Novel Edge Caching Approach Based on Multi-Agent Deep Reinforcement Learning for Internet of Vehicles
IEEE TRANSACTIONS ON INTELLIGENT TRANSPORTATION SYSTEMS(2023)
摘要
Along with the development of Internet of Vehicles (IoV) and wireless technology, the usage of applications that require low latency, such as autonomous driving and intelligent navigation, is increasing rapidly, and the demand for content is increasing greatly. This paper proposes an edge caching approach for the IoV based on multi-agent deep reinforcement learning (ECSMADRL) so as to resolve the problem of excessive response delay due to the large increase of data traffic in the IoV. The approach jointly considers content distribution and caching in dynamic environments. In other words, each moving vehicle in the IoV can be seen as an agent, and it can make decisions about content caching and content access adaptively according to the changing environment to minimize the delay in the process of content distribution. It is proved by experiments that compared with other methods, the proposed edge caching (EC) approach has better performance in reducing content distribution delay, improving content hit rate and success rate.
更多查看译文
关键词
Delays,Internet of Vehicles,Wireless communication,Low latency communication,Servers,Reinforcement learning,Optimization,Content distribution,deep reinforcement learning,edge caching,multi-agent
AI 理解论文
溯源树
样例
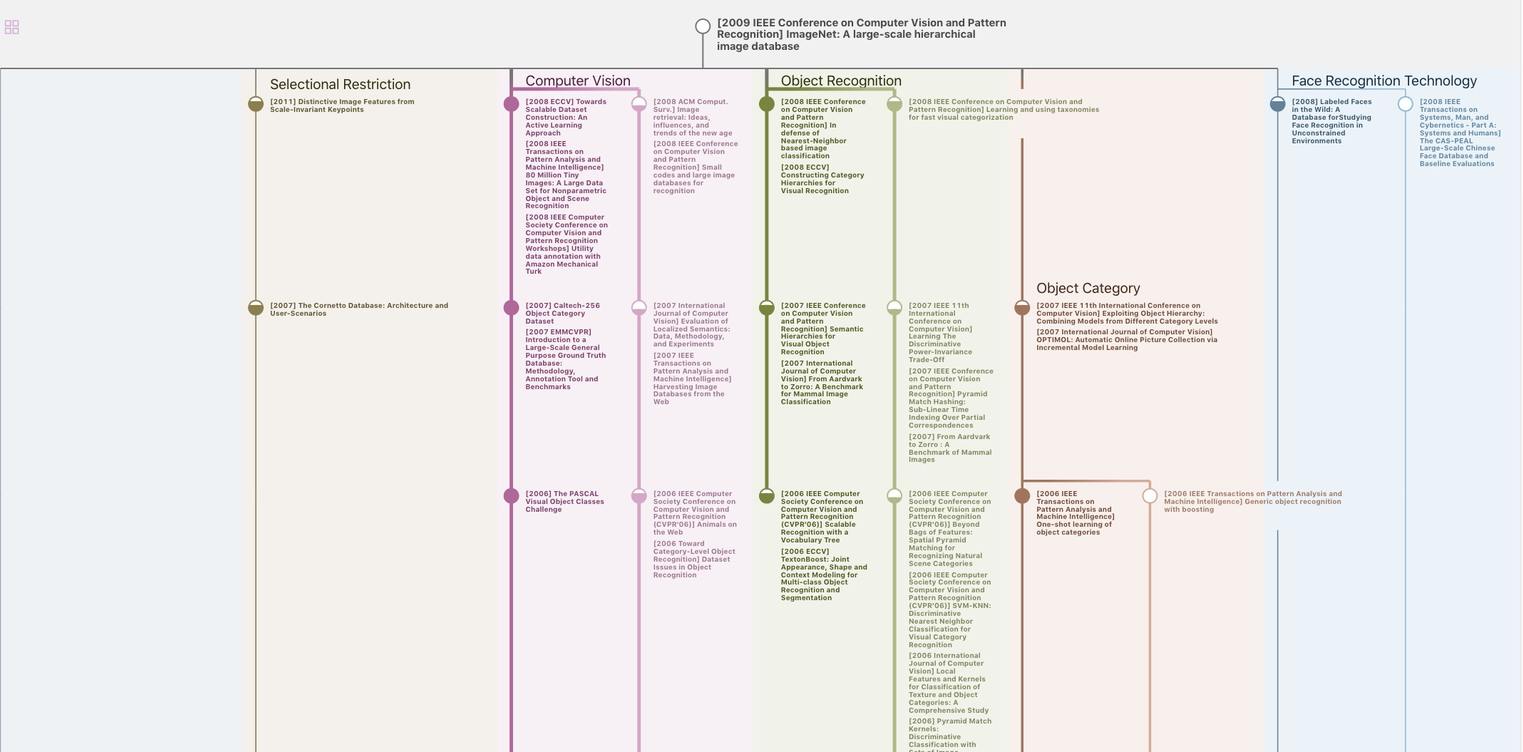
生成溯源树,研究论文发展脉络
Chat Paper
正在生成论文摘要