Evolvability of Machine Learning-based Systems: An Architectural Design Decision Framework
ICSA-C(2023)
摘要
The increasing integration of machine learning (ML) in modern software systems has lead to new challenges as a result of the shift from human-determined behavior to data-determined behavior. One of the key relevant challenges concerns concept drift (CD), i.e., the potential performance degradation due to changes in the data distribution. CD may severely affect the quality of the provided services, being also difficult to predict and detect, as well as costly to address. In this context, we focus on the evolvability of ML-based systems and the architectural considerations in addressing this concern. In this paper, we propose a novel scenario-based framework to support, justify and underpin architectural design decisions that address evolvability concerns in ML-based systems. The applicability and relevance of our framework is outlined through an illustrative example. We envision our framework to be extended to address other quality attributes important to ML-based systems and, overall, provide architectural support for ML operations (MLOps). Finally, we outline our plan to apply it in a number of industrial case studies, evaluate it with practitioners, and iteratively refine it.
更多查看译文
关键词
software architecture,machine learning,design decisions,quality attributes,evolvability,concept drift
AI 理解论文
溯源树
样例
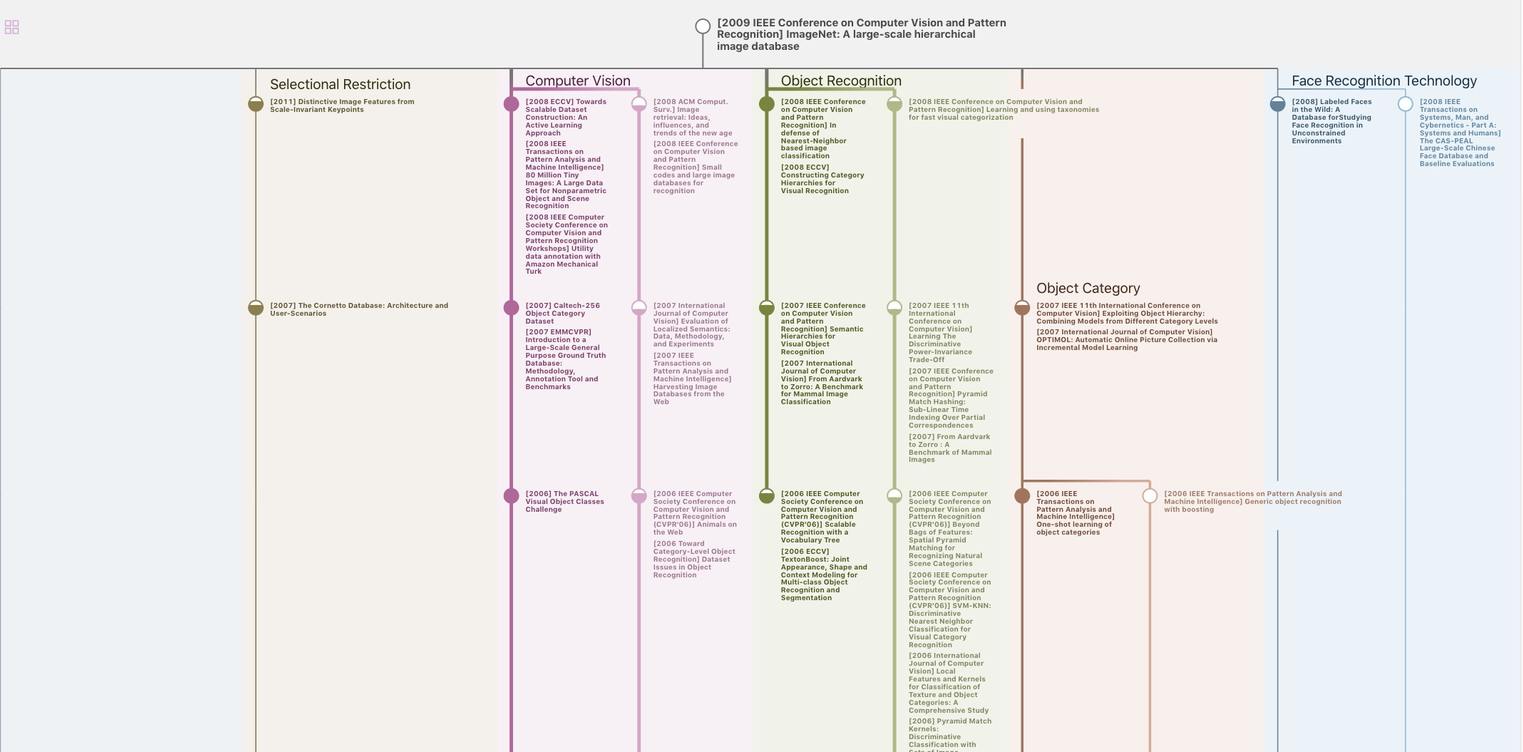
生成溯源树,研究论文发展脉络
Chat Paper
正在生成论文摘要