Tiny-PPG: A lightweight deep neural network for real-time detection of motion artifacts in photoplethysmogram signals on edge devices
INTERNET OF THINGS(2024)
摘要
Photoplethysmogram (PPG) signals are easily contaminated by motion artifacts in real-world settings, despite their widespread use in Internet-of-Things (IoT) based wearable and smart health devices for cardiovascular health monitoring. This study proposed a lightweight deep neural network, called Tiny-PPG, for accurate and real-time PPG artifact segmentation on IoT edge devices. The model was trained and tested on a public dataset, PPG DaLiA, which featured complex artifacts with diverse lengths and morphologies during various daily activities of 15 subjects using a watch-type device (Empatica E4). The model structure, training method and loss function were specifically designed to balance detection accuracy and speed for real-time PPG artifact detection in resource-constrained embedded devices. To optimize the model size and capability in multi-scale feature representation, the model employed depth-wise separable convolution and atrous spatial pyramid pooling modules, respectively. Additionally, the contrastive loss was also utilized to further optimize the feature embeddings. With additional model pruning, Tiny-PPG achieved state-of-the-art detection accuracy of 87.4% while only having 19,726 model parameters (0.15 megabytes), and was successfully deployed on an STM32 embedded system for real-time PPG artifact detection. Therefore, this study provides an effective solution for resource-constraint IoT smart health devices in PPG artifact detection.
更多查看译文
关键词
Edge AI,IoT wearables,Photoplethysmogram,Motion artifacts
AI 理解论文
溯源树
样例
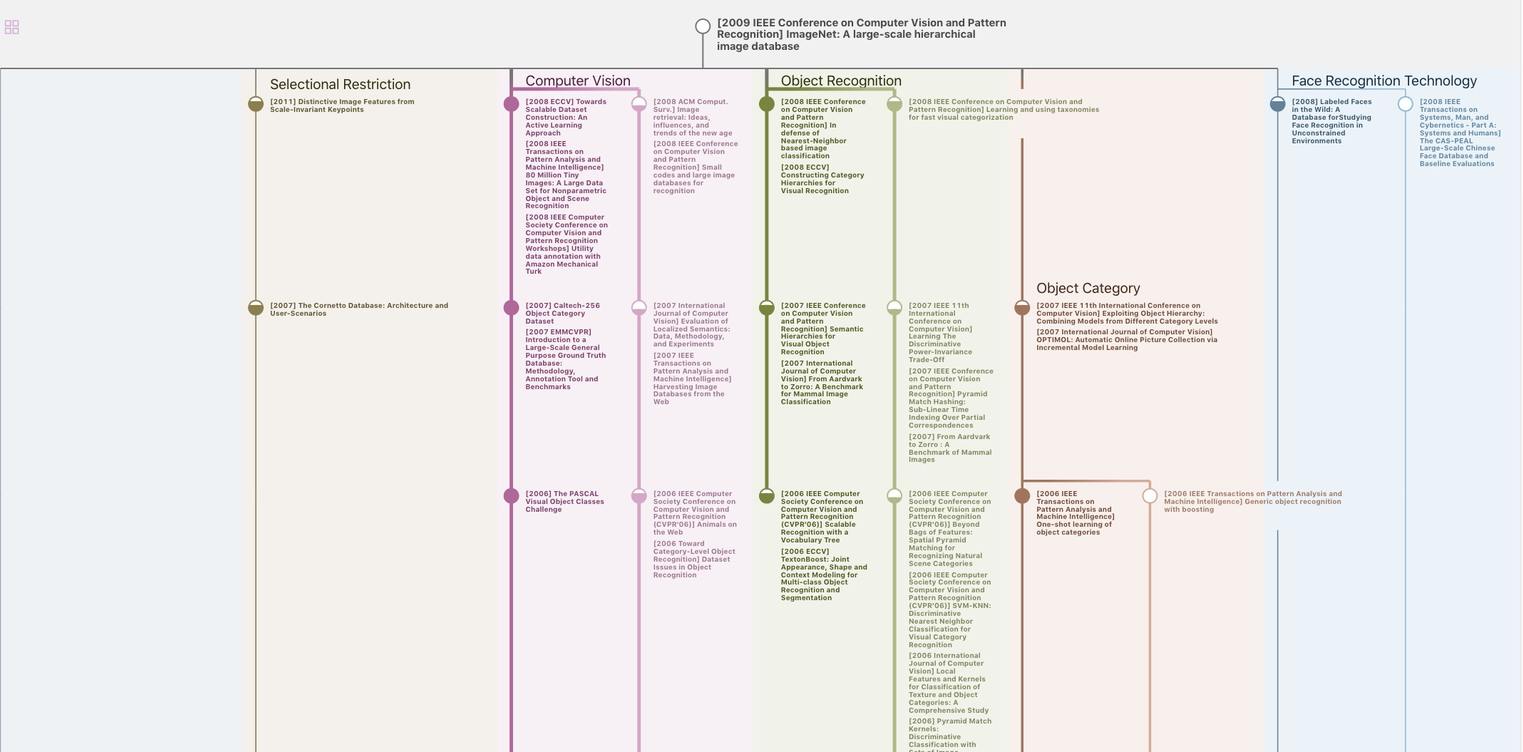
生成溯源树,研究论文发展脉络
Chat Paper
正在生成论文摘要