Minimal Learning Machine for Multi-Label Learning
CoRR(2023)
摘要
Distance-based supervised method, the minimal learning machine, constructs a predictive model from data by learning a mapping between input and output distance matrices. In this paper, we propose methods and evaluate how this technique and its core component, the distance mapping, can be adapted to multi-label learning. The proposed approach is based on combining the distance mapping with an inverse distance weighting. Although the proposal is one of the simplest methods in the multi-label learning literature, it achieves state-of-the-art performance for small to moderate-sized multi-label learning problems. Besides its simplicity, the proposed method is fully deterministic and its hyper-parameter can be selected via ranking loss-based statistic which has a closed form, thus avoiding conventional cross-validation-based hyper-parameter tuning. In addition, due to its simple linear distance mapping-based construction, we demonstrate that the proposed method can assess predictions' uncertainty for multi-label classification, which is a valuable capability for data-centric machine learning pipelines.
更多查看译文
关键词
learning,machine,multi-label
AI 理解论文
溯源树
样例
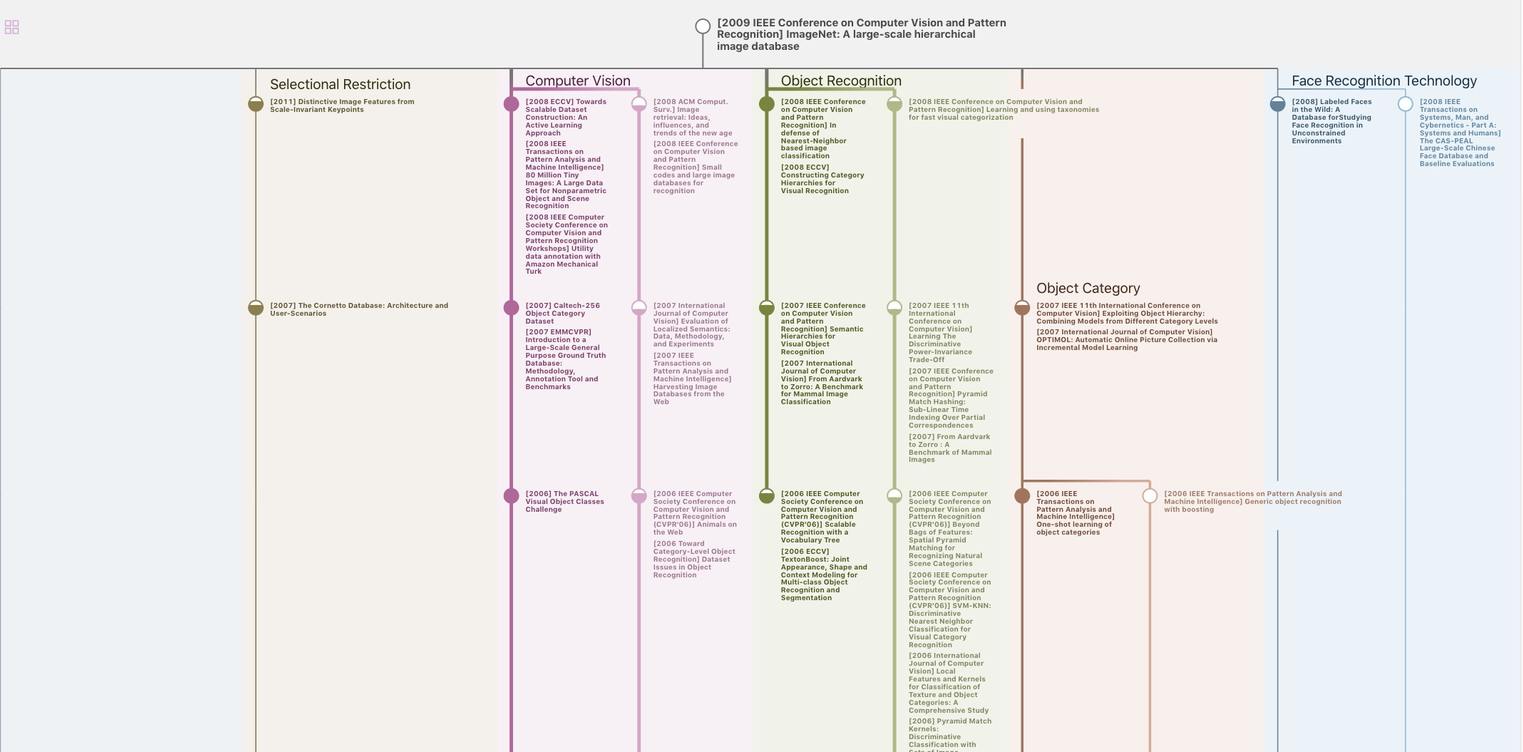
生成溯源树,研究论文发展脉络
Chat Paper
正在生成论文摘要