Efficient Data Offloading Using Markovian Decision on State Reward Action in Edge Computing
JOURNAL OF GRID COMPUTING(2023)
摘要
Efficient planning of Task offloading in edge computing for the Internet of Things (IoT) can increase latency issues and power use. In this research, we propose the task offloading issue as a joint decision-making problem for cost reductions, integrating computation delay, and handling power usage, with the goal of reducing the cost of resources required for task offloading. In this paper, a Markovian Decision Process with Deep Q-Learning (MD-DQN) is used for multi-level task offloading. The Deep Q-learning algorithm greatly enhances the accuracy and handles the task offloading decision process in mobile and edge devices. It anticipates the load on the edge server in real-time. The Markovian Decision Process reduces data unloading while assisting in decision-making. As a result, the task's reaction time will be further reduced, and the system's offloading efficiency will be improved. Employing metrics for computation time and rate power efficacy, offloading ratio, and scheduling faults, the proposed method's performance is compared. The outcomes of the experiments indicate that this proposed MD-DQN method enhances both energy efficiency and computation speed.
更多查看译文
关键词
Markovian decision process,Task offloading,Resource allocation,Decision making,Internet of Things,Computation delay,Offloading
AI 理解论文
溯源树
样例
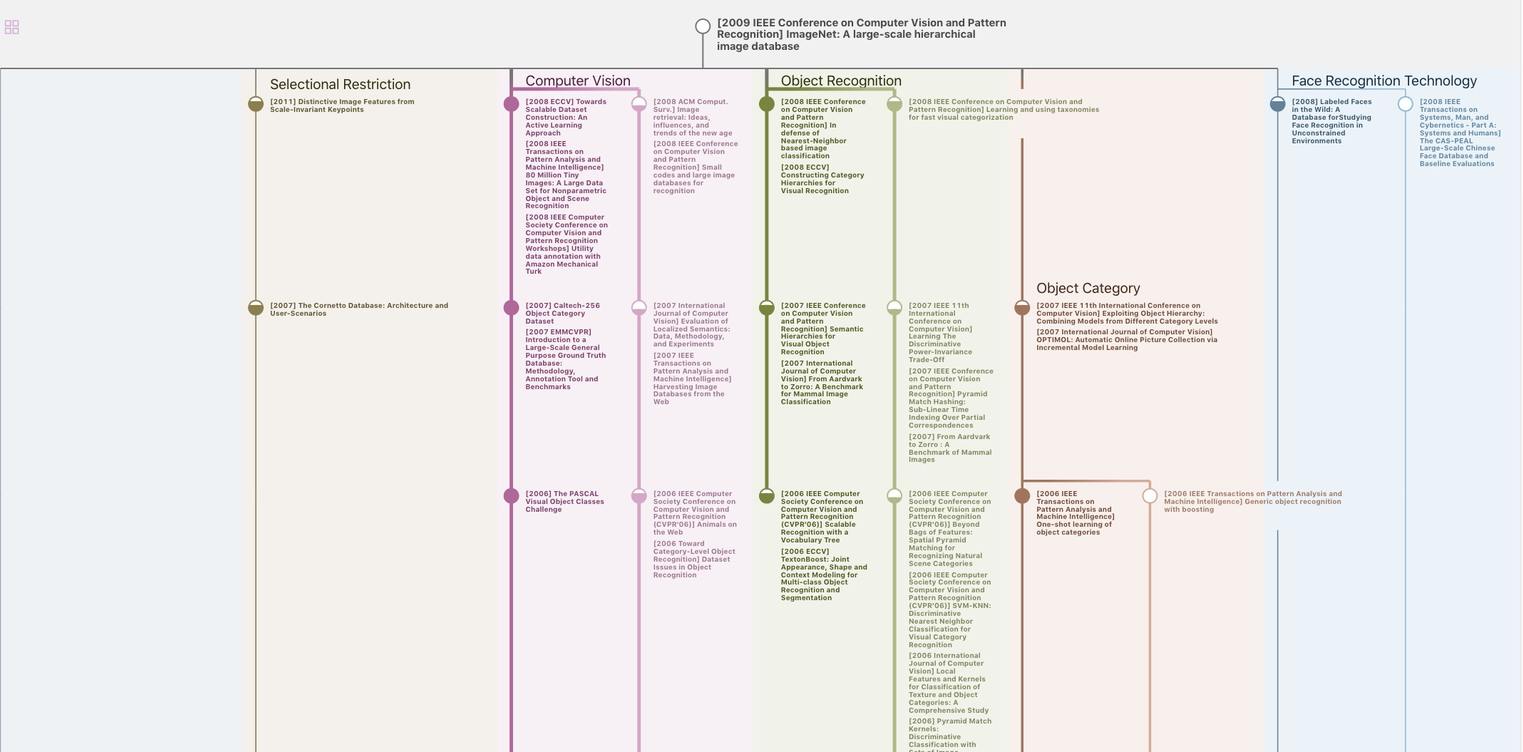
生成溯源树,研究论文发展脉络
Chat Paper
正在生成论文摘要