Semi-Supervised Semantic Segmentation with Structured Output Space Adaption
ICASSP 2023 - 2023 IEEE International Conference on Acoustics, Speech and Signal Processing (ICASSP)(2023)
摘要
Semi-supervised semantic segmentation methods rely on dense pixel-level classification with limited data and can thus be developed to adapt source ground truth labels to a target domain. In this paper, we creatively propose a method for semi-supervised semantic segmentation. The key innovation is our adversarial learning method for space adaptation in context, which can be regarded as a structured output that contains spatial similarities between unlabeled data and labeled data. To achieve this, we construct an adversarial learning network to efficiently adapt to the structural output space in labeled and unlabeled similar samples. Furthermore, we introduce two learning strategies into semi-supervised semantic segmentation, one that can selectively capture intra-category and inter-category context dependencies, resulting in robust feature representations. While the other explicitly concatenates the shape information of objects as a separate processing branch to produce sharper predicted boundaries of objects. Experimental results on two well-known benchmark datasets show that our method achieves better performance compared to the previous competitive models.
更多查看译文
关键词
Semantic segmentation,semi-supervised learning,generative adversarial networks
AI 理解论文
溯源树
样例
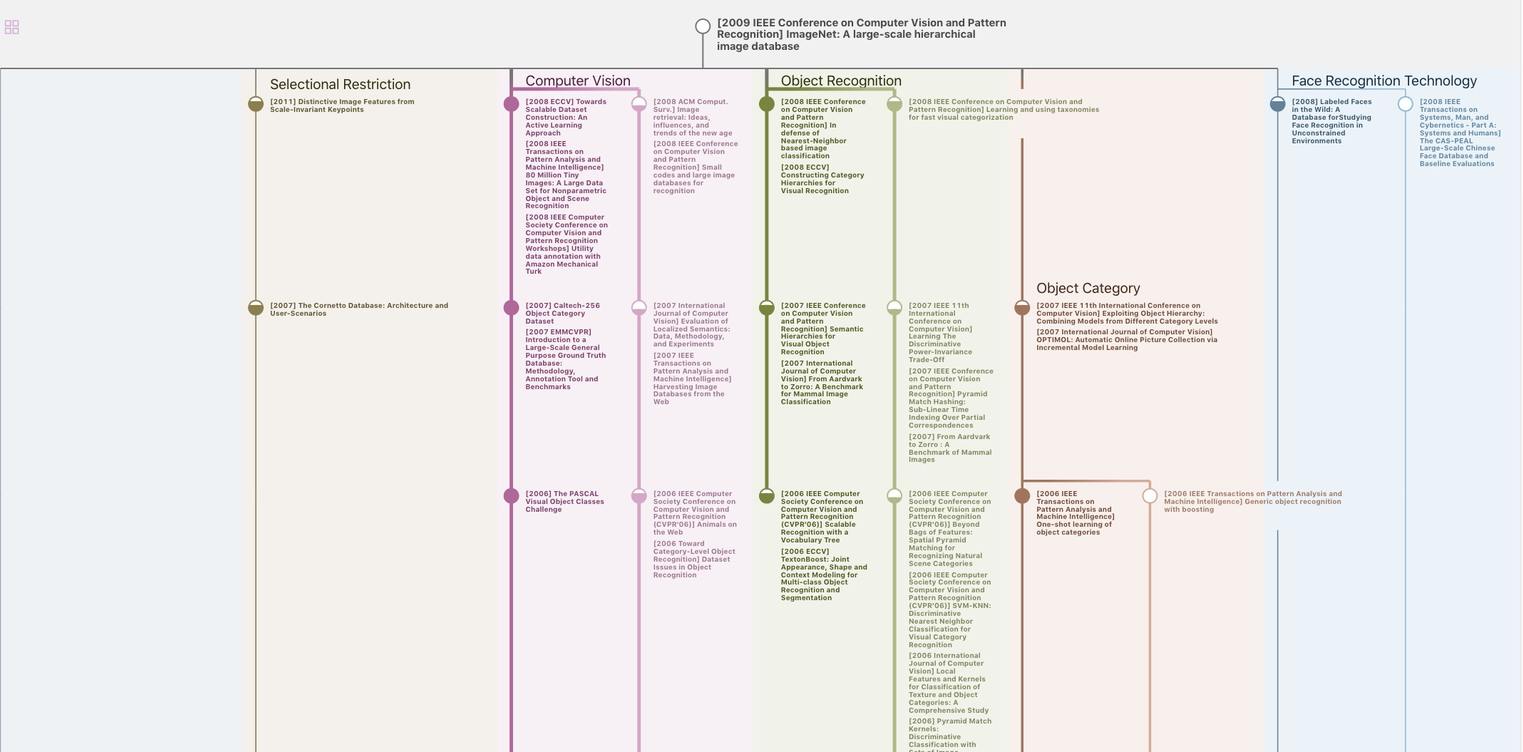
生成溯源树,研究论文发展脉络
Chat Paper
正在生成论文摘要