BoFL: bayesian optimized local training pace control for energy efficient federated learning.
Middleware(2022)
摘要
Federated learning (FL) is a machine learning paradigm that enables a cluster of decentralized edge devices to collaboratively train a shared machine learning model without exposing users' raw data. However, the intensive model training computation is energy-demanding and poses severe challenges to end devices' battery life. In this paper, we present BoFL, a training pace controller deployed on the edge devices that actuates the hardware operational frequencies over multiple configurations to achieve energy-efficient federated learning. BoFL operates in an explore-then-exploit manner within limited rounds of FL tasks. BoFL explores the large hardware frequency space strategically with a tailor-designed Bayesian optimization algorithm. BoFL first finds a set of good operational configurations within few task training rounds, and then exploits these configurations in the remaining rounds to achieve minimized energy consumption for model training. Experiments on multiple real-world edge devices with different FL tasks suggest that BoFL can reduce energy consumption of model training by around 26%, and achieve near-optimal energy efficiency.
更多查看译文
关键词
Federated learning, Energy efficient, Dynamic voltage and frequency scaling, Bayesian optimization
AI 理解论文
溯源树
样例
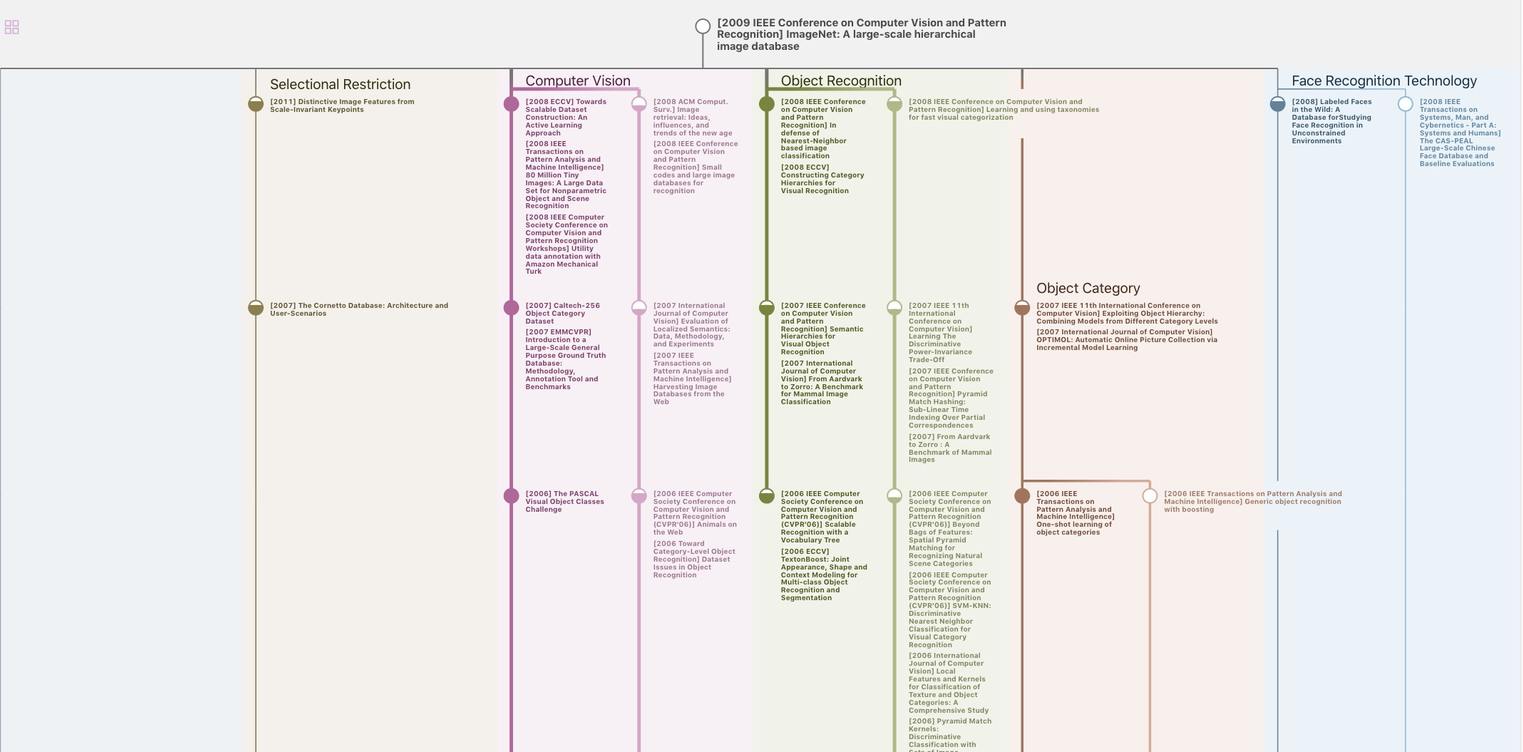
生成溯源树,研究论文发展脉络
Chat Paper
正在生成论文摘要