IoT-Based Android Malware Detection Using Graph Neural Network With Adversarial Defense
IEEE Internet of Things Journal(2023)
摘要
Since the Internet of Things (IoT) is widely adopted using Android applications, detecting malicious Android apps is essential. In recent years, Android graph-based deep learning research has proposed many approaches to extract relationships from the application as a graph to generate graph embeddings. First, we demonstrate the effectiveness of graph-based classification using graph neural networks (GNNs)-based classifier to generate API graph embedding. The graph embedding is used with “Permission” and “Intent” to train multiple machine learning and deep learning algorithms to detect Android malware. The classification achieved an accuracy of 98.33% in CICMaldroid and 98.68% in the Drebin data set. However, the graph-based deep learning is vulnerable as an attacker can add fake relationships to avoid detection by the classifier. Second, we propose a generative adversarial network (GAN)-based algorithm named VGAE-MalGAN to attack the graph-based GNN Android malware classifier. The VGAE-MalGAN generator generates adversarial malware API graphs, and the VGAE-MalGAN substitute detector (SD) tries to fit the detector. Experimental analysis shows that VGAE-MalGAN can effectively reduce the detection rate of GNN malware classifiers. Although the model fails to detect adversarial malware, experimental analysis shows that retraining the model with generated adversarial samples helps to combat adversarial attacks.
更多查看译文
关键词
Android,deep learning,generative adversarial network (GAN),graph neural network (GNN),Internet of Things (IoT),machine learning
AI 理解论文
溯源树
样例
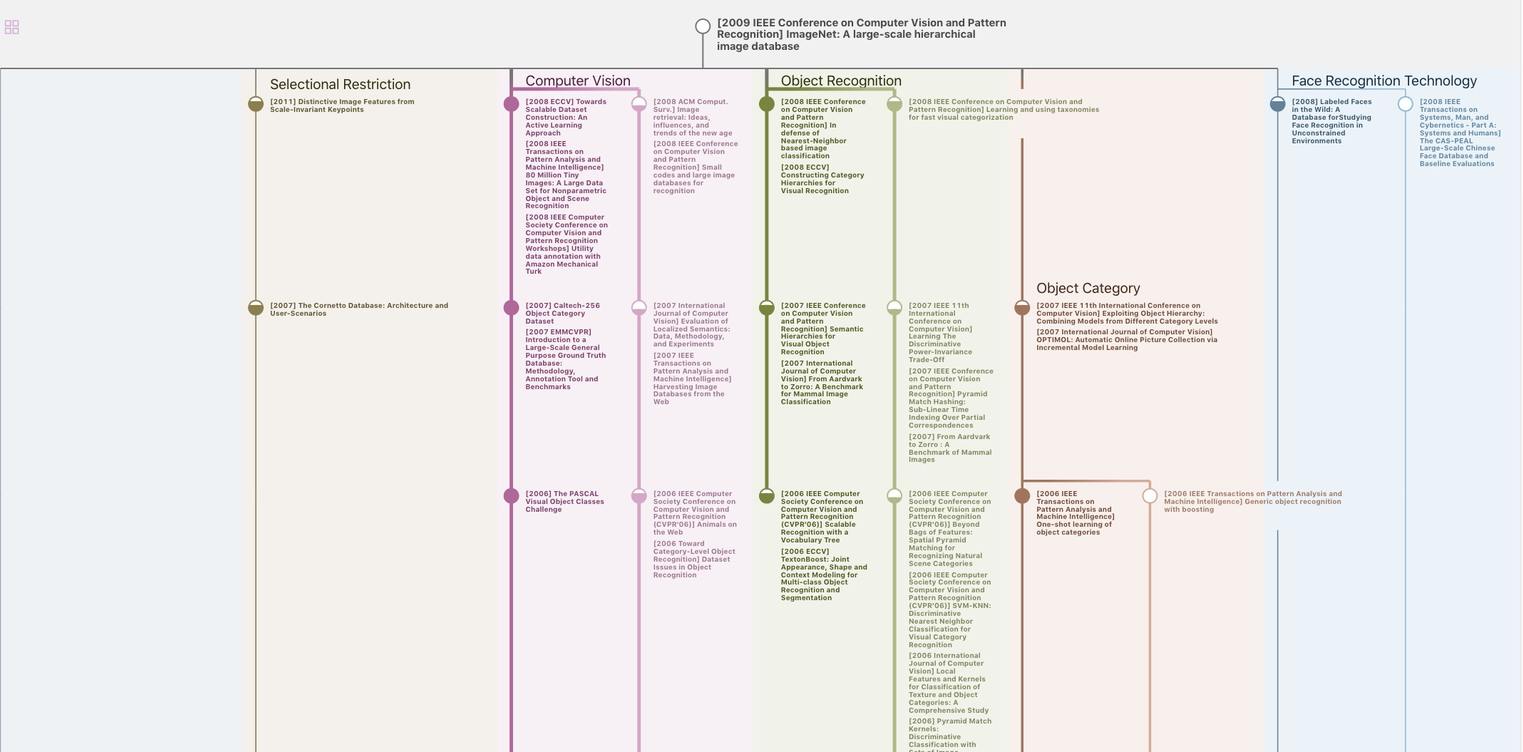
生成溯源树,研究论文发展脉络
Chat Paper
正在生成论文摘要