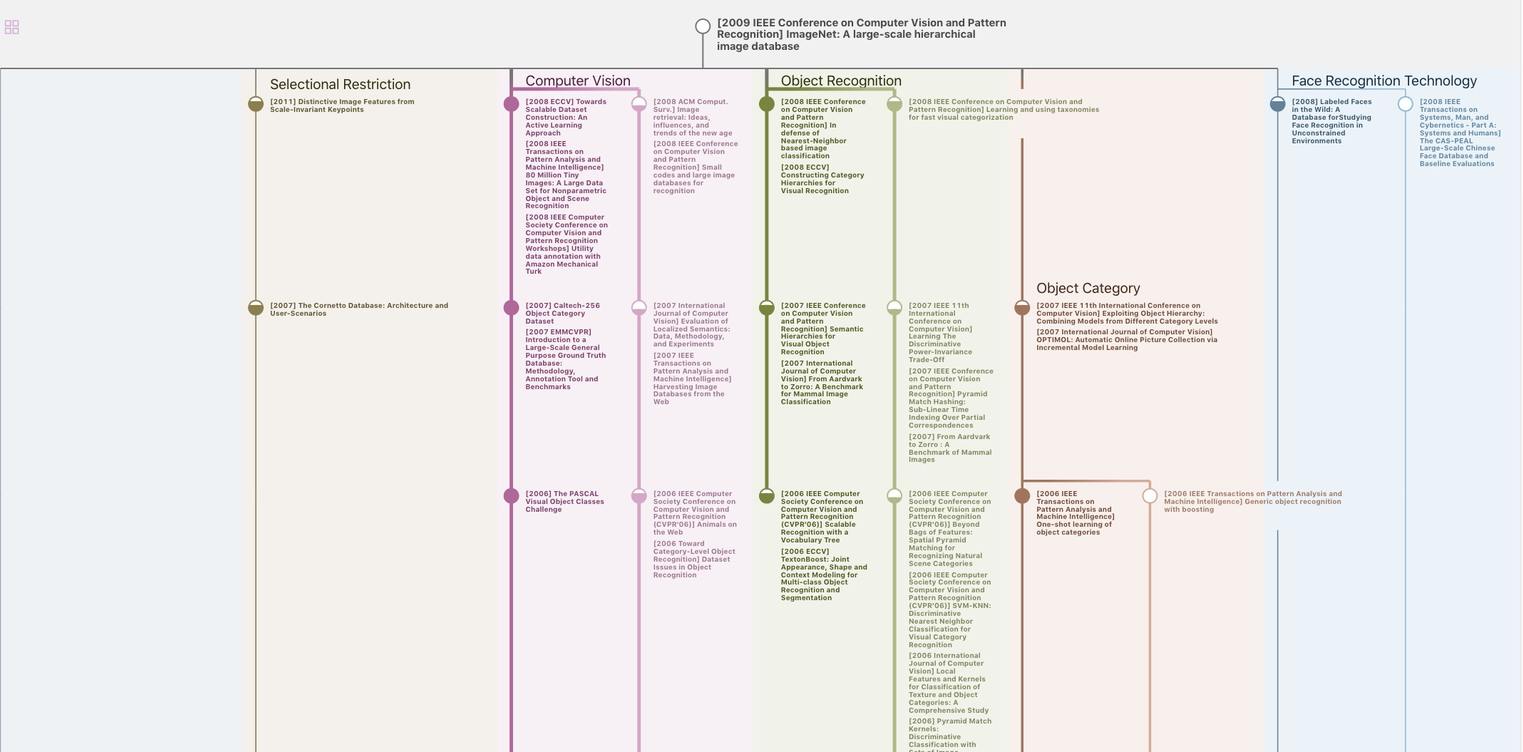
生成溯源树,研究论文发展脉络
Chat Paper
正在生成论文摘要