LSTM-based failure prediction for railway rolling stock equipment
EXPERT SYSTEMS WITH APPLICATIONS(2023)
摘要
In the railway domain, rolling stock maintenance affects service operation time and efficiency. Minimizing train unavailability is essential for reducing capital loss and operational costs. To this aim, prediction of failures of rolling stock equipment is crucial to proactively trigger proper maintenance activities. Indeed, predictive maintenance is a golden example of the digital transformation within Industry 4.0, which affects several engineering processes in the railway domain. Nowadays, it may leverage artificial intelligence and machine learning algorithms to forecast failures and schedule the optimal time for maintenance actions. Generally, rail systems deteriorate gradually over time or fail directly, leading to data that vary extremely slowly. Indeed, ML approaches for predictive maintenance should consider this type of data to accurately predict and forecast failures.This paper proposes a methodology based on Long Short-Term Memory deep learning algorithms for predictive maintenance of railway rolling stock equipment. The methodology allows us to properly learn long-term dependencies for gradually changing data, and both predicting and forecasting failures of rail equipment. In the framework of an academic-industrial partnership, the methodology is experimented on a train traction converter cooling system, demonstrating its applicability and benefits. The results show that it outperforms state-of-the-art methods, reaching a failure prediction and forecasting accuracy over 99%, with a false alarm rate of similar to 0.4% and a mean absolute error in the order of 10-4, respectively.
更多查看译文
关键词
Predictive maintenance,Machine learning,Industry 4,0,Railway rolling stock equipment
AI 理解论文
溯源树
样例
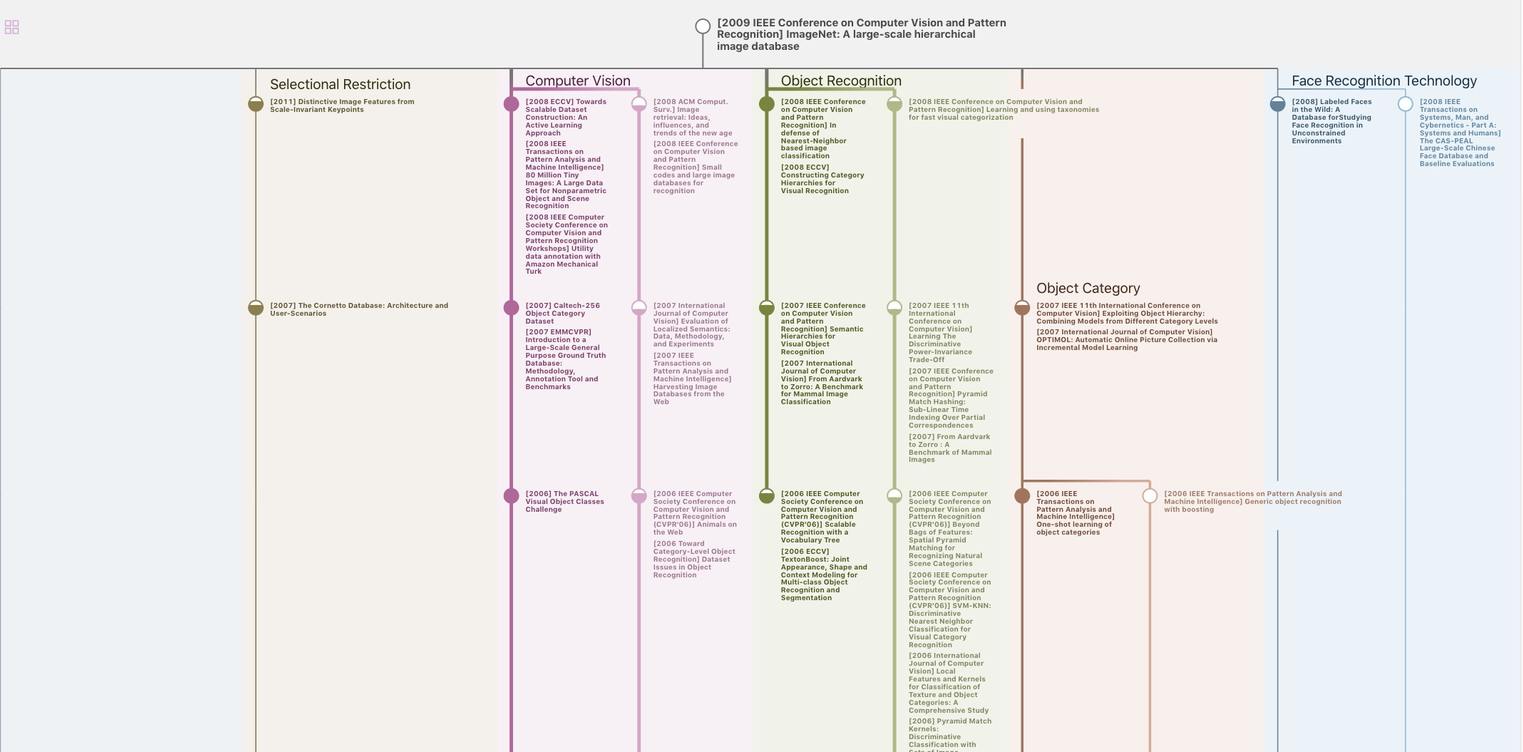
生成溯源树,研究论文发展脉络
Chat Paper
正在生成论文摘要