Semi-Supervised Federated Learning for Keyword Spotting
CoRR(2023)
摘要
Keyword Spotting (KWS) is a critical aspect of audio-based applications on mobile devices and virtual assistants. Recent developments in Federated Learning (FL) have significantly expanded the ability to train machine learning models by utilizing the computational and private data resources of numerous distributed devices. However, existing FL methods typically require that devices possess accurate ground-truth labels, which can be both expensive and impractical when dealing with local audio data. In this study, we first demonstrate the effectiveness of Semi-Supervised Federated Learning (SSL) and FL for KWS. We then extend our investigation to Semi-Supervised Federated Learning (SSFL) for KWS, where devices possess completely unlabeled data, while the server has access to a small amount of labeled data. We perform numerical analyses using state-of-the-art SSL, FL, and SSFL techniques to demonstrate that the performance of KWS models can be significantly improved by leveraging the abundant unlabeled heterogeneous data available on devices.
更多查看译文
关键词
Spotting,Semi-Supervised Learning,Federated Learning,Semi-Supervised Federated Learning
AI 理解论文
溯源树
样例
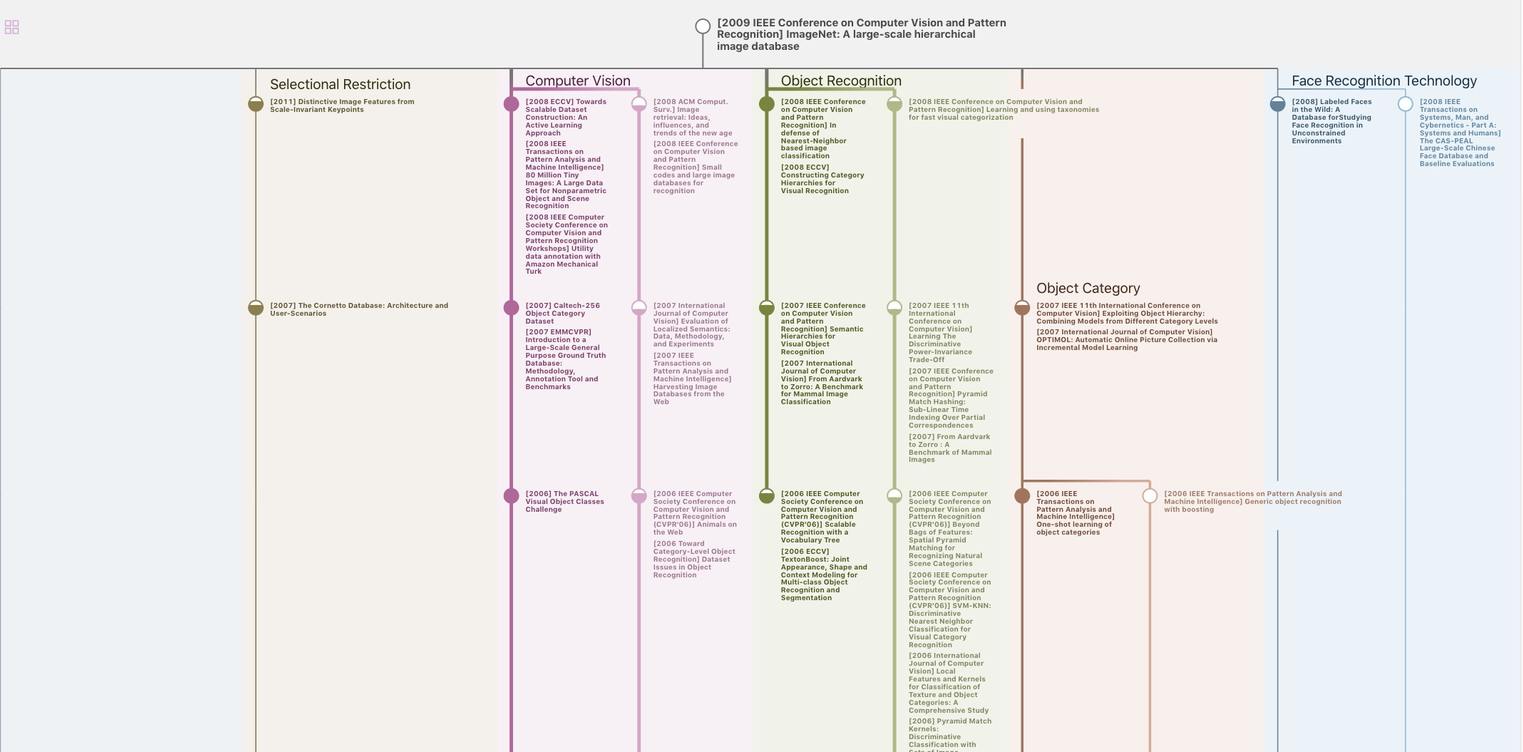
生成溯源树,研究论文发展脉络
Chat Paper
正在生成论文摘要