Uncertainty Quantification of Cardiac Position on Deep Graph Network ECGI.
CinC(2022)
摘要
Subject-specific geometry such as cardiac position and torso size plays an important role in electrocardiographic imaging (ECGI). Previously, we introduced a graph-based neural network (GNN) that is dependent on patient-specific geometry to improve reconstruction accuracy. However, geometric uncertainty, including changes in cardiac position and torso size, has not been addressed in network-based methods. In this study, we estimate geometrical uncertainty on GNN by applying uncertainty quantification with polynomial chaos emulators (PCE). To estimate the effect of geometric variation from common motions, we evaluated the model on samples generated by different heart torso geometries. The experiments shows that the GNN is less sensitive to heart position and torso shape and helps us direct development of similar models to account of possible variability.
更多查看译文
关键词
deep graph network ecgi,cardiac position,uncertainty
AI 理解论文
溯源树
样例
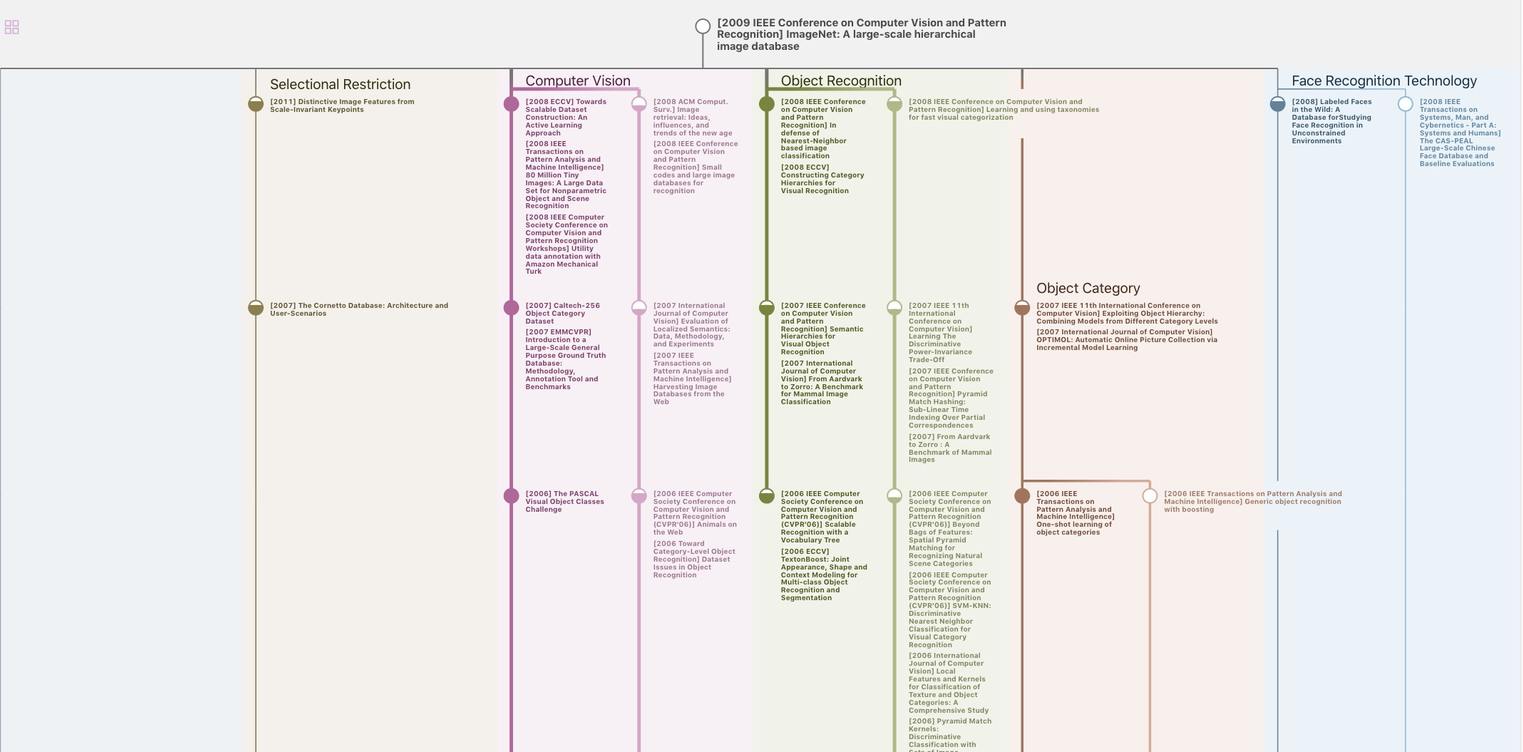
生成溯源树,研究论文发展脉络
Chat Paper
正在生成论文摘要