Adversarial Attacks on Deep Temporal Point Process
ICMLA(2022)
摘要
forecasting to modeling earthquake aftershocks sequences. Due to the flexibility and expressiveness of deep learning, neural network-based approaches have recently shown promise for modeling point process intensities. However, there is a lack of research on the robustness of such models in regards to adversarial attacks and natural shocks to systems. Precisely, while neural point processes may outperform simpler parametric models on in-sample tests, how these models perform when encountering adversarial examples or sharp non-stationary trends remains unknown. Current work proposes several white-box and blackbox adversarial attacks against temporal point processes modeled by deep neural networks. Extensive experiments confirm that predictive performance and parametric modeling of neural point processes are vulnerable to adversarial attacks. Additionally, we evaluate the vulnerability and performance of these models in the presence of non-stationary abrupt changes, using the crimes dataset, during the Covid-19 pandemic, as an example.
更多查看译文
关键词
Point process, Adversarial attacks, Deep learning, Nonparametric modeling
AI 理解论文
溯源树
样例
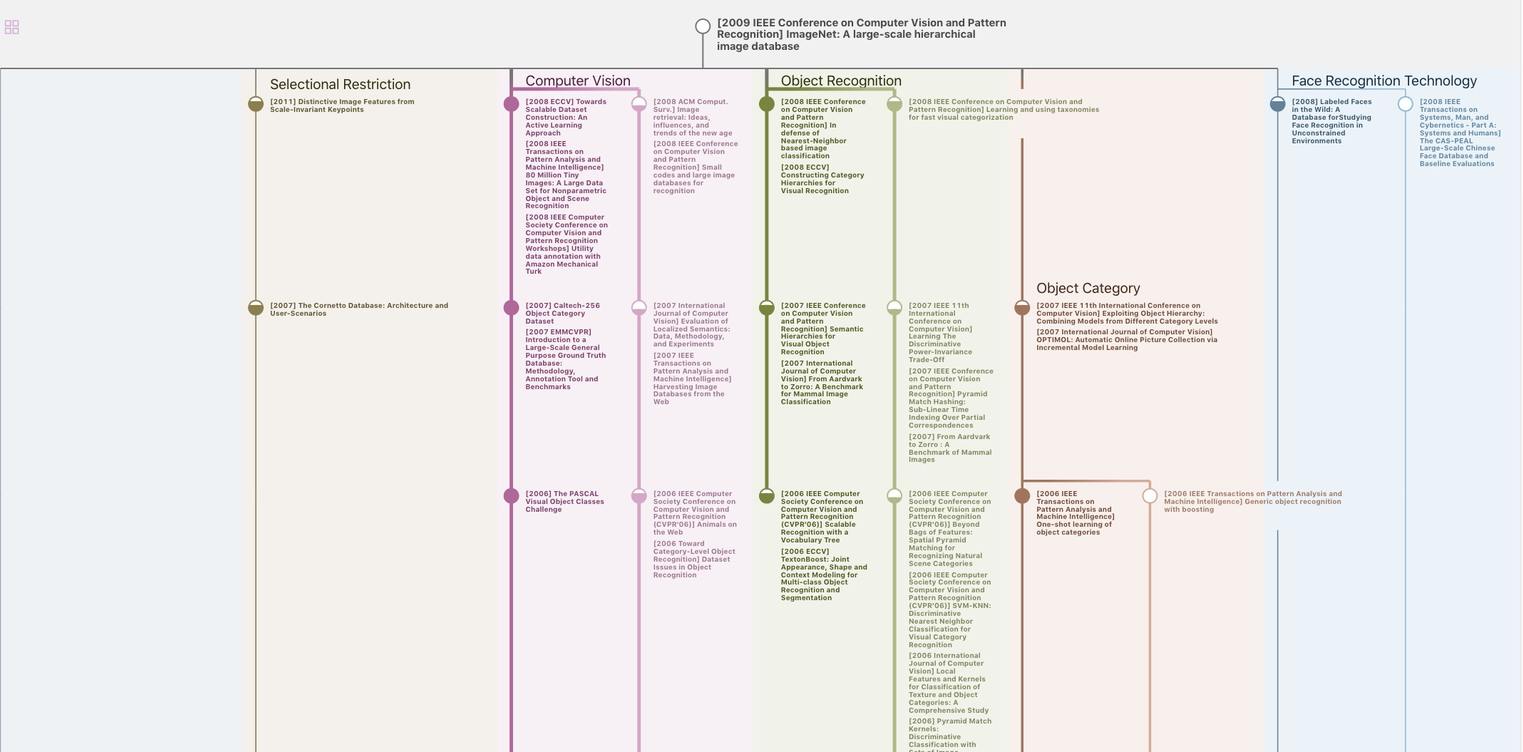
生成溯源树,研究论文发展脉络
Chat Paper
正在生成论文摘要