Ubiquitous intelligent federated learning privacy-preserving scheme under edge computing
FUTURE GENERATION COMPUTER SYSTEMS-THE INTERNATIONAL JOURNAL OF ESCIENCE(2023)
摘要
With the rapid development of artificial intelligence (AI), combining machine learning (ML) and edge computing powered by big data has become a growing trend. However, under edge computing, ML faces problems such as limited energy consumption, insufficient computing power, and data security threats. Therefore, we propose a ubiquitous intelligent federated learning privacy protection scheme(UIFLPP), which provides privacy protection for data under edge computing. First, we train part of the models in embedded devices and add matrix masks to ensure secure transmission between embedded devices and edge servers. In addition, residual model training is performed on the edge server with a differential privacy mechanism. After aggregation in the cloud, noise is added and the model is fed back to the edge server. The experimental results show that the scheme can achieve 86% accuracy on CIFAR10 on the premise of ensuring privacy and reducing the training time by 5% compared with the baseline scheme, which can well meet the needs of ML in edge scenarios. (c) 2023 Elsevier B.V. All rights reserved.
更多查看译文
关键词
Federated learning,Edge computing,Privacy protection,Ubiquitous intelligence,Machine learning
AI 理解论文
溯源树
样例
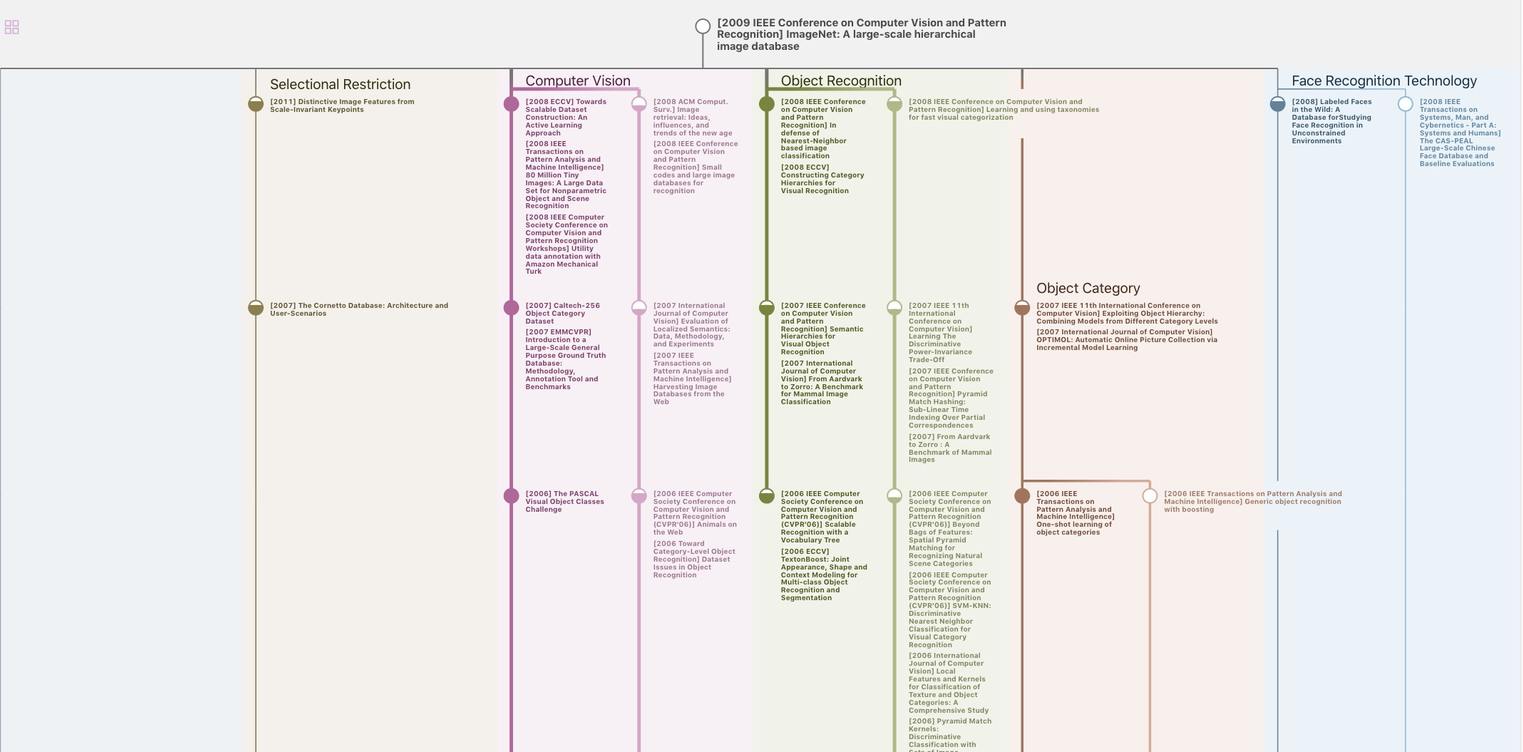
生成溯源树,研究论文发展脉络
Chat Paper
正在生成论文摘要