Learned Wyner-Ziv Compressors Recover Binning
2023 IEEE International Symposium on Information Theory (ISIT)(2023)
摘要
We consider lossy compression of an information source when the decoder has lossless access to a correlated one. This setup, also known as the Wyner-Ziv problem, is a special case of distributed source coding. To this day, real-world applications of this problem have neither been fully developed nor heavily investigated. We propose a data-driven method based on machine learning that leverages the universal function approximation capability of artificial neural networks. We find that our neural network-based compression scheme re-discovers some principles of the optimum theoretical solution of the Wyner-Ziv setup, such as binning in the source space as well as linear decoder behavior within each quantization index, for the quadratic-Gaussian case. These behaviors emerge although no structure exploiting knowledge of the source distributions was imposed. Binning is a widely used tool in information theoretic proofs and methods, and to our knowledge, this is the first time it has been explicitly observed to emerge from data-driven learning.
更多查看译文
关键词
artificial neural networks,data-driven learning,data-driven method,distributed source coding,information source,information theoretic proofs,learned Wyner-Ziv compressors recover binning,linear decoder behavior,lossless access,lossy compression,machine learning,neural network-based compression scheme re-discovers,optimum theoretical solution,quadratic-Gaussian case,real-world applications,source distributions,source space,universal function approximation capability,Wyner-Ziv problem,Wyner-Ziv setup
AI 理解论文
溯源树
样例
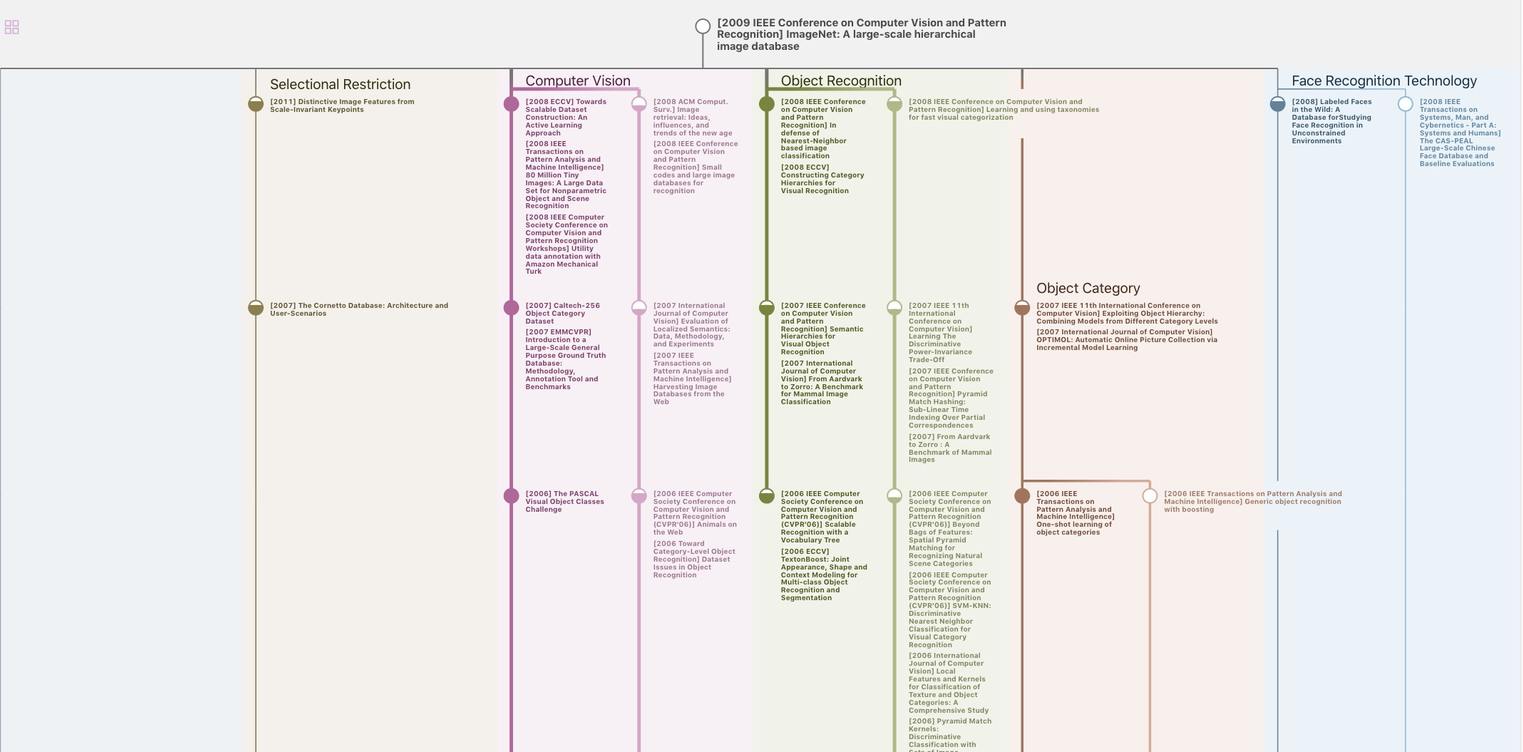
生成溯源树,研究论文发展脉络
Chat Paper
正在生成论文摘要