Two-stage Classification for Detecting Murmurs from Phonocardiograms Using Deep and Expert Features.
CinC(2022)
摘要
Detection of heart murmurs from stethoscope sounds is a key clinical technique used to identify cardiac abnormalities. We describe the creation of an ensemble classifier using both deep and hand-crafted features to screen for heart murmurs and clinical abnormality from phonocar-diogram recordings over multiple auscultation locations. The model was created by the team Murmur Mia! for the George B. Moody PhysioNet Challenge 2022. Methods: Recordings were first filtered through a gradient boosting algorithm to detect Unknown. We assume that these are related to poor quality recordings, and hence we use input features commonly used to assess audio quality. Two further models, a gradient boosting model and ensemble of convolutional neural networks, were trained using time-frequency features and the mel-frequency cepstral coefficients (MFCC) as inputs, respectively. The models were combined using logistic regression, with bespoke rules to convert individual recording outputs to patient predictions. Results: On the hidden challenge test set, our classifier scored 0.755 for the weighted accuracy and 14228 for clinical outcome challenge metric. This placed 9/40 and 28/39 on the challenge leaderboard, for each scoring metric, respectively.
更多查看译文
关键词
cardiac abnormalities,clinical abnormality,convolutional neural networks,deep hand-crafted features,ensemble classifier,heart murmurs,mel-frequency cepstral coefficients,phonocar-diogram recordings,stethoscope sounds,time-frequency features
AI 理解论文
溯源树
样例
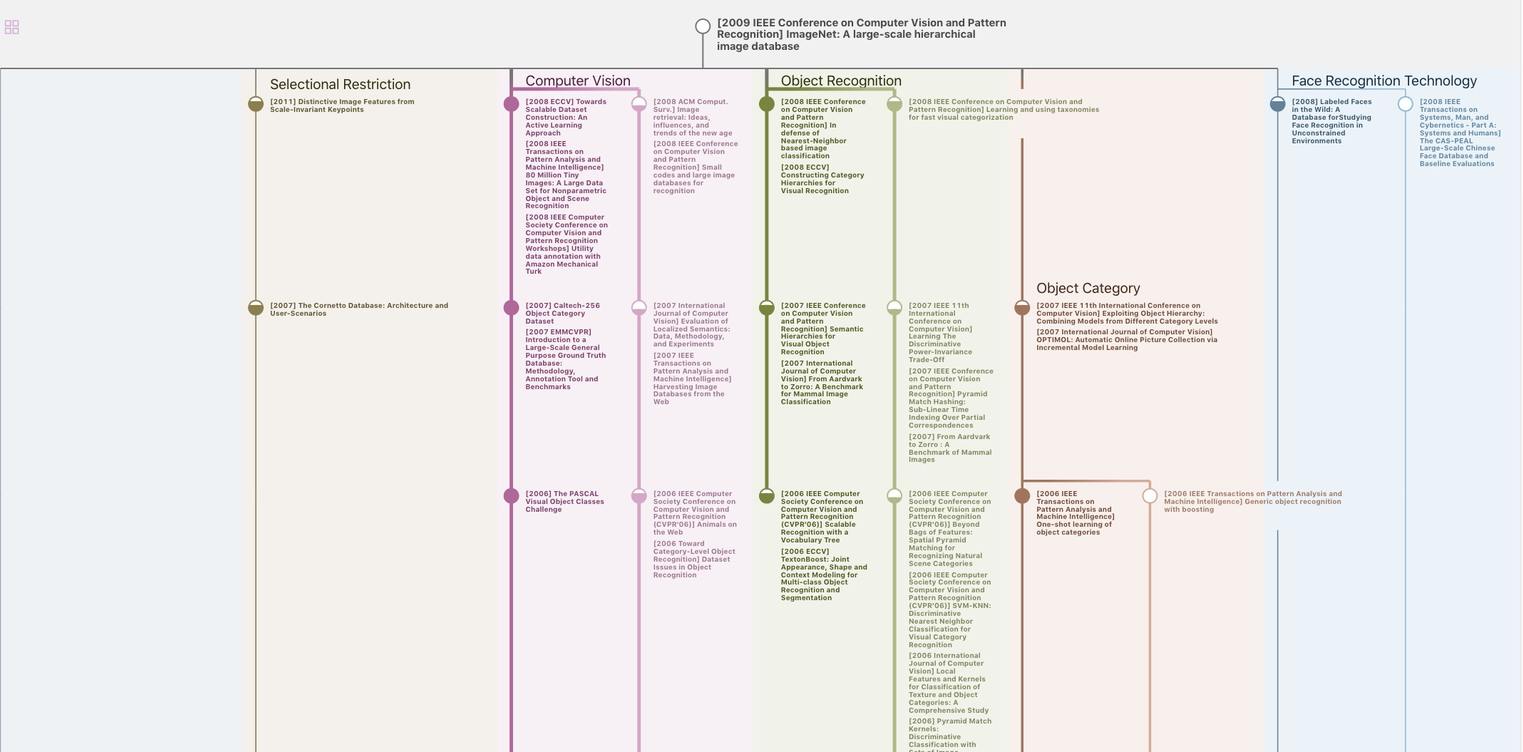
生成溯源树,研究论文发展脉络
Chat Paper
正在生成论文摘要