Meta-Path Based Social Relation Reasoning in a Deep and Robust Way.
DASFAA (3)(2023)
Abstract
Social relation reasoning in heterogeneous social networks (HSNs) should not only infer whether people are connected, but also why they know each other and what their relationship is. This task is challenging because the heterogeneous social network is non-trivial to leverage and the collected data is always noisy and incomplete, which leads that the existing rule-based and representation methods cannot efficiently and accurately reason social relations. To reason social relations in noisy and incomplete HSNs, we shift from simply inferring meta-path based social relations by rule-based methods to reasoning by social relation learning. Then, we present an inductive model named Heterogeneous Graph Variational AutoEncoders (HGVAE) towards robust social relation learning on noisy and incomplete HSN data. In HGVAE, the well-designed heterogeneous graph encoder, multi-signal decoder, and variational inference mechanism bring prominent robustness and significant performance improvement. Extensive experiments are conducted to compare our methods against a set of the most representative baseline methods. The efficiency analysis, robust analysis and ablation studies confirm the value of our proposed approach for modelling meta-path based social relations buried in various HSNs.
MoreTranslated text
Key words
relation,reasoning,meta-path
AI Read Science
Must-Reading Tree
Example
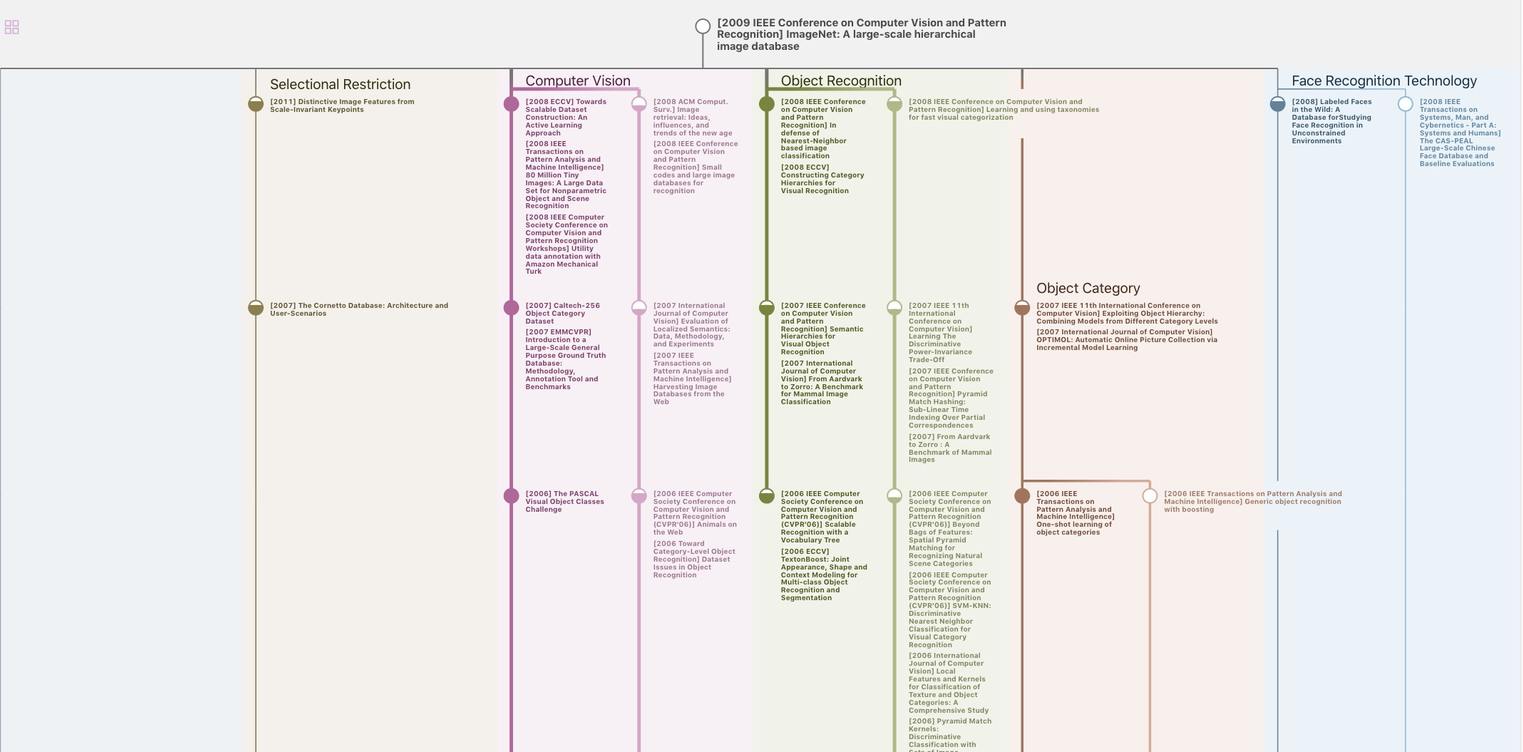
Generate MRT to find the research sequence of this paper
Chat Paper
Summary is being generated by the instructions you defined