RoIA: Region of Interest Attention Network for Surface Defect Detection
IEEE Transactions on Semiconductor Manufacturing(2023)
摘要
Surface defect detection plays an important role in manufacturing and has aroused widespread interests. However, it is challenging as defects are highly similar to non-defects. To address this issue, this paper proposes a Region of Interest Attention (RoIA) network based on deep learning for automatically identifying surface defects. It consists of three parts: multi-level feature preservation (MFP) module, region proposal attention (RPA) module, and skip dense connection detection (SDCD) ones, where MFP is designed to differentiate defect features and texture information by feature reserved block, RPA is developed to locate the position of the defects by capturing global and local context information, and SDCD is proposed to better predict defect categories by propagating the fine-grained details from low-level feature map to high-level one. Experimental results conducted on three public datasets (e.g., NEU-DET, DAGM and Magnetic-Tile) demonstrate that the proposed method can significantly improve the detection performance than state-of-the-art ones and achieve an average defect detection accuracy of 99.49%.
更多查看译文
关键词
Surface defect detection,defect similarity,region of interest,region proposal attention (RPA),skip dense connection detection (SDCD)
AI 理解论文
溯源树
样例
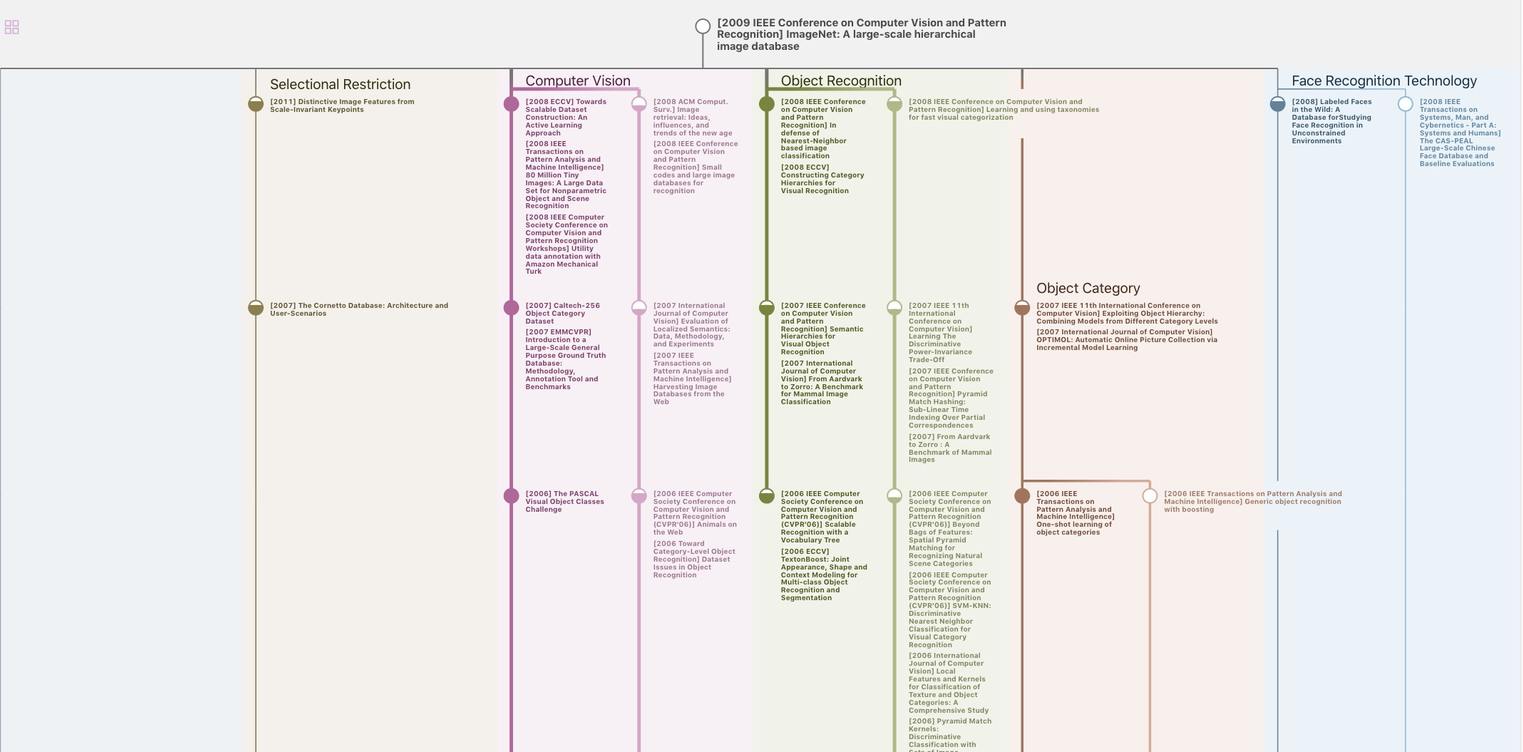
生成溯源树,研究论文发展脉络
Chat Paper
正在生成论文摘要