BS2Vec: A Spatial Representation Learning Model for Large Scale Base Stations.
HPCC/DSS/SmartCity/DependSys(2022)
摘要
With the prosperity of mobile communications, Cell-ID (the unique identifier of a base station) trajectory mining has become popular. To predict locations of base stations (BS) with Cell-ID trajectories and enhance the application of machine learning on Cell-ID trajectory mining, it is essential to learn representations of BSs. Existing methods primarily concentrate on the spatial correlations, which only reflect topological structural relations of BSs, and limit the capability for modeling the BS's representation. To address this issue, we attempt to leverage an unsupervised deep graph embedding technique to learn the representation for large-scale BSs, namely BS2Vec. It incorporates spatial attributes and correlations by developing a variational graph auto encoder with the attention mechanism. The spatial attributes such as vague addresses and Location Area Codes (LAC) imply the coarse-grain location information of BSs, which can significantly enhance the model's ability. To validate BS2Vec, we design a location evaluation task to predict Cell-ID locations merely with Cell-ID trajectories. Experiments on real-world datasets demonstrate the performance gain compared to shallow graph embedding methods. Moreover, it improves the trajectory prediction accuracy from 0.76 to 0.83 compared to the state-of-the-art Cell-ID embedding method.
1
1
These authors contributed to the work equally and should be regarded as co-first authors.
更多查看译文
关键词
representation learning,base station,Cell-ID embedding,graph neural networks
AI 理解论文
溯源树
样例
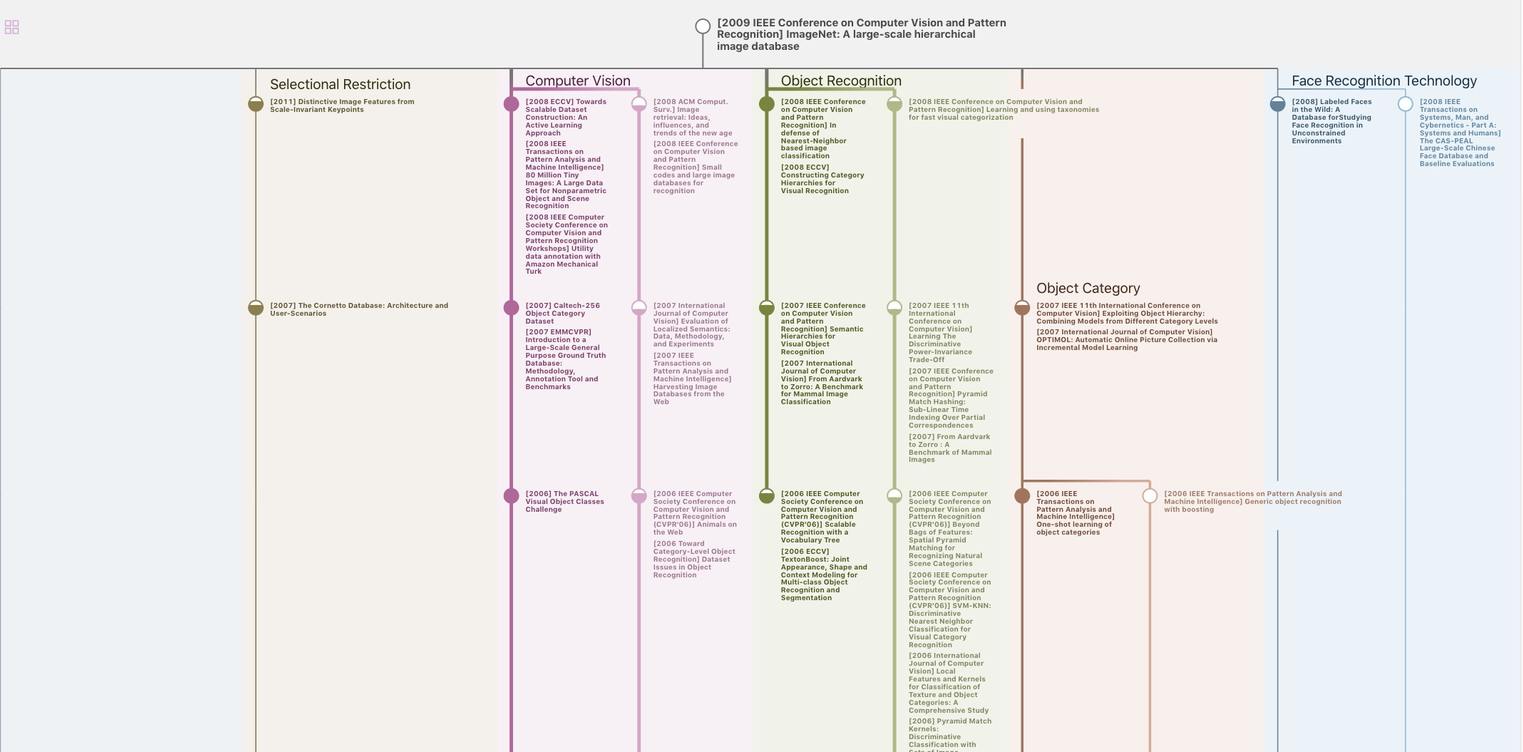
生成溯源树,研究论文发展脉络
Chat Paper
正在生成论文摘要