Self-Supervised Federated Learning for Fast MR Imaging
IEEE TRANSACTIONS ON INSTRUMENTATION AND MEASUREMENT(2024)
摘要
Federated learning (FL)-based magnetic resonance (MR) image reconstruction can facilitate learning valuable priors from multisite institutions without violating patient's privacy for accelerating MR imaging. However, existing methods rely on fully sampled data for collaborative training of the model. The client that only possesses undersampled data can neither participate in FL nor benefit from other clients. Furthermore, heterogeneous data distributions hinder FL from training an effective deep learning reconstruction model and thus cause performance degradation, and exchanging models frequently causes communication inefficiency. To address these issues, we propose a self-supervised FL method for accelerating MR imaging (SSFedMRI). SSFedMRI explores the physics-based contrastive reconstruction networks in each client to realize cross-site collaborative training in the absence of fully sampled data. Furthermore, a personalized update scheme in the local client is designed to simultaneously capture the global shared representations among different centers and maintain the specific data distribution of each client, and reduce the communication cost by downloading the global model selectively. Extensive experiments demonstrate that SSFedMRI possesses strong capability in reconstructing accurate MR images directly from multiinstitutional undersampled data with low communication cost in FL.
更多查看译文
关键词
Image reconstruction,Data models,Training,Magnetic resonance imaging,Federated learning,Deep learning,Costs,Deep learning,fast magnetic resonance (MR) imaging,federated learning (FL),MR imaging (MRI) reconstruction,self-supervised learning
AI 理解论文
溯源树
样例
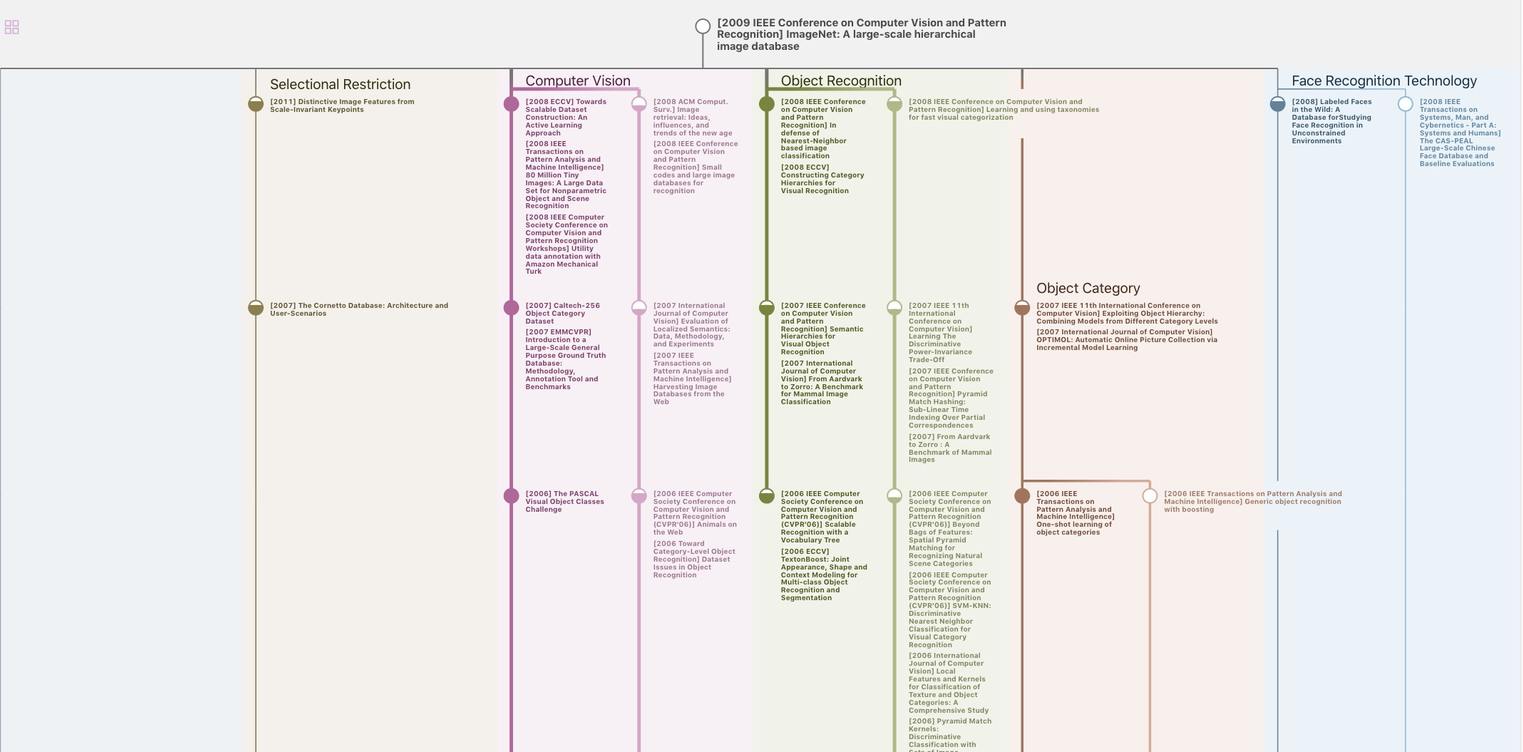
生成溯源树,研究论文发展脉络
Chat Paper
正在生成论文摘要