A learnable self-supervised task for unsupervised domain adaptation on point cloud classification and segmentation
FRONTIERS OF COMPUTER SCIENCE(2023)
摘要
Conclusion To address the UDA problem for point clouds, we propose a novel learnable self-supervised task that helps the adapted neural network extract transferable features. Specifically, we propose a learnable point cloud transformation and use it in a point cloud destruction-reconstruction self-supervised auxiliary task. We train the main task network and the auxiliary task network, which share an encoder, so that the encoder extracts features that are highly transferable to the target domain. We further propose a multi-region transformation strategy to make the network focus on local features, which are more transferable. New state-of-the-art performance is achieved on the point cloud classification and segmentation UDA benchmarks.
更多查看译文
关键词
point cloud classification,unsupervised domain adaptation,self-supervised
AI 理解论文
溯源树
样例
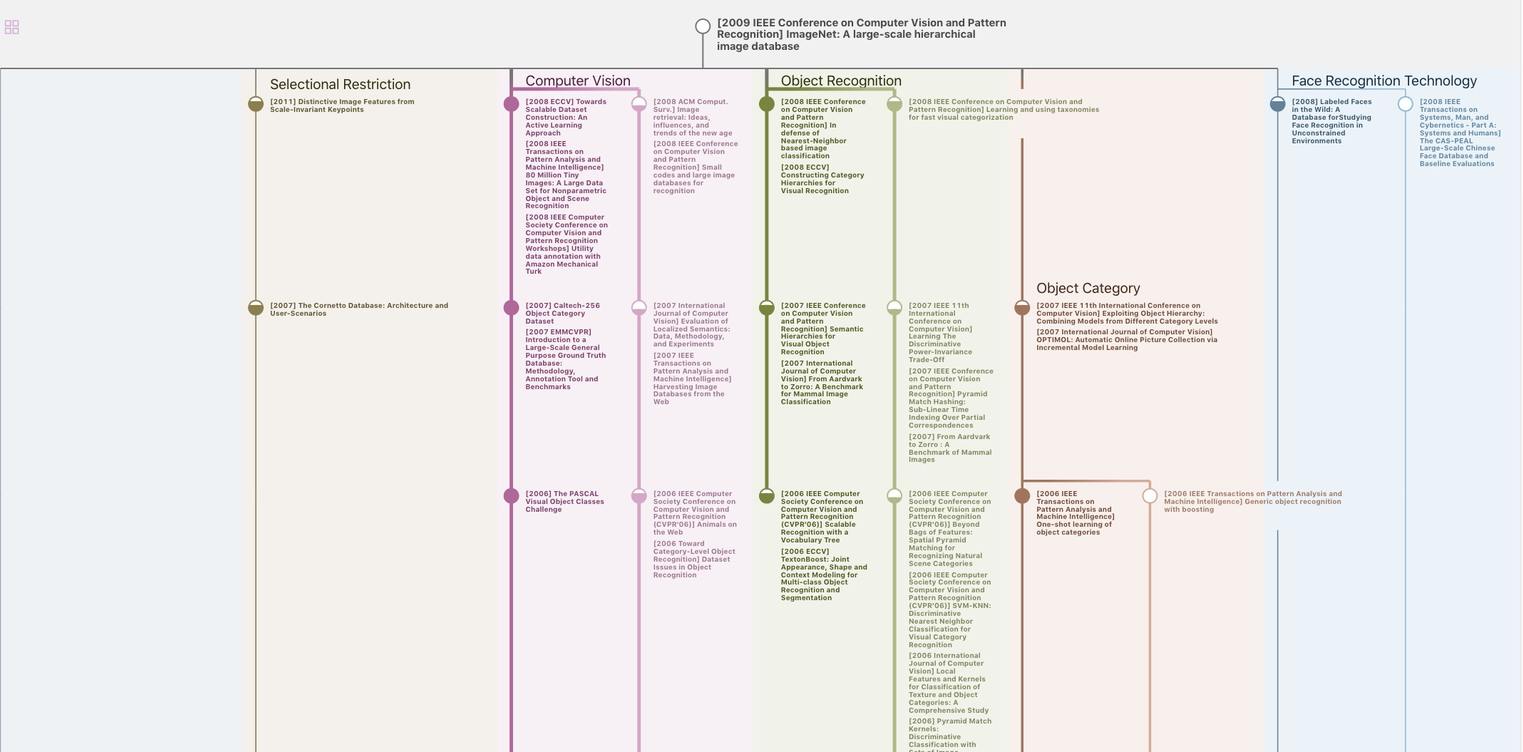
生成溯源树,研究论文发展脉络
Chat Paper
正在生成论文摘要