Exploring the Feasibility of Transformer Based Models on Question Relatedness.
HPCC/DSS/SmartCity/DependSys(2022)
摘要
Professional question answering communities, such as Stack Overflow, are becoming a significant aspect of many intellectual endeavors. As a result, developing a strategy for swiftly locating relevant questions and answers can effectively assist experts in issue solving. Various detection models have been presented to address the problem of question-relatedness prediction, but all are sub-optimal since they cannot effectively capture the long-distance dependency of a long sequence. With the invention of the self-attention mechanism and transformer, a better approach to dealing with question-relatedness prediction has just emerged. The primary objective of this study is to investigate the feasibility of the transformer-based model for question-relatedness problems. We turn the question relatedness problem into a text classification problem and introduce a representative transformer-based model, namely Bidirectional Encoder Representation from Transformers (BERT), to deal with text classification. In our experiment, we show that BERT outperforms both SoftSVM and BiDotLSTM, implying that the transformer-based model has considerable potential to address the challenge in the question-relatedness problem. Furthermore, since we can re-formulate text classification problem into a link prediction problem, we discuss the possibility of incorporating the transformer-based approach and graph representation learning.
更多查看译文
关键词
Question Relatedness Prediction,Self-Attention,Transformer
AI 理解论文
溯源树
样例
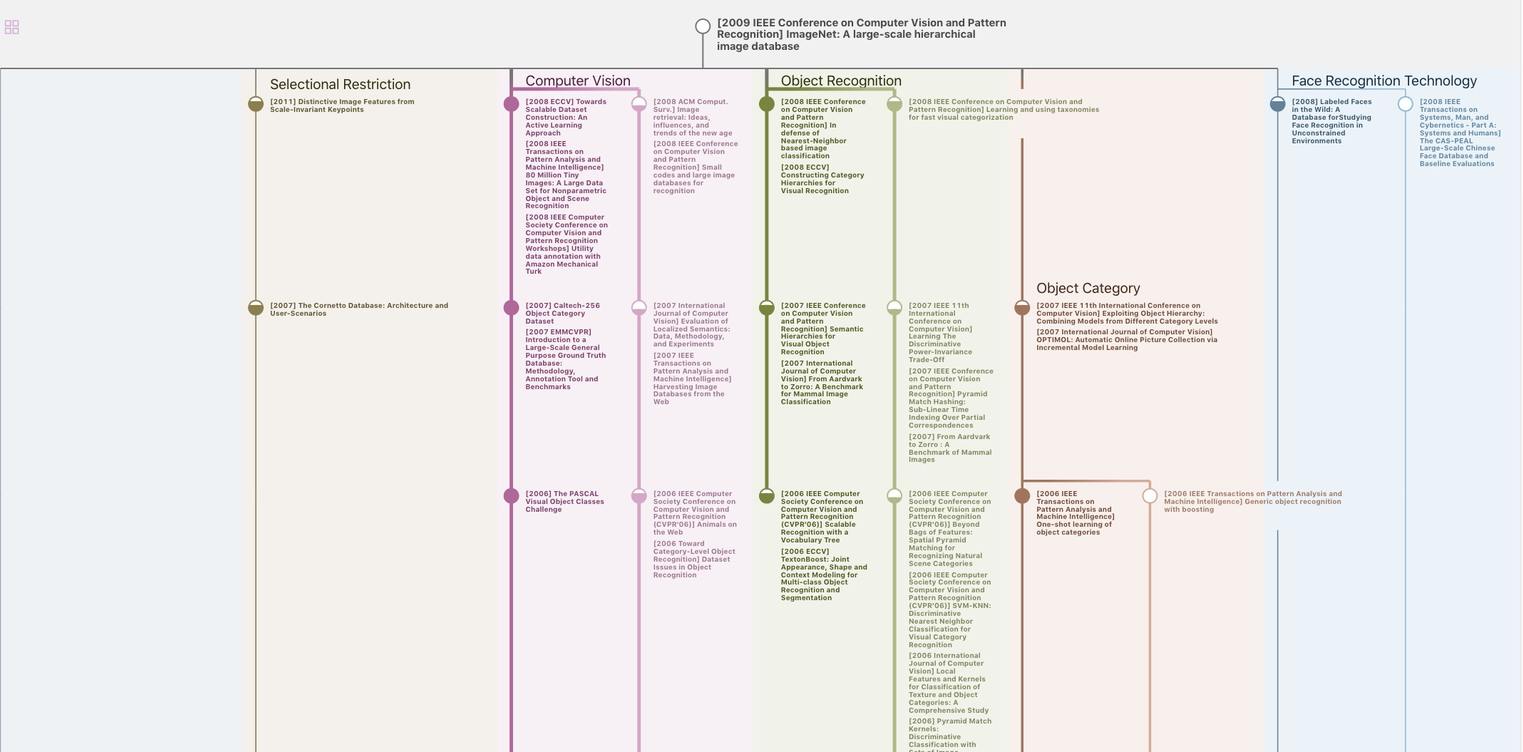
生成溯源树,研究论文发展脉络
Chat Paper
正在生成论文摘要