Robust face alignment via adaptive attention-based graph convolutional network
NEURAL COMPUTING & APPLICATIONS(2023)
摘要
We address the problem of robust face alignment in the presence of occlusions, which remains a lingering problem in facial analysis despite intensive long-term studies. This paper proposes an adaptive attention-based graph convolutional network for face alignment. Different from most existing methods that ignore the structural information, we combine local features and global structural relationships to construct the landmark-connection graph and optimize the graph to improve the robustness of the model under occlusion conditions. Specifically, we introduce a novel graph convolutional network architecture consisting of three parts: GCN-global, GCN-local, and the adaptive channel attention module. GCN-global estimates the global transformation of landmarks through 3D face fitting to obtain initial coordinates. Considering the interaction between vertexes and edges in the graph, GCN-local jointly trains local edges and vertexes to improve the accuracy. The channel attention module can adaptively select essential features to enhance the performance. In addition, to reduce the influence of occlusion parts on the other landmarks and improve the working efficiency, we apply the preprocessing module to select which keypoints need to be connected. Our method achieves 5.17% mean error with 1.89% failure rate on COFW dataset and 4.16% mean error on 300W-Full dataset. Extensive experiments demonstrate that our method outperforms most state-of-the-art models on three public datasets, including WFLW, COFW, and 300W.
更多查看译文
关键词
Face alignment,Graph convolution,Adaptive attention,Deep learning
AI 理解论文
溯源树
样例
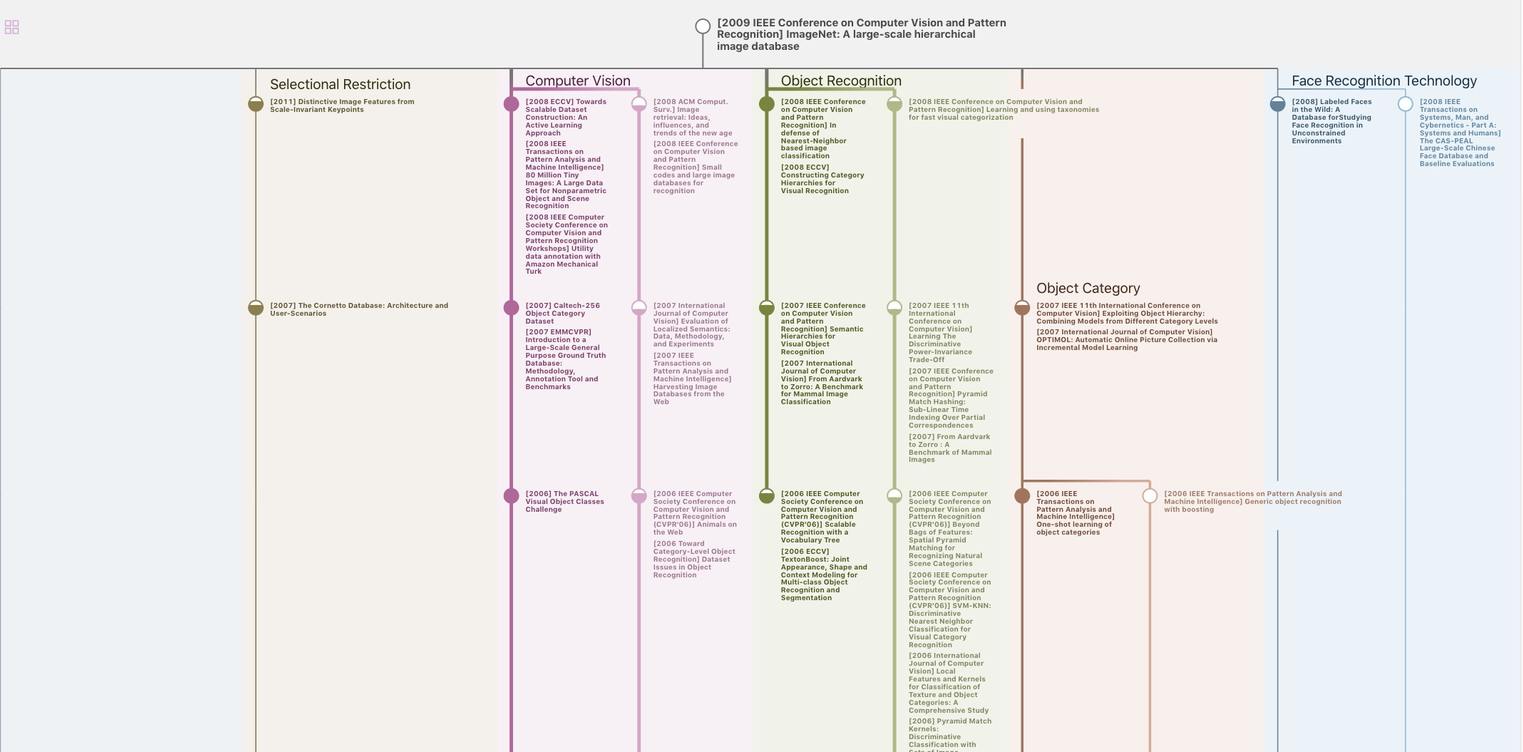
生成溯源树,研究论文发展脉络
Chat Paper
正在生成论文摘要