Representation Learning for Person or Entity-Centric Knowledge Graphs: An Application in Healthcare
CoRR(2023)
摘要
Knowledge graphs (KGs) are a popular way to organise information based on ontologies or schemas. Despite advances in KGs, representing knowledge remains a non-trivial task across industries and it is especially challenging in the biomedical and healthcare domains due to complex interdependent relations between entities, heterogeneity, lack of standardization, and sparseness of data. KGs are used to discover diagnoses or prioritize genes relevant to disease, but they often rely on schemas that are not centred around a node or entity of interest, such as a person. Entity-centric KGs are relatively unexplored but hold promise in representing important facets connected to a central node and unlocking downstream tasks beyond graph traversal and reasoning, such as training graph neural networks (GNNs) for a wide range of predictive tasks. This paper presents an end-to-end representation learning framework to extract entity-centric KGs from structured and unstructured data. We introduce a star-shaped ontology to represent the multiple facets of a person and use it to guide KG creation. Compact representations of the graphs are created leveraging GNNs and experiments are conducted using different levels of heterogeneity or explicitness. A readmission prediction task is used to evaluate the results of the proposed framework, showing a stable system, robust to missing data, that outperforms a range of baseline machine learning classifiers. We highlight that this approach has several potential applications across domains and is open-sourced.
更多查看译文
关键词
representation,knowledge,graphs
AI 理解论文
溯源树
样例
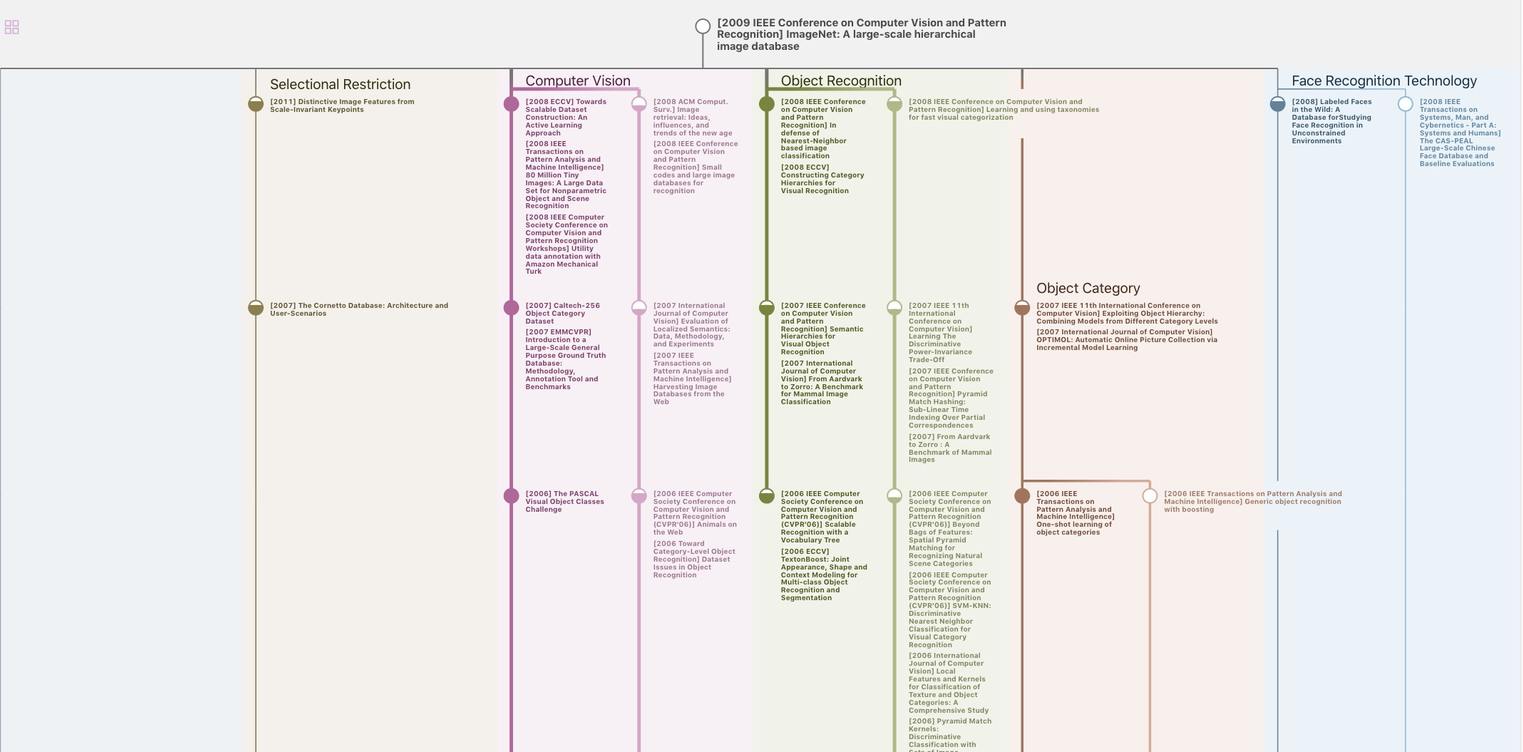
生成溯源树,研究论文发展脉络
Chat Paper
正在生成论文摘要