Exploring Information-Theoretic Criteria to Accelerate the Tuning of Neuromorphic Level-Crossing ADCs
NICE(2023)
摘要
Level-crossing analog-to-digital converters (LC-ADCs) are neuromorphic, event-driven data converters that are gaining much attention for resource-constrained applications where intelligent sensing must be provided at the extreme edge, with tight energy and area budgets. LC-ADCs translate real-world analog signals (such as ECG, EEG, etc.) into sparse spiking signals, providing significant data bandwidth reduction and inducing savings of up to two orders of magnitude in area and energy consumption at the system level compared to the use of conventional ADCs. In addition, the spiking nature of LC-ADCs make their use a natural choice for ultra-low-power, event-driven spiking neural networks (SNNs). Still, the compressed nature of LC-ADC spiking signals can jeopardize the performance of downstream tasks such as signal classification accuracy, which is highly sensitive to the LC-ADC tuning parameters. In this paper, we explore the use of popular information criteria found in model selection theory for the tuning of the LC-ADC parameters. We experimentally demonstrate that information metrics such as the Bayesian, Akaike and corrected Akaike criteria can be used to tune the LC-ADC parameters in order to maximize downstream SNN classification accuracy. We conduct our experiments using both full-resolution weights and 4-bit quantized SNNs, on two different bio-signal classification tasks. We believe that our findings can accelerate the tuning of LC-ADC parameters without resorting to computationally-expensive grid searches that require many SNN training passes.
更多查看译文
关键词
LC-ADC, Information criteria, event-based sampling, Spiking Neural Networks
AI 理解论文
溯源树
样例
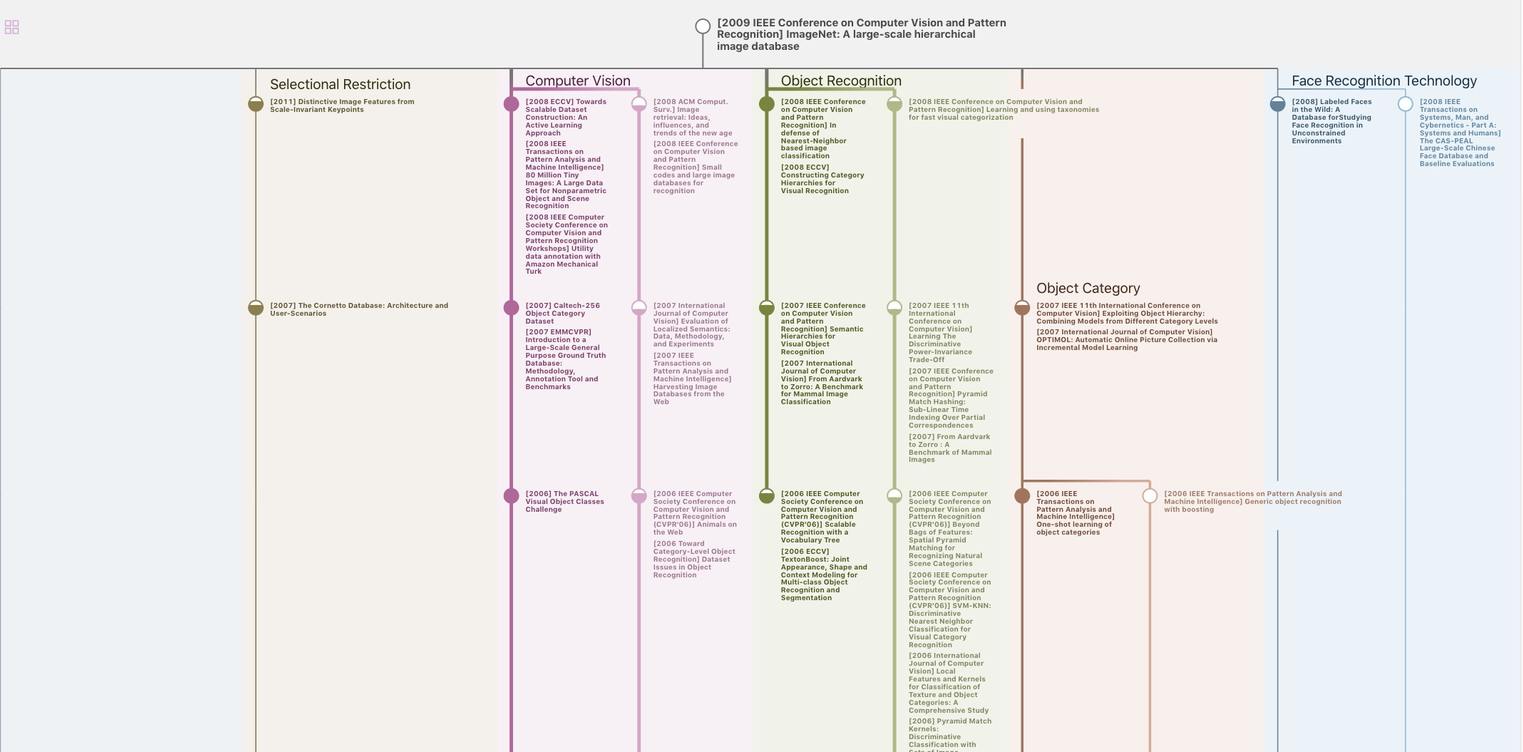
生成溯源树,研究论文发展脉络
Chat Paper
正在生成论文摘要