Learning to Evaluate the Artness of AI-generated Images
CoRR(2023)
摘要
Assessing the artness of AI-generated images continues to be a challenge within the realm of image generation. Most existing metrics cannot be used to perform instance-level and reference-free artness evaluation. This paper presents ArtScore, a metric designed to evaluate the degree to which an image resembles authentic artworks by artists (or conversely photographs), thereby offering a novel approach to artness assessment. We first blend pre-trained models for photo and artwork generation, resulting in a series of mixed models. Subsequently, we utilize these mixed models to generate images exhibiting varying degrees of artness with pseudo-annotations. Each photorealistic image has a corresponding artistic counterpart and a series of interpolated images that range from realistic to artistic. This dataset is then employed to train a neural network that learns to estimate quantized artness levels of arbitrary images. Extensive experiments reveal that the artness levels predicted by ArtScore align more closely with human artistic evaluation than existing evaluation metrics, such as Gram loss and ArtFID.
更多查看译文
关键词
artness,images,learning,ai-generated
AI 理解论文
溯源树
样例
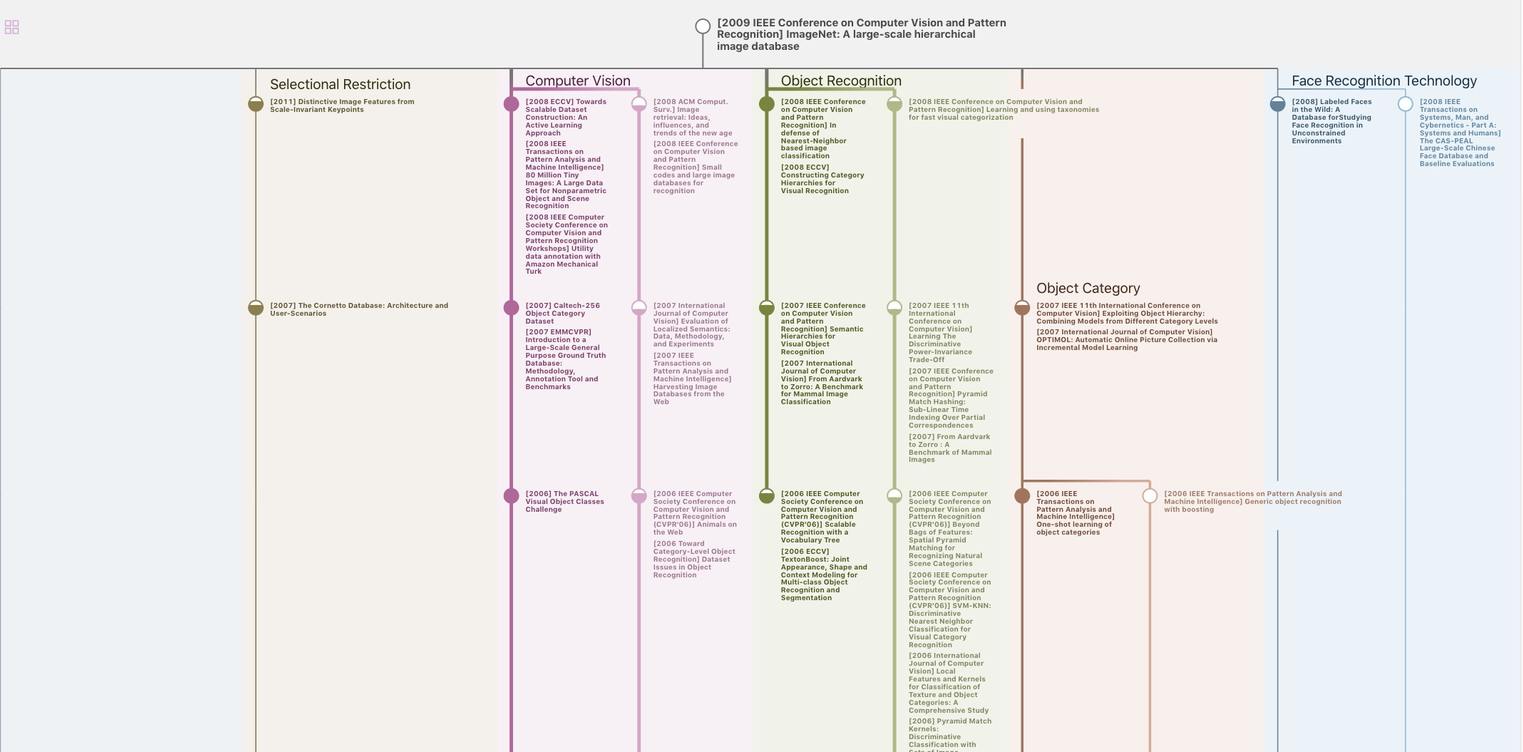
生成溯源树,研究论文发展脉络
Chat Paper
正在生成论文摘要