Segmentation Uncertainty Quantification in Cardiac Propagation Models.
CinC(2022)
摘要
A key part of patient-specific cardiac simulations is segmentation, yet the impact of this subjective and error-prone process hasn't been quantified in most simulation pipelines. In this study we quantify the dependence of a cardiac propagation model on from segmentation variability. We used statistical shape modeling and polynomial Chaos (PC) to capture segmentation variability dependence and applied its affects to a propagation model. We evaluated the predicted local activation times (LATs) an body surface potentials (BSPs) from two modeling pipelines: an EIkonal propagation model and a surface-based fastest route model. The predicted uncertainty due to segmentation shape variability was distributed near the base of the heart and near high amplitude torso potential regions. Our results suggest that modeling pipelines may have to accommodate segmentation errors if regions of interest correspond to high segmentation error. Further, even small errors could proliferate if modeling results are used to to feed further computations, such as ECGI.
更多查看译文
关键词
body surface potentials,cardiac propagation model,EIkonal propagation model,patient-specific cardiac simulations,segmentation errors,segmentation shape variability,segmentation uncertainty quantification,segmentation variability dependence,simulation pipelines,statistical shape modeling,subjective error-prone process,surface-based fastest route model
AI 理解论文
溯源树
样例
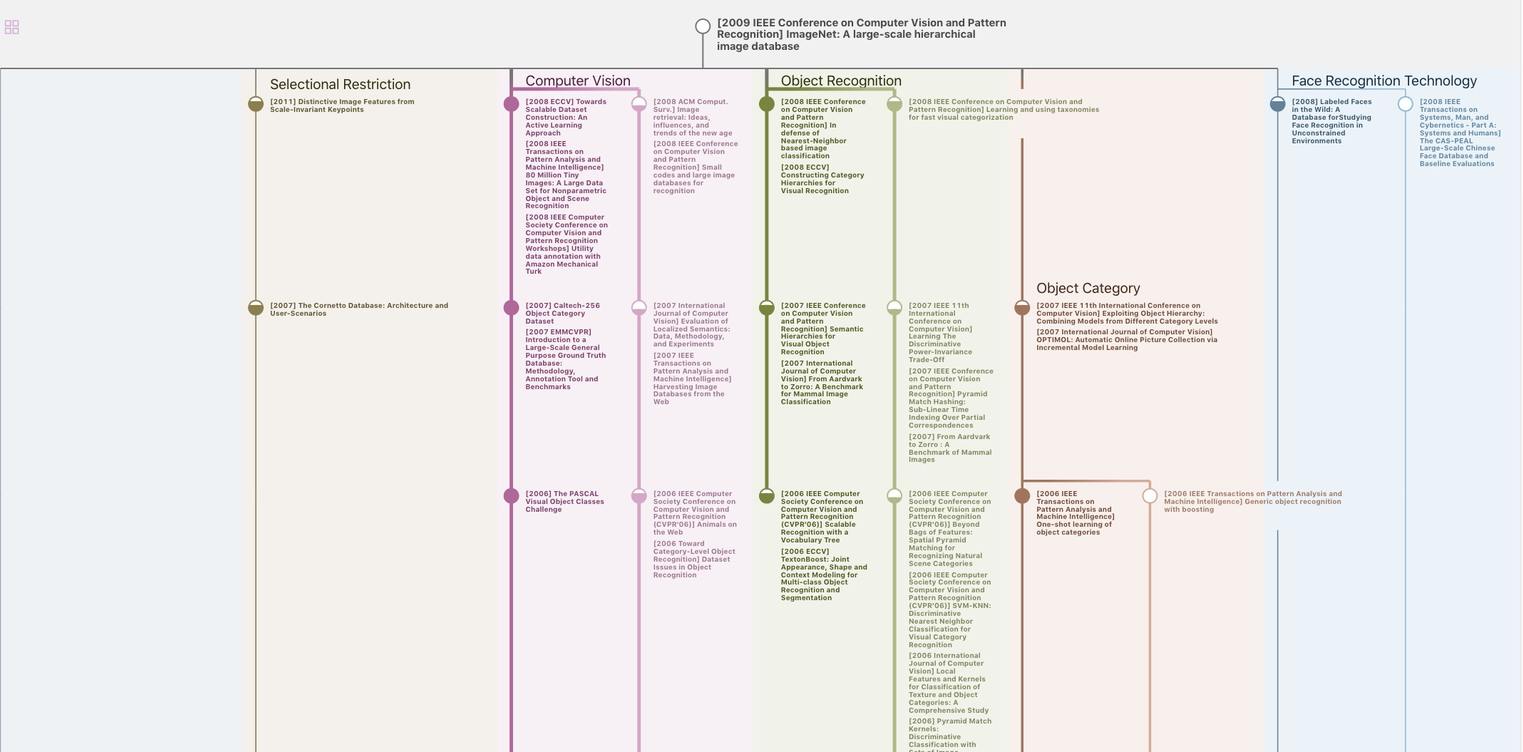
生成溯源树,研究论文发展脉络
Chat Paper
正在生成论文摘要