An improved lightweight high-resolution network based on multi-dimensional weighting for human pose estimation
Scientific Reports(2023)
摘要
Human pose estimation is one of the key technologies in action recognition, motion analysis, human–computer interaction, animation generation etc. How to improve its performance has become a current research hotspot. Lite-HRNet establishes long range connections between keypoints and exhibits good performance in human pose estimation tasks. However, the scale of this method to extract features is relatively single and lacks sufficient information interaction channels. To solve this problem, we propose an improved lightweight high-resolution network based on multi-dimensional weighting, named MDW-HRNet, which is implemented by the following aspects: first, we propose global context modeling, which can learn multi-channel and multi-scale resolution information weights. Second, a cross-channel dynamic convolution module is designed, it performs inter-channel attention aggregation between dynamic and parallel kernels, replacing the basic convolution module. These make the network capable of channel weighting, spatial weighting and convolution weighting. At the same time, we simplify the network structure to perform information exchange and information compensation between high-resolution modules while ensuring speed and accuracy. Experimental results show that our method achieves good performance on both COCO and MPII human pose estimation datasets, and its accuracy surpasses mainstream lightweight pose estimation networks without increasing computational complexity.
更多查看译文
关键词
network,high-resolution,multi-dimensional
AI 理解论文
溯源树
样例
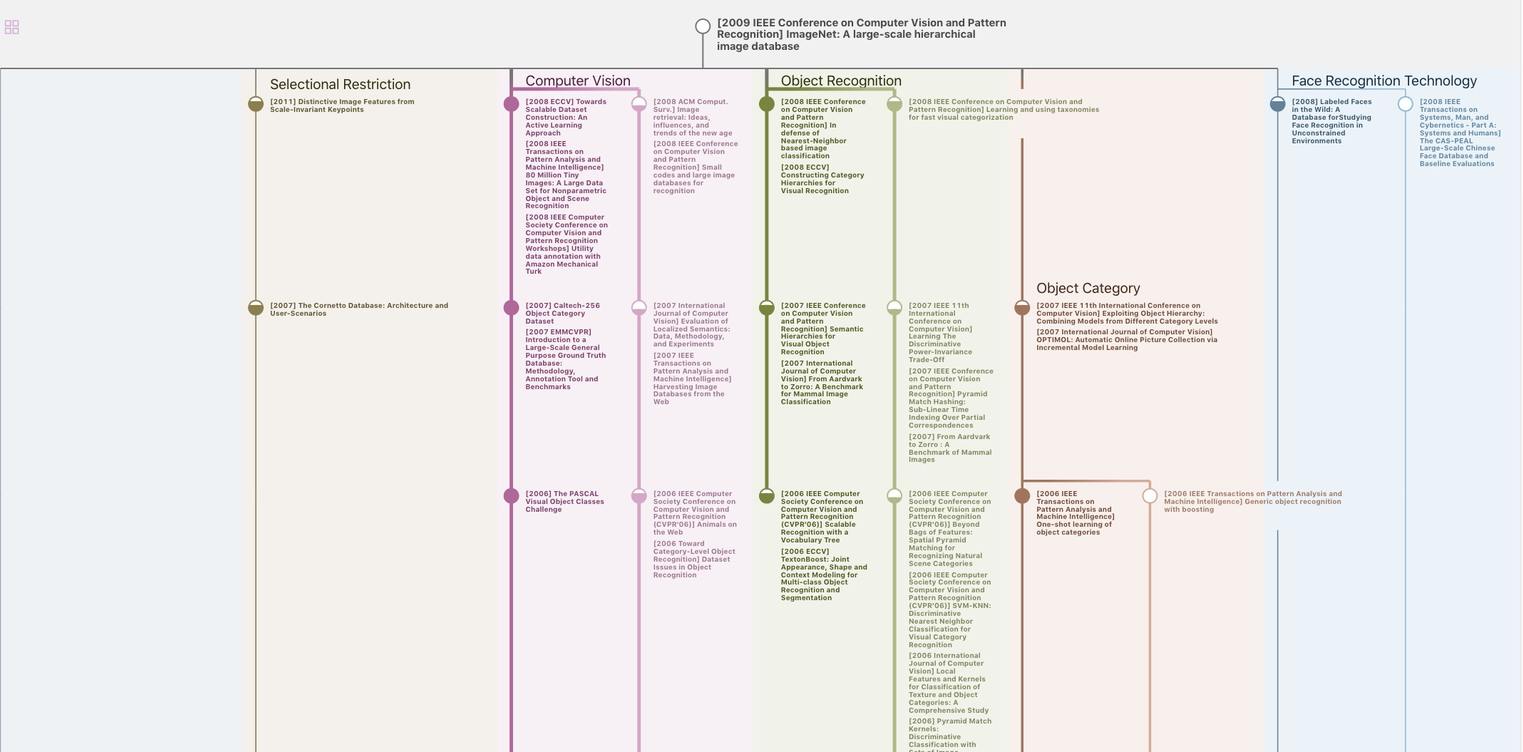
生成溯源树,研究论文发展脉络
Chat Paper
正在生成论文摘要