A multi-label learning prediction model for heart failure in patients with atrial fibrillation based on expert knowledge of disease duration
APPLIED INTELLIGENCE(2023)
摘要
Patients with atrial fibrillation (AF) are prone to complications such as heart failure with preserved ejection fraction (HFpEF), which accelerates the progress of the disease and can lead to death. It is of great practical importance to predict the onset timing and probability of HFpEF, which can guide the personalized treatment of patients with AF and improve their survival rate. However, there are no models for predicting HFpEF in patients with AF at present. A multi-label learning prediction model based on expert knowledge of disease duration is proposed in this paper to achieve accurate prediction of HFpEF in patients with AF. First, the expert knowledge of the relationship between the duration of disease progression and outcome is adopted to divided the duration of AF disease into different periods. Next, multi-label decision tree models were used to build different multi-label prediction models for each stage. With the multi-label design, the model can learn the intrinsic correlation between different labels and achieve dual-task prediction of HFpEF attack time and probability. Finally, the results from different periods were fused and output. The proposed method considers the time dependence of medical data and the development pattern of the disease, which realizes 0.0352 hloss , 0.8571 micro-F 1 score, and 0.90 average micro-AUC. The experimental results show that the proposed model achieved better prediction of onset time and outcomes in HFpEF patients, which will help the prognosis and personalize the treatment of patients.
更多查看译文
关键词
Atrial fibrillation,Heart failure prediction,Multi-label learning,Expert knowledge,Decision tree
AI 理解论文
溯源树
样例
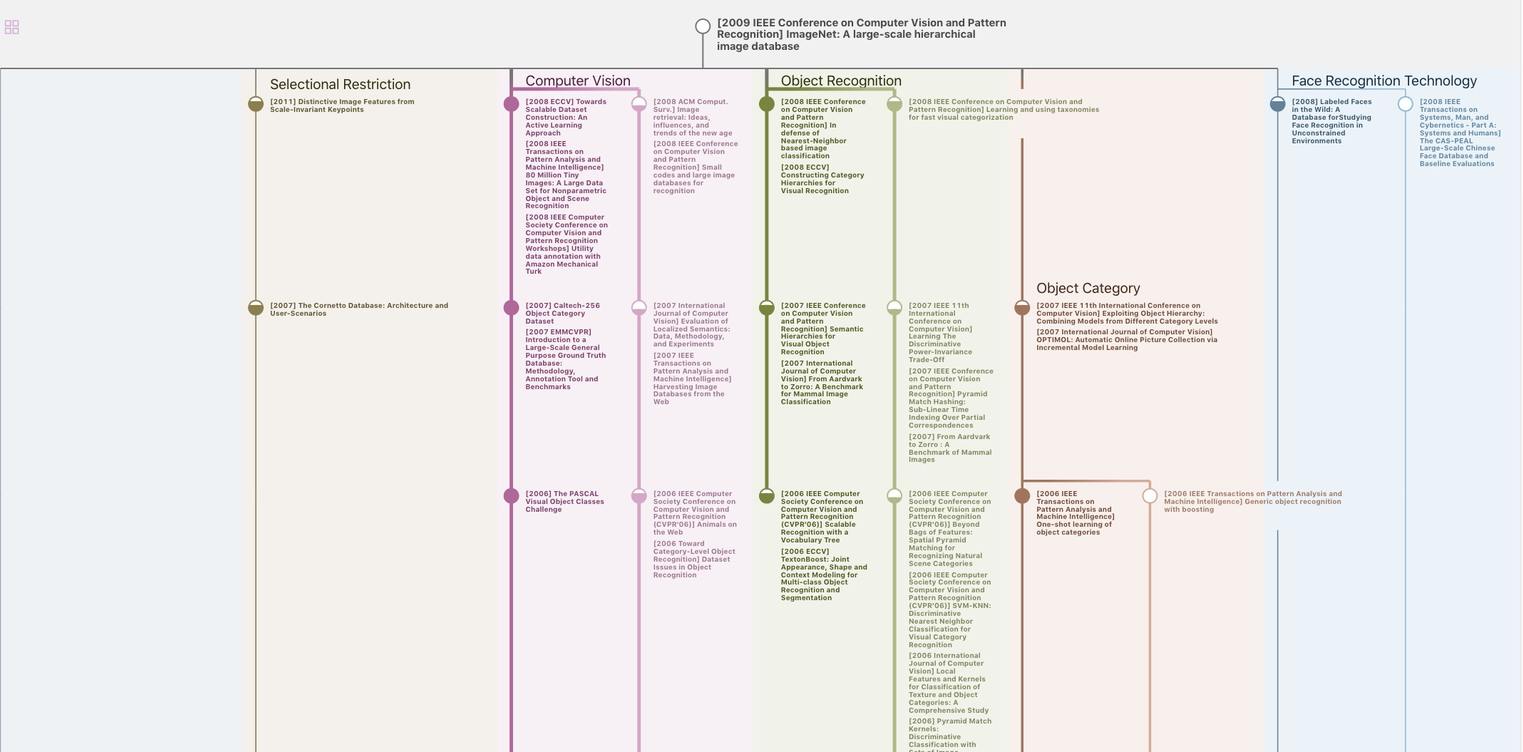
生成溯源树,研究论文发展脉络
Chat Paper
正在生成论文摘要