Non-Invasive Atrial Fibrillation Driver Localization Using Recurrent Neural Networks and Body Surface Potentials.
CinC(2022)
摘要
Ablation is the main therapy to control Atrial Fibrillation (AF). However, the underlying mechanism for AF initiation and maintenance remains mostly unknown and represent a major challenge. ECG Imaging (ECGI) has been presented to address this issue, but it is an ill-posed problem and presents several limitations. Many Deep Learning methods have been proposed for AF characterization, but few provide a solution involving the location of the AF driver. In this work, we propose finding the location of AF drivers using Body Surface Potentials (BSPs) and CNN-LSTM with an attention layer networks as a supervised classification problem. The AF driver was correctly located the 94.42% of the time with an average Cohen's Kappa of 0.87. Hence, the proposed model could provide an effective solution for identifying AF driver location for ablation procedures as a non-invasive approach.
更多查看译文
关键词
AF characterization,AF driver location,attention layer networks,Body Surface Potentials,Deep Learning methods,main therapy,maintenance,noninvasive approach,noninvasive Atrial Fibrillation driver localization,recurrent neural networks
AI 理解论文
溯源树
样例
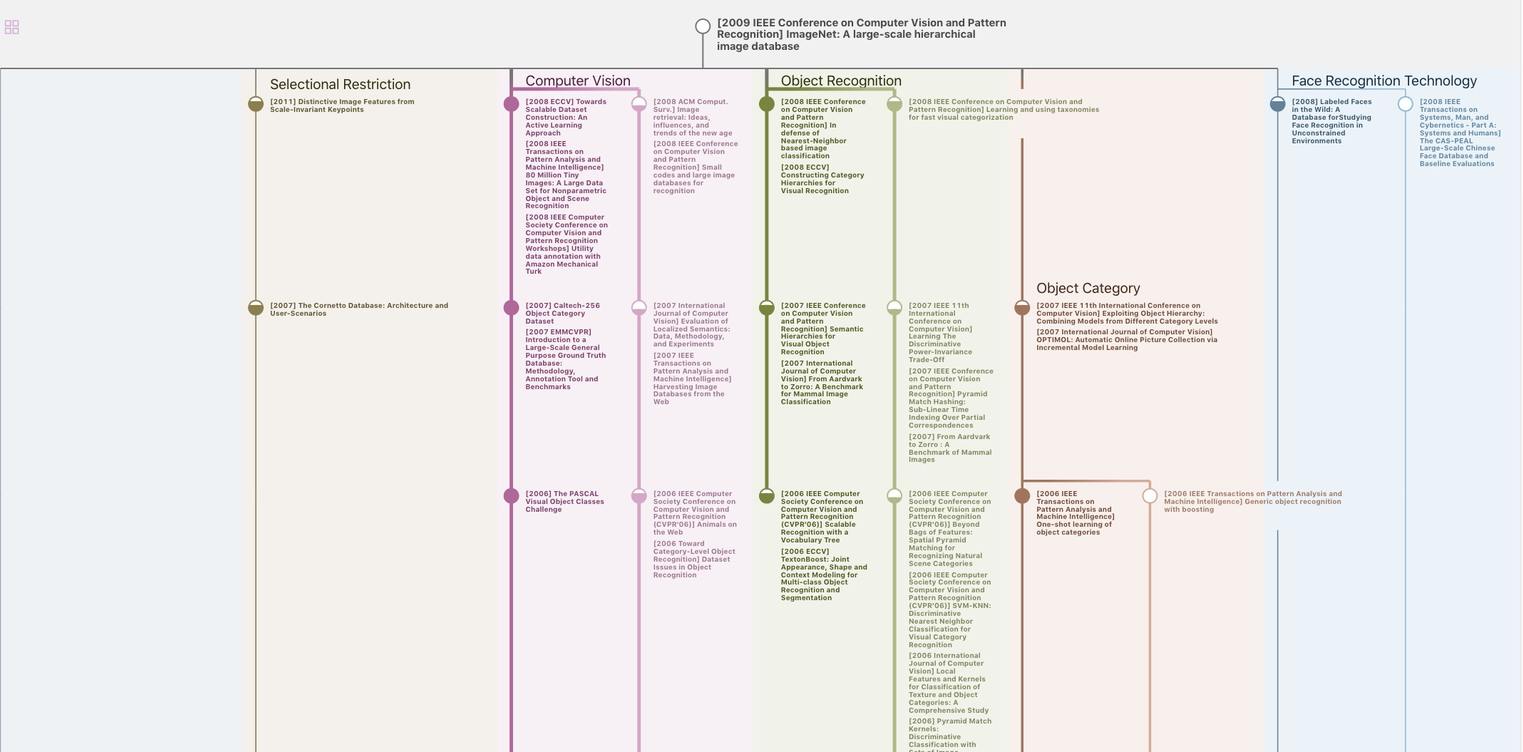
生成溯源树,研究论文发展脉络
Chat Paper
正在生成论文摘要