Deep Learning-based Workflow for Automatic Extraction of Atria and Epicardial Adipose Tissue on cardiac Computed Tomography in Atrial Fibrillation
medRxiv (Cold Spring Harbor Laboratory)(2023)
摘要
Background Preoperative measurements of left atrium (LA) and epicardial adipose tissue (EAT) volumes in computed tomography (CT) images have been reported to be associated with an increased risk of atrial fibrillation (AF) recurrence. We aimed to design a deep learning-based workflow to provide a reliable automatic segmentation of atria, pericardium and EAT, which can facilitate future applications in AF. Methods A total of 157 patients with AF who underwent radiofrequency catheter ablation were enrolled in this study. The 3D U-Net models of LA, right atrium (RA) and pericardium were used to develop the pipeline of total, LA- and RA-EAT automatic segmentation. We defined the attenuation range between -190 to -30 HU as fat within the pericardium to obtain total EAT. Regions between the dilated endocardial boundaries and endocardial walls of LA or RA within the pericardium were used to detect the voxels attributed to fat, resulting in LA-EAT and RA-EAT. Results The LA, RA and pericardium segmentation models achieved Dice coefficients of 0.960 ± 0.010, 0.945 ± 0.013 and 0.967 ± 0.006, respectively. The 3D segmentation models correlated well with ground truth for LA, RA and pericardium (r=0.99 and p < 0.001 for all). For the results of EAT, LA-EAT and RA-EAT segmentation, Dice coefficients of our proposed method were 0.870 ± 0.027, 0.846 ± 0.057 and 0.841 ± 0.071, respectively. Conclusions Our proposed workflow for automatic LA/RA and EAT segmentation applying 3D U-Nets on CT images was reliable in patients with AF.
### Competing Interest Statement
The authors have declared no competing interest.
### Funding Statement
This study was supported by the grant of Taipei Veterans General Hospital (V112B-002), National Science and Technology Council (109-2314-B-010-022-MY3), Veterans General Hospitals and University System of Taiwan Joint Research Program (VGHUST112-G1-3-3).
### Author Declarations
I confirm all relevant ethical guidelines have been followed, and any necessary IRB and/or ethics committee approvals have been obtained.
Yes
The details of the IRB/oversight body that provided approval or exemption for the research described are given below:
Institutional Review Board of Taipei Veterans General Hospital gave ethical approval (IRB number: 2022-06-016AC) for this work and waived the requirement of informed consent.
I confirm that all necessary patient/participant consent has been obtained and the appropriate institutional forms have been archived, and that any patient/participant/sample identifiers included were not known to anyone (e.g., hospital staff, patients or participants themselves) outside the research group so cannot be used to identify individuals.
Yes
I understand that all clinical trials and any other prospective interventional studies must be registered with an ICMJE-approved registry, such as ClinicalTrials.gov. I confirm that any such study reported in the manuscript has been registered and the trial registration ID is provided (note: if posting a prospective study registered retrospectively, please provide a statement in the trial ID field explaining why the study was not registered in advance).
Yes
I have followed all appropriate research reporting guidelines, such as any relevant EQUATOR Network research reporting checklist(s) and other pertinent material, if applicable.
Yes
All data produced in the present study are available upon reasonable request to the authors.
更多查看译文
关键词
cardiac computed tomography,epicardial adipose tissue,atrial fibrillation,adipose tissue,computed tomography,learning-based
AI 理解论文
溯源树
样例
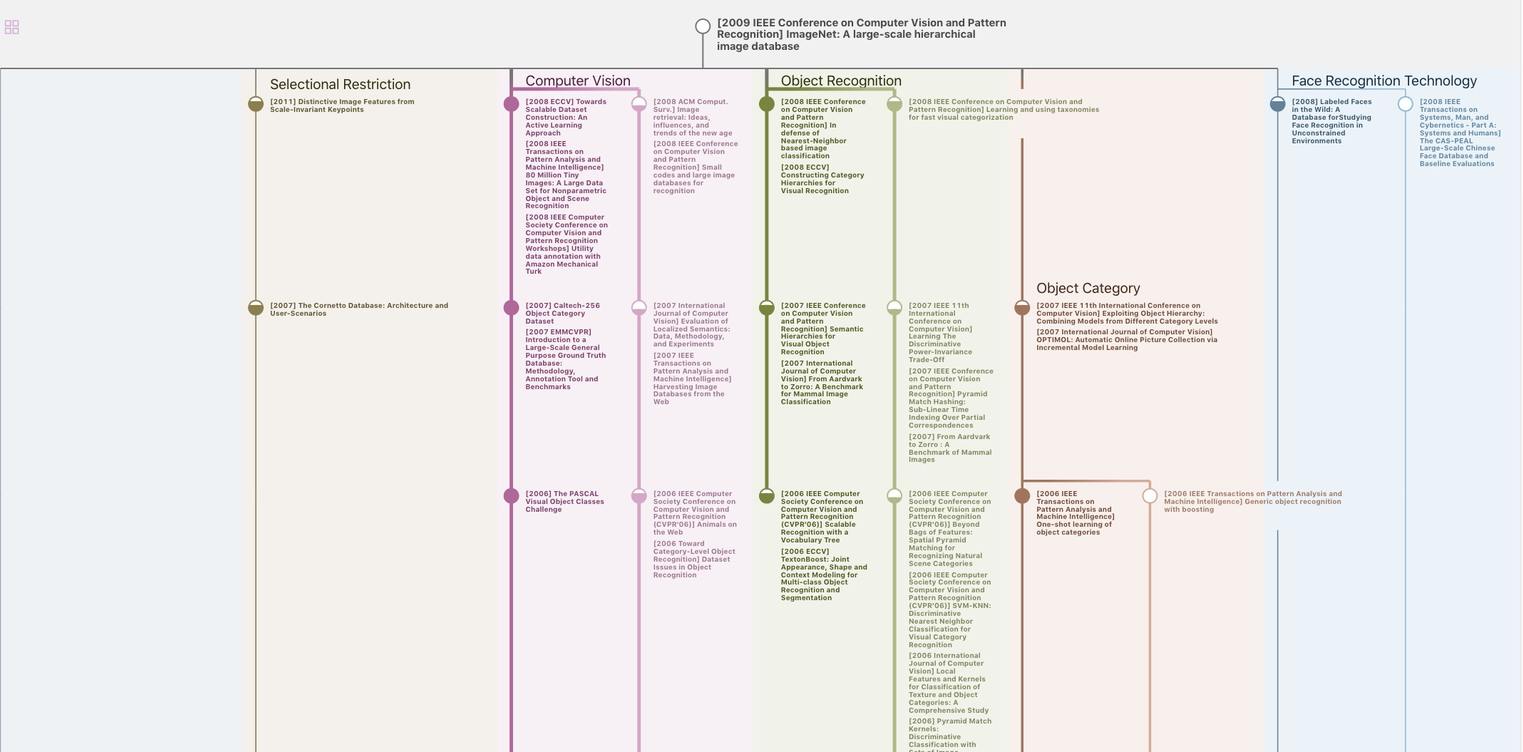
生成溯源树,研究论文发展脉络
Chat Paper
正在生成论文摘要