Performance of the low-latency GstLAL inspiral search towards LIGO, Virgo, and KAGRA's fourth observing run
PHYSICAL REVIEW D(2024)
摘要
GstLAL is a stream -based matched -filtering search pipeline aiming at the prompt discovery of gravitational waves from compact binary coalescences such as the mergers of black holes and neutron stars. Over the past three observation runs by the LIGO, Virgo, and KAGRA Collaboration, the GstLAL search pipeline has participated in several tens of gravitational wave discoveries. The fourth observing run (O4) is set to begin in May 2023 and is expected to see the discovery of many new and interesting gravitational wave signals which will inform our understanding of astrophysics and cosmology. We describe the current configuration of the GstLAL low -latency search and show its readiness for the upcoming observation run by presenting its performance on a mock data challenge. The mock data challenge includes 40 days of LIGO Hanford, LIGO Livingston, and Virgo strain data along with an injection campaign in order to fully characterize the performance of the search. We find an improved performance in terms of detection rate and significance estimation as compared to that observed in the O3 online analysis. The improvements are attributed to several incremental advances in the likelihood ratio ranking statistic computation and the method of background estimation.
更多查看译文
AI 理解论文
溯源树
样例
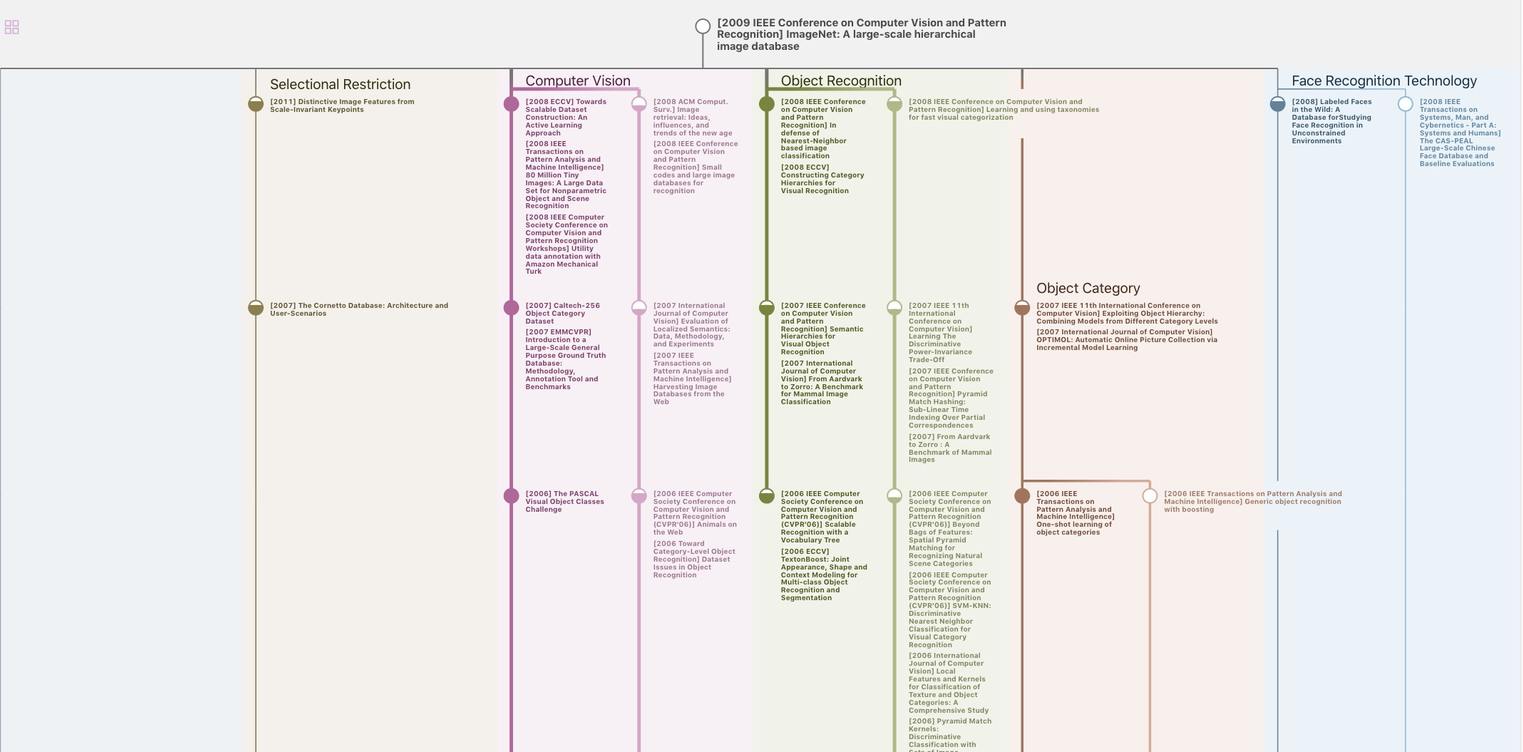
生成溯源树,研究论文发展脉络
Chat Paper
正在生成论文摘要