Low-power Robustness Learning Framework for Adversarial Attack on Edges
MSN(2022)
摘要
Recent works on adversarial attacks uncover the intrinsic vulnerability of neural networks, which reveal a critical issue that the neural networks are easily misled by adversarial attacks. As the development of edge computing, more and more real-time tasks are deployed on edge devices. The safety of these neural network-based applications is threatened by adversarial attack. Therefore, the defense technique against adversarial attack has very important application value for edges. Especially, the defense technique should consider the deployment condition on edges, such as low power and low time consumption. Unfortunately, until now, very limited research considers the security problem under adversarial attack on edges. In this paper, we propose a low-power robust learning framework to deal with the adversarial attacks at resource-constrained edge devices. In this framework, we make a rough categorization of approaches on defending against adversarial attacks, and reveal how this edge device-based framework can be used to resist adversarial attacks. Furthermore, we propose a staged ensemble defense strategy in the framework, which achieves better defensive performance than a single defense algorithm. To verify our framework on real application, we build a Drone Search and Rescue System (DSRS) which is employed to examine the performance of the proposed framework. The results indicate that our framework achieves outstanding performance in all aspects, such as robustness, time and power consumption. Multiple evaluations of the low-power robust learning framework provide the advice that help to choose the optimal security configuration on power-constrained and performance-expected environments.
更多查看译文
关键词
Edge device,UAVs,Neural networks,Adversar-ial attack and defense,Low-power robustness learning
AI 理解论文
溯源树
样例
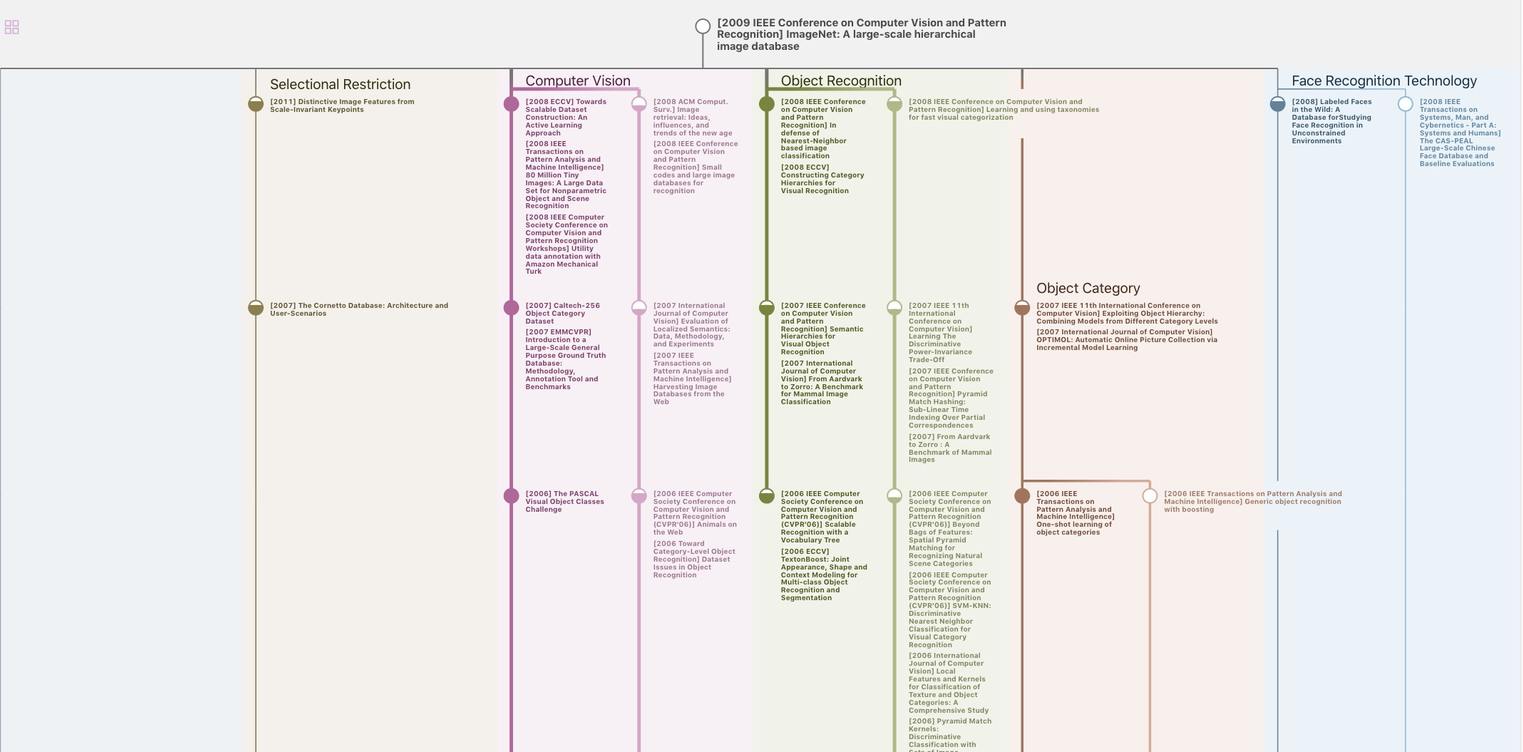
生成溯源树,研究论文发展脉络
Chat Paper
正在生成论文摘要