Feature Difference based Misclassified Sample Detection for CNN Models Deployed in Online Environment
QRS Companion(2022)
摘要
In recent years, Convolutional Neural Network (CNN) has achieved a great success in computer vision. However, at present, for an image classification task, there is no CNN model that can perform 100% accurately due to insufficient or excessive feature learning. Once a CNN model deployed to perform tasks online, misclassified samples might lead the system with the CNN model deployed to enter an unsafe state such as collisions. To assess the performance of such online models, we, in this paper, propose Parallel Signal Routing Paths (PSRP) method to identify misclassified samples by extracting execution paths for each sample and comparing inherent feature differences in terms of CNN nodes between misclassified and well-classified samples, for the ultimate aim of addressing the challenge of test data not having ground-truth labels in online environment where the CNN models are deployed, and give availability results for applying PSRP on 3 public datasets and 3 typical CNN models.
更多查看译文
关键词
Binary classification model, CNN, feature extraction, misclassified sample, well-classified sample
AI 理解论文
溯源树
样例
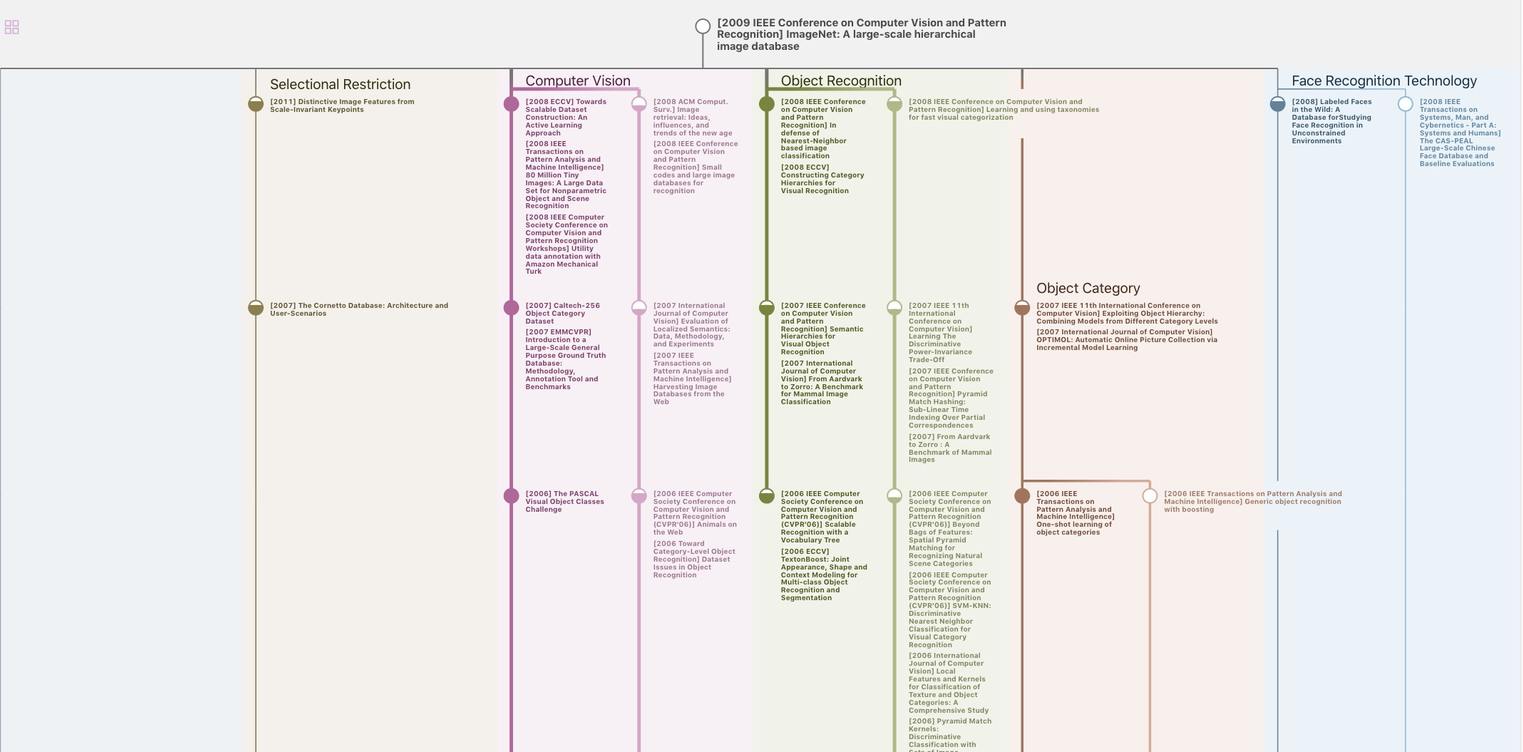
生成溯源树,研究论文发展脉络
Chat Paper
正在生成论文摘要