Robust unsupervised feature selection via dual space latent representation learning and adaptive structure learning
INTERNATIONAL JOURNAL OF MACHINE LEARNING AND CYBERNETICS(2023)
摘要
Great significance has been attached to unsupervised feature selection in consideration of the difficulty in obtaining labels. Existent unsupervised feature selection methods have the following three main shortcomings: (1) The link information between features and that between samples are not taken into account simultaneously. (2) A fixed graph is constructed to preserve the local manifold structure. (3) The impact of the different sparsification terms is ignored. To tackle these problems, robust unsupervised feature selection via dual space latent representation learning and adaptive structure learning, DSLRAS in short, is proposed. The algorithm captures the correlation between features and the correlation between samples on the basis of latent representation learning in both feature space and data space. Adaptive graph learning is utilized to maintain the local geometric structure of data more accurately. The l_2,p -norm regularization term is added so as to guarantee the row-sparsity and achieve better results. An efficient algorithm is designed to optimize the minimization problem iteratively. The convergence is proved in theory and in experiments. Extensive experiments on nine benchmark datasets are conducted which verify the effectiveness of DSLRAS in comparison with seven state-of-the-art algorithms.
更多查看译文
关键词
Unsupervised feature selection,Latent representation learning,Adaptive graph learning,Local manifold structure,Dual space
AI 理解论文
溯源树
样例
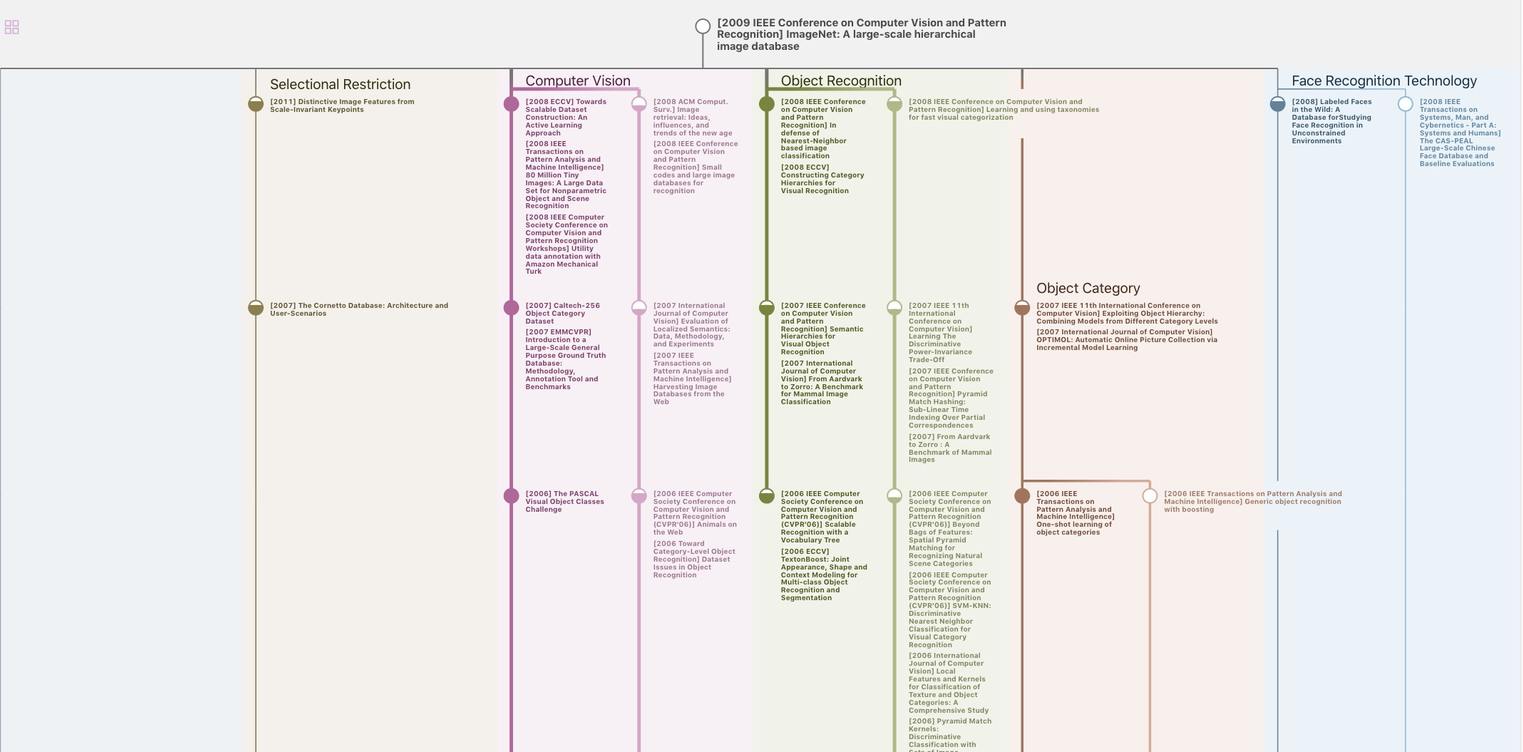
生成溯源树,研究论文发展脉络
Chat Paper
正在生成论文摘要