A Joint Link-Retrieve Framework for Open Table-and-Text Question Answering.
DASFAA (3)(2023)
摘要
Open Table-and-Text Question Answering (OTTQA) task aims at answering questions that require retrieving and combining information from unstructured passages and semi-structured tables. Despite the great success achieved by the existing models, they still flaw in several drawbacks, including lacking valuable global or contextual information at the linking stage, ignoring potential error propagation and noise distraction from linking to retrieval, and neglecting the huge structural gap between table and passage. To address these problems, in this paper we propose a novel joint link-retrieve OTTQA framework, where the table block embedding and passage embedding are shared in the unified framework, and all modules are trained jointly. More specifically, we encode the table block and passage by taking full advantage of their global and contextual information and then leverage a contrastive learning approach to deeply interact with their embedding and link them together. Meanwhile, we establish a novel retrieval method to retrieve fusion blocks after linking, which does not require explicitly generating or encoding the fusion block but only focuses on the passage most relevant to the question. Furthermore, considering the structural gap between the table and text, we introduce the Table-to-Text as an auxiliary task to help the model better understand the structural difference and capture the inner semantic correlations between them. Our empirical study demonstrates that the proposed framework achieves new state-of-the-art performance.
更多查看译文
关键词
question answering,link-retrieve,table-and-text
AI 理解论文
溯源树
样例
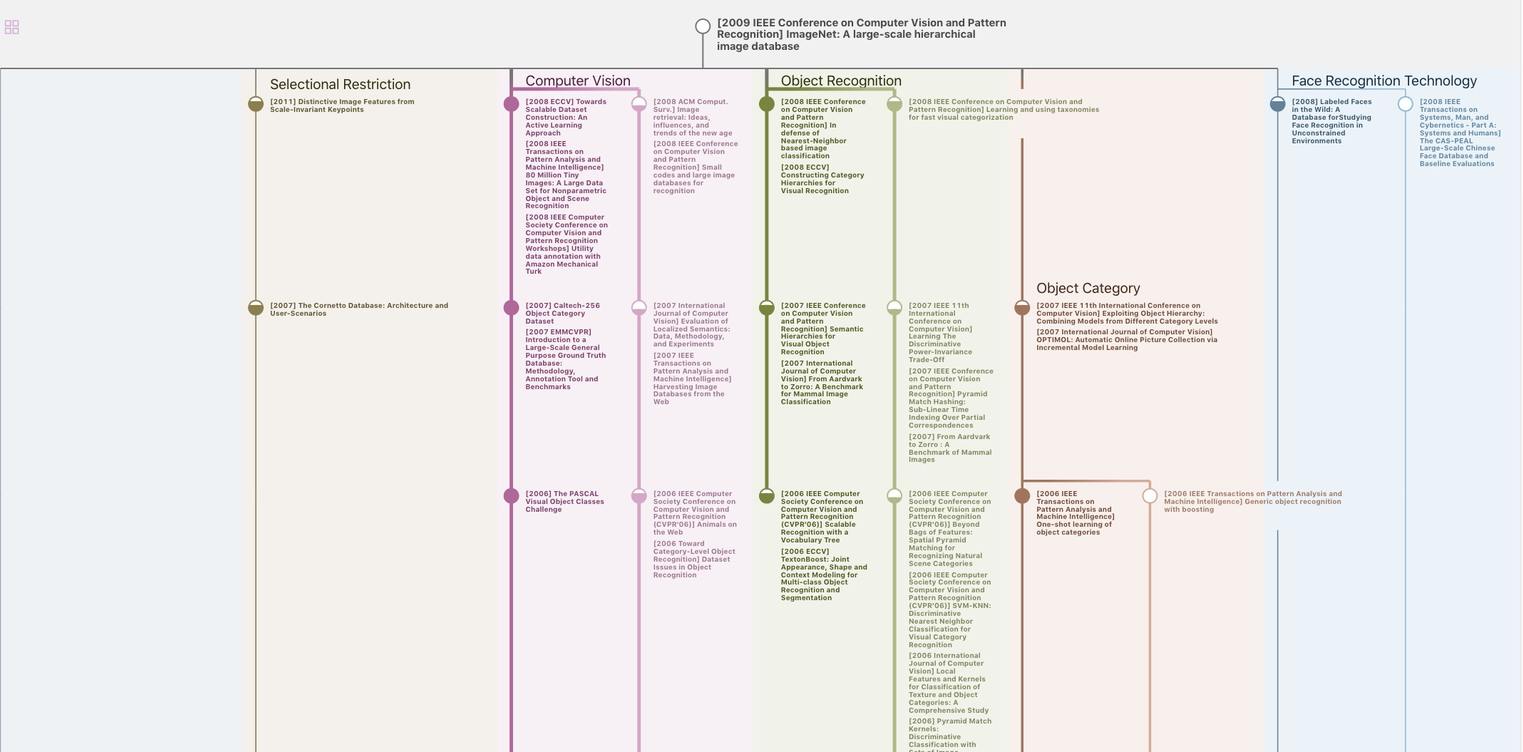
生成溯源树,研究论文发展脉络
Chat Paper
正在生成论文摘要